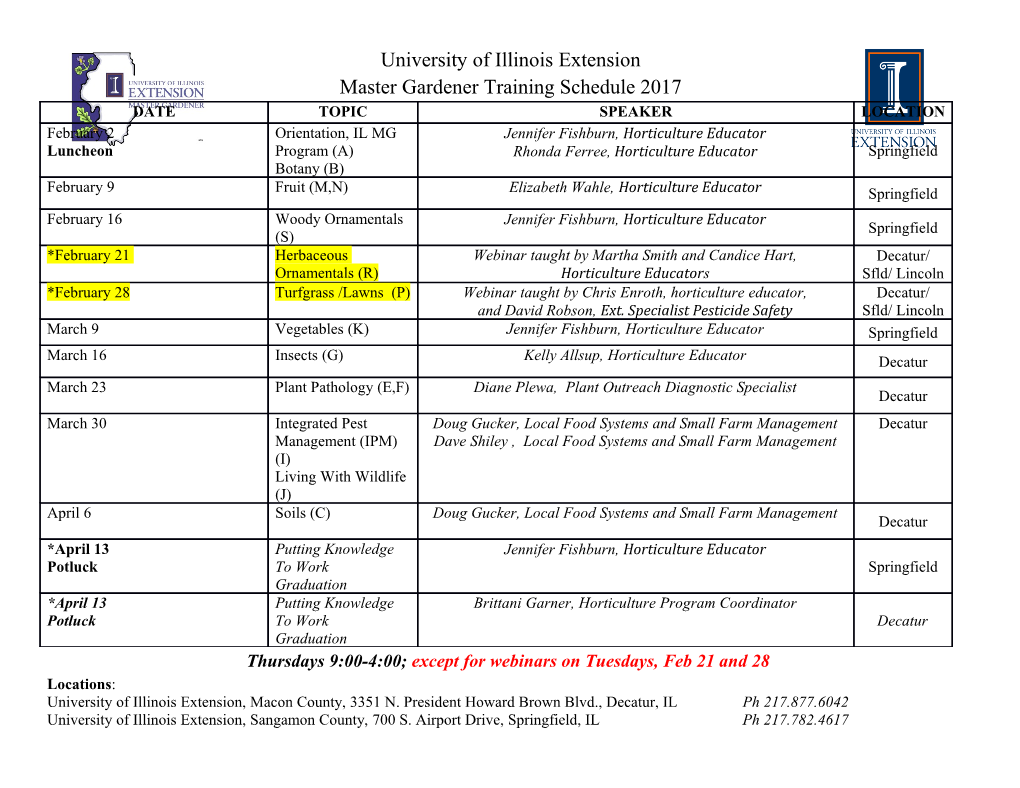
Generative Model-Based Text-to-Speech Synthesis Heiga Zen (Google London) February rd, @MIT Outline Generative TTS Generative acoustic models for parametric TTS Hidden Markov models (HMMs) Neural networks Beyond parametric TTS Learned features WaveNet End-to-end Conclusion & future topics Outline Generative TTS Generative acoustic models for parametric TTS Hidden Markov models (HMMs) Neural networks Beyond parametric TTS Learned features WaveNet End-to-end Conclusion & future topics Text-to-speech as sequence-to-sequence mapping Automatic speech recognition (ASR) “Hello my name is Heiga Zen” ! Machine translation (MT) “Hello my name is Heiga Zen” “Ich heiße Heiga Zen” ! Text-to-speech synthesis (TTS) “Hello my name is Heiga Zen” ! Heiga Zen Generative Model-Based Text-to-Speech Synthesis February rd, of Speech production process text (concept) fundamental freq voiced/unvoiced char freq transfer frequency speech transfer characteristics magnitude start--end Sound source fundamental voiced: pulse frequency unvoiced: noise modulation of carrier wave by speech information air flow Heiga Zen Generative Model-Based Text-to-Speech Synthesis February rd, of Typical ow of TTS system TEXT Sentence segmentation Word segmentation Text normalization Text analysis Part-of-speech tagging Pronunciation Speech synthesis Prosody prediction discrete discrete Waveform generation ) NLP Frontend discrete continuous SYNTHESIZED ) SEECH Speech Backend Heiga Zen Generative Model-Based Text-to-Speech Synthesis February rd, of Rule-based, formant synthesis [] Sample-based, concatenative synthesis [] Model-based, generative synthesis Speech synthesis approaches Heiga Zen Generative Model-Based Text-to-Speech Synthesis February rd, of Sample-based, concatenative synthesis [] Model-based, generative synthesis Speech synthesis approaches Rule-based, formant synthesis [] Heiga Zen Generative Model-Based Text-to-Speech Synthesis February rd, of Model-based, generative synthesis Speech synthesis approaches Rule-based, formant synthesis [] Sample-based, concatenative synthesis [] Heiga Zen Generative Model-Based Text-to-Speech Synthesis February rd, of Speech synthesis approaches Rule-based, formant synthesis [] Sample-based, concatenative synthesis [] Model-based, generative synthesis p(speech= | text=”Hello, my name is Heiga Zen.”) Heiga Zen Generative Model-Based Text-to-Speech Synthesis February rd, of Synthesis Estimate posterior predictive distribution • p(x w, , ) ! | X W Sample x¯ from the posterior distribution • Probabilistic formulation of TTS Random variables Speech waveforms (data) Observed X Transcriptions (data) Observed W w Given text Observed x Synthesized speech Unobserved Heiga Zen Generative Model-Based Text-to-Speech Synthesis February rd, 6of Probabilistic formulation of TTS Random variables Speech waveforms (data) Observed X Transcriptions (data) Observed W w Given text Observed x Synthesized speech Unobserved Synthesis Estimate posterior