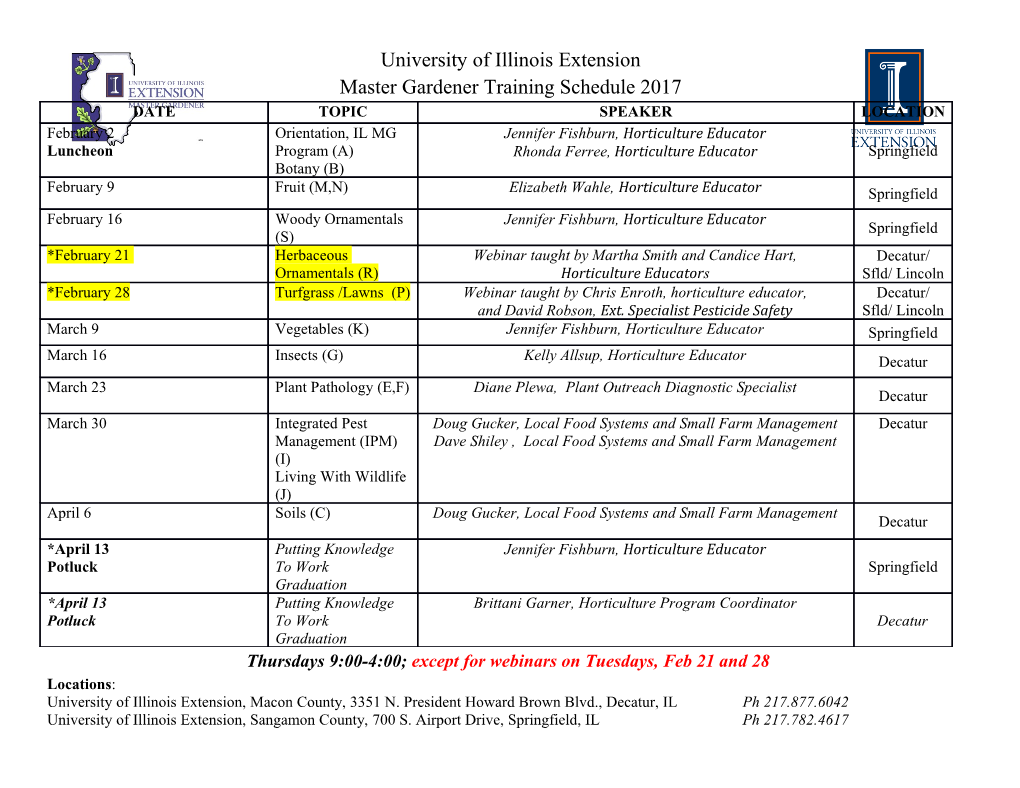
Rice Science, 2011, 18(2): − Copyright © 2010, China National Rice Research Institute. Published by Elsevier BV. All rights reserved Estimating Rice Yield by HJ-1A Satellite Images 1 1, 2 1 LI Wei-guo , LI Hua , ZHAO Li-hua (1Institute of Economy and Information, Jiangsu Academy of Agricultural Sciences, Nanjing 210014, China; 2Anhui Agricultural University, Hefei 230036, China) Abstract: Being taken Xuyi County, Jinhu County and Hongze County in Jiangsu Province, China as examples, monitoring and forecasting of rice production were carried out by using HJ-1A satellite remote sensing images. The handhold GPS machines were used to measure the geographical position and some other information of these samples such as areas shapes. The GPS data and the interpretation mark were used to correct HJ-1 image, assist human-computer interactive interpretation, and other operations. The test data had been participated in the whole classification process. The accuracy of interpreted information on rice planting area was more than 90%. By using the leaf area index from the normalized difference vegetation index inversion, the biomass from the ratio vegetation index inversion, and combined with the rice yield estimation model, the rice yield was estimated. Further the thematic map of rice production classification was made based on the rice yield data. According to the comparison results between measured and fitted values of yields and areas of sample sites, the accuracy of the yield estimation was more than 85%. The results suggest that HJ-A/B images could basically meet the demand of rice growth monitoring and yield forecasting, and could be widely applied to rice production monitoring. Key Words: rice; yield; satellite remote sensing images; estimation model China is one of the biggest rice cultivation estimation. In recent years, some domestic researchers countries. Monitoring and forecasting rice production have made some crop yield estimation research based timely and accurately is very significance for on our satellites data and achieved preferable results adjusting planting structure and making food policy. (Qi et al, 2008; Qin et al, 2009). However, there are In the early 1990s, Chinese Academy of Sciences and few reports about rice yield estimation (Yang et al, other units, organized by the Ministry of Science and 2009). Taking the main rice producing region of Technology in China, used Landsat/TM and Jiangsu Province in China as an experimental area and NOAA/AVHRR images data to estimate planting combining with rice yield estimation model, we used areas and yields of rice, wheat and corn in main HJ-A/B images and conducted related exploratory grain-producing area. The estimation of planting area research on remote sensing yield estimation to exactly and yield of rice was conducted in Hubei and Jiangsu monitor and forecast rice planting areas and yields, Provinces, China and the prediction accuracy was which provided information for rice quantitative above 85%. The main methods and technology system management and macro-control. of crop yield assessment were preliminarily mastered by using of remote sensing data (Xia et al, 1996; Chen MATERIALS AND METHODS et al, 1997; Lobell et al, 2003). As many kinds of satellites such as land resources satellite, environmental Research regional overviews mitigation satellite, meteorological ocean satellite and other series have been successfully launched, China Being taken Xuyi County, Jinhu County and has made great progress in the sensor field of Hongze County in Jiangsu Province, China as study multi-spectral and even hyperspectral. Using our own area (Fig. 1), these regions locate at 118°12'E– satellites data to carry out related rice yield estimation 119°36'E, 32°43'N–36°06'N with temperate zone studies have become an inevitable trend of crop yield monsoon climate and four distinct seasons. It has an annual frost-free period of about 204 d, an average annual temperature of 14°C, an average annual Received: 12 August 2010; Accepted: 30 November 2010 Corresponding author: LI Wei-guo ([email protected]) precipitation of 940 mm and average annual sunshine Rice Science, Vol. 18, No. 2, 2011 was good. Geometric correction was first roughly made using 1:100 000 topographic maps, and then accurately carried out by the GPS route recording and ground truth data to make sure the alignment error within one pixel. According to the experience linear transformation, the atmospheric radiation correction and reflection conversion were obtained by using the actual reflectivity of ground calibration body and the corresponding primitive