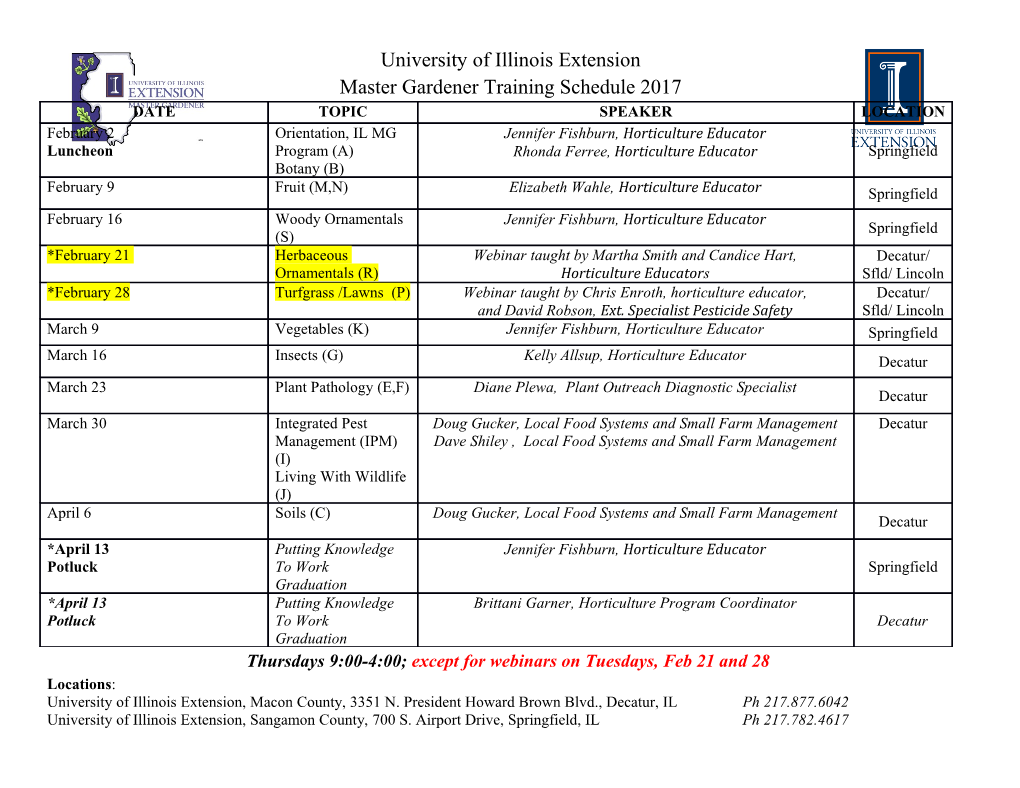
International Journal of Statistics and Systems ISSN 0973-2675 Volume 12, Number 1 (2017), pp. 119-137 © Research India Publications http://www.ripublication.com Weighted Inverse Rayleigh Distribution Kawsar Fatima and S.P Ahmad Department of Statistics, University of Kashmir, Srinagar, India. Corresponding author Abstract In this paper, we have introduced weighted inverse Rayleigh (WIR) distribution and investigated its different statistical properties. Expressions for the Mode and entropy have also been derived. In addition, it also contains some special cases that are well known. Moreover, we apply the maximum likelihood method to estimate the parameter , and applications to two real data sets show the superiority of this new distribution by comparing the fitness with its special cases. Keywords: Inverse Rayleigh distribution, weighted distribution, Reliability Analysis, Entropy, Maximum likelihood estimation, Real life data sets. 1. INTRODUCTION The IR distribution was proposed by Voda (1972). He studies some properties of the MLE of the scale parameter of inverse Rayleigh distribution which is also being used in lifetime experiments. If X has IR distribution, its probability density function (pdf) takes the following form: 2 2 g() x ex ; x 0, 0 )1.1( x3 The corresponding cumulative distribution function is 2 G( x ) ex ; x 0, 0 )2.1( Where x 0 the scale parameter 0 The kth moment of the IR distribution is given as the following: 120 Kawsar Fatima and S.P Ahmad 2 k k 2 x Eg () x x e dx. 0 x3 1 1 2 Making the substitution y , dx dy , so that x 2/1 ,we obtain x 2 x3 y y E() xk y(1k / 2) 1 e dy. g 0 k k 2/ Eg ( x ) (1 k / 2) )3.1( Then, the expected value of X can be written as: 2/1 Eg ( x ) (1/ 2) )4.1( 2. WEIGHTED INVERSE RAYLEIGH DISTRIBUTION The use and application of weighted distributions in research related to reliability, biomedicine, ecology and many other areas are of great practical importance in mathematics, probability and statistics. These distributions arise naturally as a result of observations generated from a stochastic process and recorded with some weight function. Firstly the model of weighted distributions was introduced by Fisher (1934). Cox (1962) originally provided the concept of length-biased sampling and after that Rao (1965) recognized a unifying method that can be used for several sampling situations and can be displayed by means of the weighted distributions. Cox (1968) expected mean of the original distribution built on length biased data. Recently, many researches are applied to length-biased for lifetime distribution, The Length-Biased Weighted Weibull Distribution; Tanusree Deb Roy et al(2011), The Length-biased inverse Weibull distribution; Jing Kersey and Broderick O. Oluyede (2012),The Length biased Beta distribution of first kind; Mir et al (2013),The Length- biased Exponentiated Inverted Weibull Distribution (2014),The Length-biased weighted Nakagami Distribution; Sofi Mudasir and S.P Ahmad (2015), The Length- biased Weighted Lomax Distribution; Afaq et al(2016). In this study, we propose a new distribution