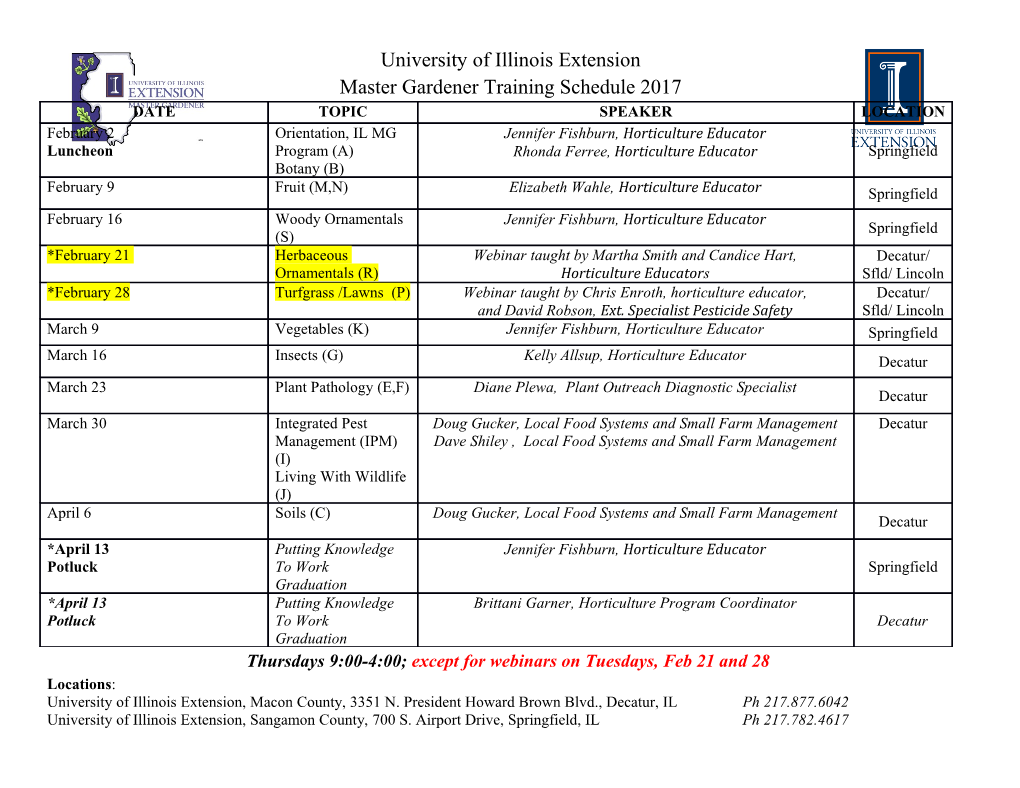
Towards Mechanistic Pharmacodynamic Modeling for Cancer Precision Medicine Marc R. Birtwistle Associate Professor Department of Chemical and Biomolecular Engineering Clemson University Western South Carolina AIChE Meeting Greenville, SC 12 Feb 2019 Snapshot of Attrition During Drug Development 808 oral small- molecule compounds at their highest recorded phase of development Waring et al., Nat Rev Drug Discovery, 2015 Marc R. Birtwistle 2 Simulation is Typically an Integral Component of Design • Example of airplane building – Build many airplanes, see which ones don’t crash? – No! – Sufficient understanding of fluid dynamics and physics allows simulation to screen design ideas • Human biology is far more complex and less understood—even in how to simulate it – Need more basic research o Physiological and pathophysiological mechanisms o Modeling and simulation methods to capture said mechanisms Marc R. Birtwistle 3 Systems Pharmacology Drug Model Outcome 1. Modeling Drug ??? Outcome 2. Simulation Drug Model ??? 3. Control ??? Model Outcome 4 Targeted Therapy was found not to outperform Physician’s Choice Genomics ≠ Drugs 5 BRAF CRAF BRAF Dabrafenib MEK MEK Trametinib ERK ERK 6 What More Does Cancer Precision Medicine Need to Consider? One DriverOne TargetOne Drug 1. Systems • Driver may not be a good direct drug target • Drivers interact; 4-7 drivers per tumor (maybe more) 2. Polypharmacology • Multiple driversmultiple targetsmultiple drugs • Most targeted drugs are promiscuous 3. Dynamics • Tumors adapt and evolve on multiple time scales 4. Heterogeneity • Clonal cells show transient resistance • Cancers comprise multiple subclones with different drivers and microenvironments Marc R. Birtwistle 7 Marc R. Birtwistle 8 Quantitative Systems Pharmacology: Mechanistic Kinetic Modeling of Biochemical Networks ka g g* E-I ki kbm kdm m I E E-S P kbp k S dp p dp = k m - k p I E-S-I dt bp dp Stochastic Gene Expression dm = k g*-k m dt bm dm dg* = k g - k g* dt a i dg = k g*-k g dt i a Marc R. Birtwistle 9 Outline • Mechanistic Models of Cancer Cell Signaling – Formulation, Building, and Training – Stochastic Cell Cycle Entry – Stochastic Cell Death • Towards Training with Big Pharmacological Data • Reconstructing Cell Signaling Networks from Perturbation Time Course Data Marc R. Birtwistle 10 Marc R. Birtwistle 11 Defining Model Scope Pathways: • RTK-RAS-RAF-MAPK • PI3K-AKT-mTOR • Cell Cycle • p53-DNA Damage Adapted from Ciriello G et al., Nature Genetics 2013 Model is Composed of Pathway-Specific Models from the Literature Submodel Origin • Birtwistle MR et al., MSB 2007 Receptor • Bouhaddou M & Birtwistle MR, Tyrosine Mol Biosys 2014 Kinase • Many others • Birtwistle MR et al., MSB 2007 Proliferation & • Nakakuki T et al., Cell 2010 Growth • Kriegsheim A et al., Nat Cell Bio 2009 DNA Damage • Batchelor E et al., MSB, 2011 • Gerard C & Goldbeter A, PNAS Cell Cycle 2009 • Albeck JG et al., Plos Biology Apoptosis 2008 Expression The standard model System of ordinary differential equations (ODEs) ~800 species and ~2800 reactions A Prettier Picture EXTRACELLULAR SPACE CYTOPLASM RECEPTOR DEATH TYROSINE KINASE RECEPTORS EGFR, Erbb2, Erbb3, Erbb4, cMet, PDGFR, FGFR, IGFR, INSR, EGF, HRG, HGF, PDGF, FGF, IGF, Insulin