predictive distribution w • X W p(x w, , ) ! | X W x Sample x¯ from the posterior distribution • Heiga Zen Generative Model-Based Text-to-Speech Synthesis February rd, 6of Probabilistic formulation Introduce auxiliary variables (representation) +factorizedependency p(x w, , )= p(x o)p(o l, λ)p(l w) | X W y | | | l X8 X8L p( )p( , λ)p(λ)p( )/ p( ) dod dλ X|O O | L L |W X O where , o: Acoustic features w O X W , l: Linguistic features L l λ: Model O L λ o x Heiga Zen Generative Model-Based Text-to-Speech Synthesis February rd, of Approximation () Approximate sum & integral by best point estimates (like MAP) [] { } p(x w, , ) p(x oˆ) | X W ⇡ | w where X W ˆ ˆ ˆ ˆ l oˆ, l, , , λ = arg max O L { O L } o,l, , ,λ O L p(x o)p(o l, λ)p(l w) λ | | | p( )p( , λ)p(λ)p( ) o X|O O | L L |W oˆ x Heiga Zen Generative Model-Based Text-to-Speech Synthesis February rd, 8of Approximation () Joint Step-by-step maximization [] ! ˆ = arg max p( ) Extract acoustic features w O X|O X W O ˆ = arg max p( ) Extract linguistic features l L L|W O L L ˆ ˆ lˆ λˆ = arg max p( ˆ ˆ,λ)p(λ) Learn mapping O L λ O|L λ lˆ= arg max p(l w) Predict linguistic features l | λˆ oˆ = arg max p(o lˆ, λˆ) Predict acoustic features o o | x¯ f (oˆ)=p(x oˆ) Synthesize waveform oˆ ⇠ x | x Heiga Zen Generative Model-Based Text-to-Speech Synthesis February rd, of Approximation () Joint Step-by-step maximization [] ! ˆ = arg max p( ) Extract acoustic features w O X|O X W O ˆ = arg max p( ) l L L|W O L L ˆ ˆ lˆ λˆ = arg max p( ˆ ˆ,λ)p(λ) O L λ O|L λ lˆ= arg max p(l w) l | λˆ oˆ = arg max p(o lˆ, λˆ) o o | x¯ f (oˆ)=p(x oˆ) oˆ ⇠ x | x Heiga Zen Generative Model-Based Text-to-Speech Synthesis February rd, of Approximation () Joint Step-by-step maximization [] ! ˆ = arg max p( ) Extract acoustic features w O X|O X W O ˆ = arg max p( ) Extract linguistic features l L L|W O L L ˆ ˆ lˆ λˆ = arg max p( ˆ ˆ,λ)p(λ) O L λ O|L λ lˆ= arg max p(l w) l | λˆ oˆ = arg max p(o lˆ, λˆ) o o | x¯ f (oˆ)=p(x oˆ) oˆ ⇠ x | x Heiga Zen Generative Model-Based Text-to-Speech Synthesis February rd, of Approximation () Joint Step-by-step maximization [] ! ˆ = arg max p( ) Extract acoustic features w O X|O X W O ˆ = arg max p( ) Extract linguistic features l L L|W O L L ˆ ˆ lˆ λˆ = arg max p( ˆ ˆ,λ)p(λ) Learn mapping O L λ O|L λ lˆ= arg max p(l w) l | λˆ oˆ = arg max p(o lˆ, λˆ) o o | x¯ f (oˆ)=p(x oˆ) oˆ ⇠ x | x Heiga Zen Generative Model-Based Text-to-Speech Synthesis February rd, of Approximation () Joint Step-by-step maximization [] ! ˆ = arg max p( ) Extract acoustic features w O X|O X W O ˆ = arg max p( ) Extract linguistic features l L L|W O L L ˆ ˆ lˆ λˆ = arg max p( ˆ ˆ,λ)p(λ) Learn mapping O L λ O|L λ lˆ= arg max p(l w) Predict linguistic features l | λˆ oˆ = arg max p(o lˆ, λˆ) o o | x¯ f (oˆ)=p(x oˆ) oˆ ⇠ x | x Heiga Zen Generative Model-Based Text-to-Speech Synthesis February rd, of Approximation () Joint Step-by-step maximization [] ! ˆ = arg max p( ) Extract acoustic features w O X|O X W O ˆ = arg max p( ) Extract linguistic features l L L|W