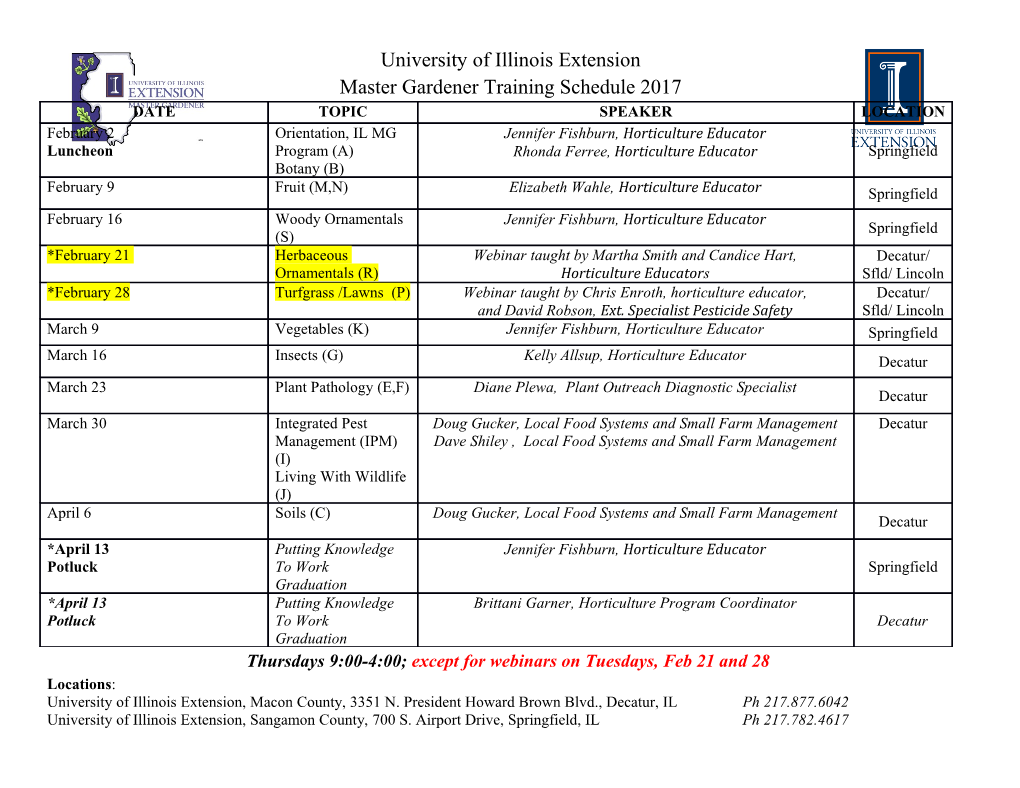
StreamBox: Modern Stream Processing on a Multicore Machine Hongyu Miao and Heejin Park, Purdue ECE; Myeongjae Jeon and Gennady Pekhimenko, Microsoft Research; Kathryn S. McKinley, Google; Felix Xiaozhu Lin, Purdue ECE https://www.usenix.org/conference/atc17/technical-sessions/presentation/miao This paper is included in the Proceedings of the 2017 USENIX Annual Technical Conference (USENIX ATC ’17). July 12–14, 2017 • Santa Clara, CA, USA ISBN 978-1-931971-38-6 Open access to the Proceedings of the 2017 USENIX Annual Technical Conference is sponsored by USENIX. StreamBox: Modern Stream Processing on a Multicore Machine Hongyu Miao1, Heejin Park1, Myeongjae Jeon2, Gennady Pekhimenko2, Kathryn S. McKinley3, and Felix Xiaozhu Lin1 1Purdue ECE 2Microsoft Research 3Google Abstract Most stream processing engines are distributed be- cause they assume processing requirements outstrip the Stream analytics on real-time events has an insatiable de- capabilities of a single machine [38, 28, 32]. How- mand for throughput and latency. Its performance on a ever, modern hardware advances make a single multi- single machine is central to meeting this demand, even in core machine an attractive streaming platform. These ad- a distributed system. This paper presents a novel stream vances include (i) high throughput I/O that significantly StreamBox processing engine called that exploits the improves ingress rate, e.g., Remote Direct Memory Ac- parallelism and memory hierarchy of modern multicore cess (RDMA) and 10Gb Ethernet; (ii) terabyte DRAMs StreamBox hardware. executes a pipeline of transforms that hold massive in-memory stream processing state; over records that may arrive out-of-order. As records ar- and (iii) a large number of cores. This paper seeks to rive, it groups the records into ordered epochs delineated maximize streaming throughput and minimize latency on by watermarks. A watermark guarantees no subsequent modern multicore hardware, thus reducing the number of record’s event timestamp will precede it. required machines to process streaming workloads. Our contribution is to produce and manage abun- Stream processing on a multicore machine raises three dant parallelism by generalizing out-of-order record pro- major challenges. First, the streaming engine must ex- cessing within each epoch to out-of-order epoch pro- tract parallelism aggressively. Given a set of trans- cessing and by dynamically prioritizing epochs to opti- forms {d ,d ,···,d } in a pipeline, the streaming en- mize latency. We introduce a data structure called cas- 1 2 n gine should exploit (i) pipeline parallelism by simultane- cading containers, which dynamically manages concur- ously processing all the transforms on different records rency and dependences among epochs in the transform in the data stream and (ii) data parallelism on all the pipeline. StreamBox creates sequential memory layout available records in a transform. Second, the engine of records in epochs and steers them to optimize NUMA must minimize thread synchronization while respecting locality. On a 56-core machine, StreamBox processes dependences. Third, the engine should exploit the mem- records up to 38 GB/sec (38M Records/sec) with 50 ms ory hierarchy by creating sequential layout and minimiz- latency. ing data copying as records flow through various trans- forms in the pipeline. 