MITOCHONDRIA GROWTH TSC1, TSC2, mTOR, APOPTOSIS Rictor, Raptor, S6K, PROLIFERATION EIF4EBP1, EIF4E, Grb-2, SOS, Cbl, Sprouty, TRAIL ligand, TRAIL Ribosomes Ras, C-Raf, B-Raf, MEK, receptors (DR4-DR5), ERK, cJun, cFos, NF1, Caspases 8/3/6/9, tBID, DUSP6, DUSP1, RSK, IRS, BIM, BAX, Cytochrome C, PLCg, GRP, PI3KC1, PI3KR1, APAF, XIAP, PARP, Flip, PI3K2, PTEN, PDK1, AKT, Bar, Smac, Bad, Bcl2, FOXO, GSK3-β, bCatenin, PUMA, NOXA f cMyc TRANSLATION DEATH EXPRESSION NUCLEUS Genes and mRNAs TRANSCRIPTION CELL CYCLE f Cyclins D/E/A/B, CDKs DNA DAMAGE 4/6/2/1, Chk1, p21, ATM, ATR, p53, WIP1, EPIGENETICS p27, E2F, Rb, Wee1, MDM2, MDM4, ARF, CDH1, CDC25A, BRCA2, MSH6, MGMT CDC25B, CDC25C, Skp2, Cdc20 DIVISION Model considers 141 genes Single Cells Have Stochastic Response to Drugs Adapted from Gascoigne and Taylor, Cancer Cell 2008 Cell-to-cell variability: Simulating Stochastic Gene Expression STOCHASTIC g g* mRNA production m f Transcriptional p f Activators and Repressors SIGNALING (TARs) DETERMINISTIC Increasing Confidence in Models INTEGRATED UNIT TESTING UNIT TESTING ANALYSIS Evaluate input-output Evaluate input- Use model to reason behavior of individual output behavior of about biological sub-models model as a whole observations Cell context: Start with non-transformed MCF10A • Predictable phenotypic behaviors • Few alterations • Extensive literature data and widely studied Marc R. Birtwistle 17 Unit Testing—Expression: Tailoring Model to Quantitative Expression Context Define “expression context”: 1. GENOME: Gene copy number 2. TRANSCRIPTOME: mRNA levels Post-Initialization 3. PROTEOME: Protein levels Concentration (nM) Concentration PROTEOMICS (Mass Spectrometry) PROTEIN Serum- LEVELS Starved MCF10A Cells TRANSCRIPTOMICS (RNA-seq) mRNA SAMPLE INITIALIZATION LEVELS GENOMICS* (Sequencing) GENE COPY RUN SIMULATIONS NUMBER* * Bessette et al., Plos One 2015 Unit Testing – Receptor Tyrosine Kinase (RTK) Ligand-Receptor EGFR ErbB2 ErbB3 ErbB4 Cooperativity Behavior EGF HRG HGF cMET PDGFR FGFR IGFR INSR PDGF LITERATURE DICTATES Negative cooperativity (n<1) FGF No cooperativity (n=1) Positive cooperativity (n>1) [Receptor Complex] (nM) Complex] [Receptor IGF INS [Ligand Dose] Unit Testing – Proliferation & Growth EGF and Insulin ERK Micro-Western Blot ERK AKT mTOR AKT mTOR Experiments by Rick Koch and Anne Marie Barrette Unit Testing Submodel Required Properties for Each Submodel Receptor • Ligand-receptor cooperativity matches experimental observations. Tyrosine Kinase • Receptor trafficking kinetics reflects experimental observations. Proliferation & • Receptor pathway preferences match experimental observations. • Basal activity fluxes through ERK and AKT pathways exist, tailored to the serum-starved state. Growth • Dynamic dose responses of ERK, AKT, and mTOR signaling matches experimental western blot data. Cell Cycle • Cell cycle entry is driven by induction of cyclin D mRNA. • Order and timing of cyclin/cdk complexes matches established observations. • Cell cycle duration matches that in MCF10A cells. • Upregulation of p21 arrests the cell cycle. Apoptosis • Robustness against small death signals. • Model exhibits all-or-nothing death response when apoptosis signaling