O L L ˆ ˆ lˆ λˆ = arg max p( ˆ ˆ,λ)p(λ) Learn mapping O L λ O|L λ lˆ= arg max p(l w) Predict linguistic features l | λˆ oˆ = arg max p(o lˆ, λˆ) Predict acoustic features o o | x¯ f (oˆ)=p(x oˆ) oˆ ⇠ x | x Heiga Zen Generative Model-Based Text-to-Speech Synthesis February rd, of Approximation () Joint Step-by-step maximization [] ! ˆ = arg max p( ) Extract acoustic features w O X|O X W O ˆ = arg max p( ) Extract linguistic features l L L|W O L L ˆ ˆ lˆ λˆ = arg max p( ˆ ˆ,λ)p(λ) Learn mapping O L λ O|L λ lˆ= arg max p(l w) Predict linguistic features l | λˆ oˆ = arg max p(o lˆ, λˆ) Predict acoustic features o o | x¯ f (oˆ)=p(x oˆ) Synthesize waveform oˆ ⇠ x | x Heiga Zen Generative Model-Based Text-to-Speech Synthesis February rd, of Approximation () Joint Step-by-step maximization [] ! ˆ = arg max p( ) Extract acoustic features w O X|O X W O ˆ = arg max p( ) Extract linguistic features l L L|W O L L ˆ ˆ lˆ λˆ = arg max p( ˆ ˆ,λ)p(λ) Learn mapping O L λ O|L λ lˆ= arg max p(l w) Predict linguistic features l | λˆ oˆ = arg max p(o lˆ, λˆ) Predict acoustic features o o | x¯ f (oˆ)=p(x oˆ) Synthesize waveform oˆ ⇠ x | x Representations: acoustic, linguistic, mapping Heiga Zen Generative Model-Based Text-to-Speech Synthesis February rd, of Based on knowledge about spoken language ! Lexicon, letter-to-sound rules • Tokenizer, tagger, parser • Phonology rules • Representation – Linguistic features Hello, world. Sentence: length, ... Hello, world. Phrase: intonation, ... hello world Word: POS, grammar, ... h-e2 l-ou1 w-er1-l-d Syllable: stress, tone, ... h e l ou w er l d Phone: voicing, manner, ... Heiga Zen Generative Model-Based Text-to-Speech Synthesis February rd, of Representation – Linguistic features Hello, world. Sentence: length, ... Hello, world. Phrase: intonation, ... hello world Word: POS, grammar, ... h-e2 l-ou1 w-er1-l-d Syllable: stress, tone, ... h e l ou w er l d Phone: voicing, manner, ... Based on knowledge about spoken language ! Lexicon, letter-to-sound rules • Tokenizer, tagger, parser • Phonology rules • Heiga Zen Generative Model-Based Text-to-Speech Synthesis February rd, of Needs to solve inverse problem ! Estimate parameters from signals • Use estimated parameters (e.g., cepstrum) as acoustic features • Representation – Acoustic features Piece-wise stationary, source-lter generative model p(x o) | Vocal source Vocal tract filter Pulse train (voiced) Cepstrum, LPC, ... overlap/shift Aperiodicity, [dB] windowing voicing, ... voicing, Fundamental 80 frequency + Speech e(n) 0 x(n) = h(n)*e(n) 0 8 [kHz] h(n) White noise (unvoiced) Heiga Zen Generative Model-Based Text-to-Speech Synthesis February rd, of Representation – Acoustic features Piece-wise stationary, source-lter generative model p(x o) | Vocal source Vocal tract filter Pulse train (voiced) Cepstrum, LPC, ... overlap/shift Aperiodicity, [dB] windowing voicing, ..
Details
-
File Typepdf
-
Upload Time-
-
Content LanguagesEnglish
-
Upload UserAnonymous/Not logged-in
-
File Pages69 Page
-
File Size-