which is a Weighted Inverse Rayleigh (WIR) distribution. We first provide a general definition of the Weighted Inverse Rayleigh (WIR) distribution which will subsequently reveal its pdf. Definition1. If has a lifetime distribution with pdf g() x and expected value, k Eg () x , the pdf of Weighted distribution of X can be defined as: xk g() x () , 0, 0 )1.2( f x k k x Eg () x Weighted Inverse Rayleigh Distribution 121 Theorem 2.1: - Let be a random variable of an IR distribution with pdf . xk g() x Then () is a pdf of the WIR distribution with scale parameter and f x k Eg () x weight parameter k . The notation for with the WIR distribution is denoted as X~ WIR ( , k ). The pdf of is given by: (1 / 2) 2 k 2 f() x xk3 e / x ; x 0, 0,k 0 (2.2) (1 k / 2) Proof: -By definition 1, substitute (1.1) and (1.3) into (2.1), then the pdf for the WIR distribution can be obtained by: X k x 2 2 f() x e x k / 2(1 k / 2) x3 (1k / 2) 2 2 f() x xk3 e x (1 k / 2) Figure 1 illustrates some of the possible shapes of the probability density function of Weighted inverse Rayleigh distribution for selected values of and k g() x Figure 1: The probability density function of the WIR distribution for selected values of and k 122 Kawsar Fatima and S.P Ahmad We observe from Figure1 that the density function of WIR is positively skewed and that the curve decreases as the value of increases. So, the shape of the proposed WIR distribution could be decreasing. Also, we observed that the shape of the proposed WIR distribution could be unimodal. Theorem 2.2: - Let be a random variable of the WIR distribution with parameter & k . The distribution function of the WIR distribution is written as: k 1 , 2 x 2 F() x )3.2( k 1 2 X where s, x ts1 et dt is an upper incomplete gamma function. x Proof: - Generally, the distribution function of lifetime distribution is defined as: x F()() x f x dx )4.2( 0 Substituting (2.3) into (2.4), we obtain: (1k / 2) x 2 2 F() x xk3 e x dx 0 (1 k / 2) 2 By setting y , dx dy , y , the above integration becomes: x 2 x3 x2 k 1 1 1 2 y F() x y e dy (1 k / 2) 2 x k 1 , 2 x 2 F() x k 1 2 The corresponding plots of the WIR distribution function at various values of and k are shown in Figure 2. Weighted Inverse Rayleigh Distribution 123 X Figure 2: The distribution function of the WIR distribution for selected values of and k . The distribution curves show the increasing rate. Theorem 2.3: - Let X be a random variable of the WIR distribution with Parameter & k . The survival function of the WIR distribution can be written as: k 1 , 2 x 2 S() x )5.2( k 1 2 x where s, x ts1 e t dt is a lower incomplete gamma function. 0 Proof: - By definition, the survival function of the random variable is given by: S( x ) 1 F ( x ) . Using (2.3), the survival function of the WIR distribution can be expressed by: k 1 , 2 x 2 S( x ) 1 k `1 2 124 Kawsar Fatima and S.P Ahmad k k 1 1 , 2 2 2 x k 1 2 k 1 , 2 2 x . k 1 2 Figure 3 illustrates some of the possible shapes of the survival function of Weighted inverse Rayleigh distributionX for selected values of and k Figure 3: The survival function of the WIR distribution