1 Introduction To address these challenges, we present StreamBox, an out-of-order stream processing engine for multicore Stream processing is a central paradigm of modern data machines. StreamBox organizes out-of-order records analytics. Stream engines process unbounded numbers into epochs determined by arrival time at pipeline of records by pushing them through a pipeline of trans- ingress and delimited by periodic event time watermarks. forms, a continuous computation on records [3]. Records It manages all epochs with a novel parallel data struc- have event timestamps, but they may arrive out-of-order, ture called cascading containers. Each container man- because records may travel over diverse network paths ages an epoch, including its records and end watermark. and computations on records may execute at different StreamBox dynamically creates and manages multiple rates. To communicate stream progression, transforms inflight containers for each transform. StreamBox links emit timestamps called watermarks. Upon receiving a upstream containers to their downstream consuming con- StreamBox watermark wts, a transform is guaranteed to have ob- tainers. provides three core mechanisms: served all prior records with event time ≤ ts. (1) StreamBox satisfies dependences and transform USENIX Association 2017 USENIX Annual Technical Conference 617 correctness by tracking producer/consumer epochs, Table 1: Terminology records, and watermarks. It optimizes throughput and la- Term Definition tency by creating abundant parallelism. It populates and Stream An unbounded sequence of records processes multiple transforms and multiple in progress Transform A computation that consumes and produces streams containers per transform. For instance, when watermark Pipeline A dataflow graph of transforms processing is a long latency event, StreamBox is not Watermark A special event timestamp for marking stream progression Epoch A set of records arriving between two watermarks stalled, because as soon as any subsequent records arrive, Bundle A set of records in an epoch (processing unit of work) it opens new containers and starts processing them. Evaluator A worker thread that processes bundles and watermarks (2) StreamBox elastically maps software parallelism Container Data structure that tracks watermarks, epochs, and bundles to hardware. It binds a set of worker threads to cores. Window A temporal processing scope of records (i) Each thread independently retrieves a set of records To achieve low latency, the stream engine must contin- (a bundle) from a container and performs the transform, uously process records and thus cannot stall waiting for producing new records that it deposits to a downstream event and arrival time to align. We adopt the out-of-order container(s). (ii) To optimize latency, it prioritizes the processing (OOP) [27] paradigm based on windows to processing of containers with timestamps required for address this challenge. the next stream output. As is standard in stream pro- cessing, outputs are scoped by temporal windows that are Watermarks and stream epochs Ingress and trans- scoped by watermarks to one or more epochs. forms emit strictly monotonic event timestamps called (3) StreamBox judiciously places records in memory watermarks wts, as exemplified in Figure 1(a). A wa- by mapping streaming access patterns to the memory ar- termark guarantees no subsequent records will have an chitecture. To promote sequential memory access, it or- event time earlier than ts. At ingress, watermarks de- ganizes pipeline