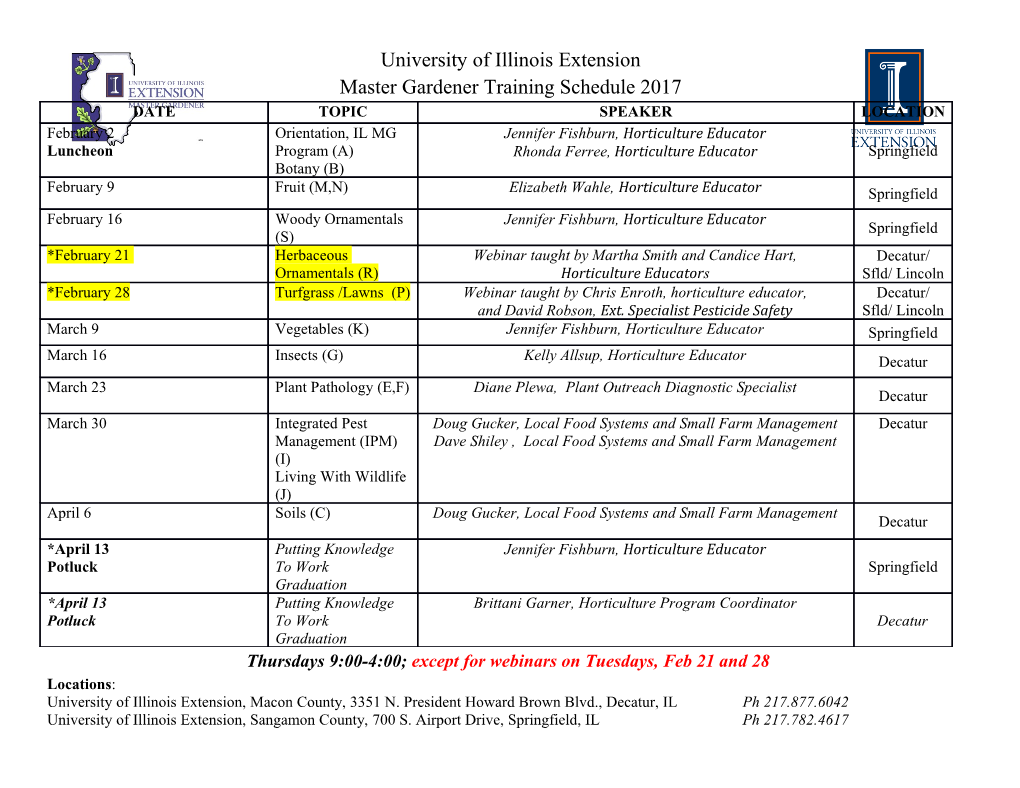
Motricidade © Edições Desafio Singular 2019, vol. 15, n. 1, pp. 49-61 http://dx.doi.org/10.6063/motricidade.16370 Data-driven analysis of point-by-point performance for male tennis player in Grand Slams Yixiong Cui1, Haoyang Liu1*, Hongyou Liu2,3, Miguel-Ángel Gómez4 ORIGINAL ARTICLE ABSTRACT Tennis is an individual sport that requires a specialized training and match preparation for every player. Former studies in tennis have tried many approaches to analyze player’s performance using descriptive statistics (such as: match time, rally duration, or game number) and match-related statistics (such as: first and second serve percentage, aces, double faults, or net points won). Although helpful in providing general information of match characteristics and evaluating player performance, there is scarce consideration over how elite male players behave on point-by-point basis according to different contextual variables. This study aimed to assess predictors of point outcome (win/lose) related to year, tournament types, round, set, quality of opposition, game status, serve and rally by using match data of 2011-2016 four Grand Slam. A total of 29675 points were recorded and analyzed through classification tree analysis (exhaustive CHAID). The results showed that the performance of tennis player was conditioned by the familiarity with court surfaces as well as other contextual variables, such as game type, quality of opposition, match status, serve and return and rally length (p< 0.05). These results provide insight for coaches and players when planning the game strategy, allowing more appropriate tactics under different game status. Keywords: racket sports; performance analysis; training; contextual variables; data analysis. INTRODUCTION 2016; Sanchez-Pay, Palao, Torres-Luque, & Sanz- Match performance of tennis player is one of Rivas, 2015), considering the match outcome or the topics that attract major research interest in game location (McGarry et al., 2013; Reid, sport performance analysis (McGarry, Morgan, & Whiteside, 2016). However, little was O'Donoghue, & Sampaio, 2013). Competing in known about how the performance of a tennis professional tennis requires player to win the player is influenced by other contextual factors, game by making proper strategies and tactics such as the opponent’s quality and match status, according to different tournaments, rounds, sets, which have been investigated in major team quality of opponents and consistently tailoring sports like basketball and football (Gómez, them based on the match periods and match Lorenzo, Ibañez, & Sampaio, 2013; Lago-Peñas, status. With the application of performance 2012). These contextual factors take into profiling techniques, the technical, tactical, consideration the game location, game status, physical and psychological features of tennis quality of opposition, period of play and type of players could be evaluated and compared competition and their effects on player’s (Butterworth, O'Donoghue, & Cropley, 2013). performance and game outcome (Gómez, Lago, & The available research has intended to describe Pollard, 2013). the performance of tennis players of different sex Gillet, Leroy, Thouvarecq, and Stein (2009) (Hizan, Whipp, & Reid, 2011) and different were ones of the first to assess the influence of levels (Galé-Ansodi, Castellano, & Usabiaga, game location on tennis player’s serve and return Manuscript received at January 6th 2019; Accepted at February 2nd 2019 1 AI Sports Engineering Lab, School of Sports Engineering, Beijing Sport University, Beijing, China 2 School of Physical Education & Sports Science, South China Normal University, Guangzhou, China 3 National Demonstration Centre for Experimental Sports Science Education, South China Normal University, Guangzhou, China 4 Facultad de Ciencias de la Actividad Física y del Deporte, Universidad Politécnica de Madrid, Madrid, Spain * Corresponding author: Informational Road No. 48, Haidian District, 100084 Beijing, China Email: [email protected] 50 | Y Cui, H Liu, H Liu, MA Gómez performance, after which Galé-Ansodi et al. analyze in detail how tennis player performed (2016) attempted to describe the physical when competing against various level of performance of youth tennis player on hard and opponents under distinct match status on clay courts. More recently Reid et al. (2016) different court surfaces and understand their described the match performance of male and interactions, instead of just analyzing and female tennis players in Australian Open, which comparing the result of the performance. was on hard court. However, the influence of Therefore, by applying the classification tree other court surface like grass, strength of the model, the present study was aimed to examine opponents and game status were not considered. the