surpasses threshold. • Dose and dynamics of TRAIL-induced extrinsic apoptosis matches experimental observations. • Intrinsic apoptosis signaling responds to interrupted survival signaling and DNA damage induced upregulation of pro-apoptotic proteins. DNA Damage • Convert original delayed differential equations into ordinary differential equations. • p53 dynamics corresponding to single- and double-stranded DNA breaks matches experimental observations. • Rate of DNA damage repair is dependent on levels of repair enzymes. • p53 activation dynamics exhibit “digital” and not “analog” behavior, whereby the number of p53 pulses, but not pulse height or width, scales to magnitude of DNA damage. • Etoposide-induced DNA damage is dependent on the cell cycle stage (S-phase). Expression • Model is tailored to genomic, transcriptomic, and proteomic context of MCF10A cells. • Stochastic gene expression is simulated with a computationally efficient algorithm. • Cell-to-cell variability in mRNA and protein levels matches experimental observations. • EIF4E levels possess extrinsic control over the translation rate. • Ribosomes double during the course of one cell cycle. Integrated Unit Testing – Cell Cycle How are synergistic EGF and Insulin signals integrated by the cell? mitogens kinase inhibitors PROLIFERATION APOPTOSIS DEATH CELL DNA CYCLE DAMAGE DIVISION chemotherapy Spatiotemporal Dynamics of Signaling? PC-12 Cells Sustained ppERK Transient Adapted from Marshall, Cell, 1995 HEK293 cells Short times (under 15 min) Marc R. Birtwistle 24 Integrated Unit Testing – Cell Cycle How are synergistic EGF and Insulin signals integrated by mitogens kinase inhibitors the cell? Cell Fate Biochemistry PROLIFERATION APOPTOSIS SIMULATIONS EXPERIMENT EGF (μ-western blot) DEATH @6hrs CELL DNA INS CYCLE DAMAGE Cyclin D (AU) EGF+INS DIVISION chemotherapy SIMULATION [CYC [CYC A/Cdk2] @6hrs % Proliferating Cells % Proliferating EGF+INS EXPERIMENT +MEKi (BrdU incorporation/ Flow cytometry) Cyclin Cyclin D (nM) @ 24hrs EGF+INS +AKTi Time (hours) @ 24hrs % Proliferating Analysis: Prolonged AKT Activation Explains EGF and Insulin S-Phase Synergy EXPERIMENT (μ-western blot) E 10nM E 1nM EGF Alone E 0.1nM E 0.01nM I 1721nM I 17nM Insulin Alone I 1.7nM ppERK ppERK (AU) I 0.17nM ppAKT (AU) High/High (E/I) High/Low Low/High Combos Low/Low ERK AKT Dynamics Dynamics 26 Inhibitor Time Course Experiments post GF post GF post GF (~24h post post GF starvation) 0 h ~ 18 h ~42 h add ~66 h Seed cells starve treat cells Inhibitors (24 h post GF harvest cells with GF treatment) cells ERK Pathway Inhibitor Akt Inhibitor Fold Proliferation Analysis – Cell Cycle: Phospho-ERK levels dictate stochastic cell cycle entry SIMULATION: In response to EGF + Insulin Initial Levels Cycling (C) *** Not cycling (NC) ppERK ppERK (nM) ppERK C NC Time (hours) * ppAKT ppAKT (nM) ppAKT C NC Time (hours) Analysis – Cell Cycle: Can Stochastic Cell Cycle Response Be Predicted? In MCF10A cells: 1. Lasso regression Predictors: Total initial protein levels SOS Responses: Cycling or non-cycling BRaf and CRaf were top hits ERK 2. Train SVM classifier
Details
-
File Typepdf
-
Upload Time-
-
Content LanguagesEnglish
-
Upload UserAnonymous/Not logged-in
-
File Pages56 Page
-
File Size-