state based on the output window size, limit ordered consecutive epochs of records. An epoch placing records in the same windows contiguously. To may have records with event timestamps greater than the maximize NUMA locality, it explicitly steers streams to epoch’s end watermark due to out-of-order arrival. The flow within local NUMA nodes rather than across nodes. stream processing engine may process records one at a We evaluate StreamBox on six benchmarks with a 12- time or in bundles. core and 56-core machine. StreamBox scales well up We rely on stream sources and transforms to cre- to 56 cores, and achieves high throughput (millions of ate watermarks based on their knowledge of the stream records per second) and low latency (tens of millisec- data [2, 3]. We do not inject watermarks (as does prior onds) on out-of-order records. On the 56-core system, work [7]) to force output and manage buffering. StreamBox reduces latency by a factor of 20 over Spark Pipeline egress Transforms define event-time windows Streaming [38] and matches the throughput of results of that dictate the granularity at which to output results. Be- Spark and Apache Beam [3] on medium-size clusters of cause we rely on watermarks to define streaming pro- 100 to 200 CPU cores for grep and wordcount. gression, the rate of egress is bounded by the rate of wa- The full source code of StreamBox is available at termarks, since a transform can only close a window af- http://xsel.rocks/p/streambox. ter it receives a watermark. We define the output delay in a pipeline from the time it first receives the watermark wts that signals the completion of the current window to 2 Stream model and background the moment when it delivers the window results to the user. This critical path is implicit in the watermark times- This section describes our out-of-order stream process- tamps. It includes processing any remaining records in ing model and terminology, summarized in Table 1. epochs that precede wts and processing wts itself. Streaming pipelines A stream processing engine re- Programming model We use the popular model from ceives one or more streams of records and performs a se- timely dataflow [30], Google dataflow [3], and others. To D { , ,···, } quence of transforms = d1 d2 dn on the records compose a pipeline, developers declare transforms and R ∈ R . Each record rts has a timestamp ts for tempo- define dataflows among transforms. This is exemplified ral processing. A record has an event timestamp defined by the following code that defines a pipeline for Win- by its occurrence (e.g., when a sensor samples a geoloca- dowed Grep, one benchmark used in our evaluation (§9). tion). Ingress of a record to the stream engine determines // 1. Declare transforms its arrival timestamp. Source<string> source(/*config info*/); FixedWindowInto <string> fwi(seconds(1)); Out-of-order streaming Because data sources are di- WindowedGrep <string>wingrep(/*regexp*/); verse, records travel different paths, and transforms op- Sink<string > sink(); erate at different rates, records may arrive out-of-order at // 2. Create a pipeline the stream processing engine or to individual transforms. Pipeline* p = Pipeline::create(); 618 2017 USENIX Annual Technical Conference USENIX Association $QHSRFK :DWHUPDUNV p->apply(source); //set source (QG 6WDUW 7UDQVIRUP // 3.
Details
-
File Typepdf
-
Upload Time-
-
Content LanguagesEnglish
-
Upload UserAnonymous/Not logged-in
-
File Pages15 Page
-
File Size-