point-by-point performance of tennis players Hence, it is of interest to know how tennis player in four Grand Slams, considering perform under these different contextual comprehensively various contextual variables circumstances. such as court surfaces, opponent’s quality and As the application of new technology in sport match status. According to the existing fields is generating a great amount of data on knowledge and variables included in the study, it match and training performance of athletes is hypothesized that players would exhibit better (Hughes & Franks, 2015; McGarry et al., 2013), performance when competing against lower- it is necessary to evaluate their performance in a ranked opponents or have positive match status more in-depth manner for advanced such as winning in his service or return game. understanding of match behaviors. This Meanwhile, we expect that players would ultimately can be used to help in-game coach perform worse when playing in the quarterfinals, decision-making with emphasis on those semifinals and finals as well as playing longer performance indicators which are of greatest rally points. relevance to achieving a winning outcome and also to optimizing individualized training for METHOD players (Reid, McMurtrie, & Crespo, 2010; Reid Participants et al., 2016). Point-level data of 145 Grand Slam main-draw Applying data mining and statistical men’s singles matches played by a top ranked modelling techniques, sport data could be male tennis player and his opponents within year interpreted to provide meaningful information 2011 to 2016 were collected from separate official and insights (Ofoghi, Zeleznikow, MacMahon, & tournament websites: Australian Open Raab, 2013). And being one of these techniques, (www.ausopen.com), Roland Garros classification tree analysis has been used lately by (www.rolandgarros.com), Wimbledon researchers of sport science, particularly in team (http://www.wimbledon.com) and US Open sports (Gómez, Battaglia, et al., 2015) and proved (www.usopen.org). The player was stably ranked to be useful in modeling non-linear phenomena. top 3 in Association of Tennis Professionals (ATP, It establishes a hierarchical solution to classify www.atpworldtour.com) through 2011-2016, complex problems, where a set of rules is derived being number 1 in ranking from 2014-2016. from the interaction between attributes in a data Therefore, the analysis of this player and his set, with high predictive accuracy (Gómez, opponents is not only expected to guarantee a Battaglia, et al., 2015). Previous studies has better understanding on how elite tennis players applied the technique to model the ball screen are conditioned by matches contexts but also and effectiveness in basketball (Gómez, Lorenzo, et provides point-level performance profiles of those al., 2013), the match outcome in Australian players. football (Robertson, Back, & Bartlett, 2016), the The data included the notational statistics such as ball possession effectiveness in futsal (Gómez, first and second serve, rally numbers and game Moral, & Lago-Peñas, 2015) and the effect of scores; and ball speed statistics collected by scoring first on match outcome in football (Lago- Doppler radar system (IBM: Armonk, NY, USA) Peñas, Gómez-Ruano, Megías-Navarro, & Pollard, that were installed in the courts. Matches that 2016). This inspired us to use this model to were not completed due to the retirement of the Point-by-point performance analysis in tennis | 51 opponents or a walkover were excluded from the Committee non-human subjects research and all study. In total, there were 29675 points (8035 in procedures are conducted following the European Australian Open, 7638 in Roland Garros, 7915 in General Data Protection Law in order to maintain Wimbledon and 6087 in US Open). The study the anonymity of sampled players. was approved by the local University Ethics Table 1 Variables analyzed and correspondent sub-categories Variable Categories M (SD) Situational Variables Grand Slam Australian Open, Roland Garros, Wimbledon and US Open N/A Year 2011, 2012, 2013, 2014, 2015, 2016 N/A First round, second round, third round, fourth round, quarterfinals, Game type N/A semifinals and final Quality of opposition Top10, top 11-20, top 21-50, top 51-100 and over 100 N/A Serving player The analyzed player and opponents N/A Serve number First and second serve N/A Winning, losing, drawing, breaking opponent (when the analyzed player Game status N/A returns), being broken (when the analyzed player serves) Match Performance Variables Fast speed (n = 7251) 188.8 (8.6) First serve (km/h) Slow speed (n = 1175) 154.7 (12.5) Fast speed (n = 1879) 182.3 (13.9) Second Serve (km/h) Slow speed (n = 3016) 145.4 (10.0)
Details
-
File Typepdf
-
Upload Time-
-
Content LanguagesEnglish
-
Upload UserAnonymous/Not logged-in
-
File Pages13 Page
-
File Size-