for different values of and k . The survival curves show the decreasing rate. Theorem 2.4: - Let be a random variable of the WIR distribution with Parameter & k . The hazard rate of the WIR distribution takes the form: 2 21k / 2x k 3 e x h() x )6.2( k 1 , 2 x 2 Proof: -Let be a continuous random variable with pdf and survival function, f() x and S() x , respectively, then the hazard rate is defined by: Weighted Inverse Rayleigh Distribution 125 f() x h() x . )7.2( S() x Substituting (2.2) and (2.5) into (2.7), we obtain: 2 21k / 2x k 3 ex / (1 k / 2) h() x k 1 , / (1 k / 2) 2 x 2 2 21k / 2x k 3 e x k 1 , X 2 2 x Figure 4 illustrates some of the possible shapes of the hazard function of Weighted inverse Rayleigh distribution for selected values of and k k Figure 4: The hazard rate of the WIR distribution for different values of and . We can infer from Figure 4 that the shape of the hazard rate is positively skewed, if the value of increases the hazard rate decreases. We can also say that the hazard rate shows an inverted bathtub shape or unimodal. Theorem 2.5: - Let be a random variable of the WIR distribution with Parameter & k . The reverse hazard rate of the WIR distribution takes the form: 126 Kawsar Fatima and S.P Ahmad 2 21k / 2x k 3 e x ()x (2.8) k 1 , 2 x 2 Proof: -Let be a continuous random variable with pdf and cdf, f() x and F() x , respectively, then the reverse hazard rate is defined by: f() x ()x . (2.9) F() x Substituting (2.2) and (2.3) into (2.9), we obtain: X2 21k / 2x k 3 ex / (1 k / 2) ()x k 1 , / (1 k / 2) 2 x 2 2 21k / 2x k 3 e x k 1 , 2 2 x k Figure 5 illustrates some of the possible shapes of the Reverse hazard rate function of weighted inverse Rayleigh distribution for selected values of and . k Figure 5: The reverse hazard function of the WIR distribution for different values of and . 3. SOME SPECIAL CASES OF WEIGHTED INVERSE RAYLEIGH DISTRIBUTIONS Weighted Inverse Rayleigh Distribution 127 This section presents some special cases that deduced from equation (2.2) are Case 1: When k 0 , then weighted inverse Rayleigh distribution (2.2) reduces to inverse Rayleigh distribution (IRD) with probability density function as: 2 2 f() x e x )1.3( x3 Case 2: When k 1, then weighted inverse Rayleigh distribution (2.2) reduces to length biased inverse Rayleigh distribution (IRD) with probability density function as: 2/1 2 2 f() x x2 e x )2.3( )2/1( Case 3: If a random variable is such that YX /1 and k k in Equation (2.2) reduces to give the weighted Rayleigh distribution (WRD) with probability density function as: 2 2(k / 2 1)x k 1 e x f() x )3.3( (k / 2 1) Case 4: If a random variable is such that and k 1 in Equation (2.2) reduces to give the length biased Rayleigh distribution (LBRD) with probability density function as: 2 4(3/ 2)x 2 ex f() x )4.3( )2/1( Case 5: If a random variable is such that YX /1 and k 0 in Equation (2.2) reduces to give the Rayleigh distribution (RD) with probability density function as: 2 f( x ) 2xe x )5.3( 4.
Details
-
File Typepdf
-
Upload Time-
-
Content LanguagesEnglish
-
Upload UserAnonymous/Not logged-in
-
File Pages20 Page
-
File Size-