DN value of the satellite images. Fig. 1. The distribution of GPS sample sites in study area. Remote sensing yield estimation model of rice In the sun radiation energy only the visible part ranged from 2130 to 2430 h, which are better climate (350–700nm), accounting for about 47% to 48%, can and soil conditions. In sync with satellite transit time, be used for photosynthesis by plants. The ability of we established 28 sample points for the difference rice daily conversion from visible light into organic GPS fixed-point investigation and sample that included light is called solar contract efficiency (also called leaf area index and biomass. For determine dry matter, daily increase in above-ground biomass weight, sampling plants were dried at 105°C for 20 min, and DABW). Daily increase in above-ground biomass then at 75°C for constant weight. The leaf area index weight calculation referred to Gao et al (1992) was measured by the gravity method (Li and Li, 2010). simulation algorithm, the indication is the type: The grain yield was got by field surveys, which took samples by 50 m×50 m type frame according to the B ⎛ 1+ D ⎞ ΔDABWi = × Ln⎜ ⎟× DL× δ, field block diagonal line 5 points, grains in 1 m2 at K × A ⎝1+ D × Exp()− K × LAIi ⎠ 2 every sampling point with totally 5 m were collected. D = A× 0.47 × (1− α)× Qi / DL , (1) 2 They were naturally air-dried (approximate 13% water Where ΔDABWi [kg/(hm ·d)] is the i-th day of the content) and weighed. The meteorological data were daily increase in above-ground biomass weight, K is provided by the local meteorological department in the group extinction coefficient; LAIi is the i-th day of the three counties. the leaf area index; D is the middle variable; α is the rice group reflection (%); Qi is the daily global solar Data source radiation (MJ/m2); B and A is the experiment The satellite in this study is China environment coefficients, the value is 22 and 4.5 respectively; δ is disaster reduction satellite, the HJ-1 satellite for short, the conversion coefficient of CH2O and CO2 with 0.68 which includes A star and B star with an orbital value; DL is the day length (h); and LAIi at the altitude of 650 km. CCD (Charge coupled device) heading stage can be computed by the model (Y=2. 1.3655NDVI camera covers the earth every 4 days (HJ-1A and 8339×e (Li et al, 2008)). NDVI is the HJ-1B satellite network revisit cycle is 2 days over the normalized difference vegetation index, NDVI= (RNIR– global), spectrum scope covers blue light (0.43–0.52 RRED)/(RNIR+RRED), where RNIR is the near infrared μm), green light (0.52–0.60 μm), red light (0.63–6.90 reflectance (the fourth band of HJ-1A satellite), and μm) and near-infrared light (0.76–0.9 μm), the RRED is the red reflectance (the third band of HJ-1A subsatellite resolution is 30 m, the breadth of single satellite ). CCD camera is 360 km (the breadth of two cameras is Daily increase in above-ground biomass weight 710 km). removing the plant growth respiration and its The HJ-1A satellite transit time was on 26 maintenance consumption is the plant daily net August, 2009, when rice was at the heading stage. It assimilation (also called the above-ground biomass was sunny and cloudless, so the satellite image quality weight, ABW). Its algorithm is as follows: LI Wei-guo, et al. Estimating Rice Yield by HJ-1A Satellite Images Table 1. Parameters of rice materials. Where i is the days from sowing to maturity (d), Seeding rate Growth Harvest which equals to growth duration, and β is the ratio of Rice material 2 K (kg/hm ) duration (d) index root to shoot the maturity stage, generally between Liangyou 6 150 130 0.41 0.63 Liangyoupeijiu 150 140 0.43 0.67 0.05 and 0.08, the value is 0.06 in the model. The Xudao 3 150 152 0.45 0.69 parameter information of rice yield estimation model is listed in Table 1. ΔABWi = (ΔDABW i-RGi-RMi) × min(TFi, NF ) (2) Where RG and RM are the photosynthesis i i RESULTS assimilation amount [kg/(hm2·d)]. They are growth breath consumption [kg/(hm2·d)] and maintenance Forecast and confirmation of leaf area index and breath consumption [kg/(hm2·d)] of the i-th day biomass respectively. TFi and NF are influence factor of above- ground biomass accumulation from air temperature The values of RVI and NDVI and the information and soil nitrogen respectively. Its algorithm was of the 28 sample points were extracted through the described by Li et al (2008). ERDAS and ENVI softwares, and the LAI and During the rice growing period, the above- biomass in each sample point were calculated by an ground biomass weight is the sum of dry matter inversion model. The rice LAI from inversion was low, accumulation. ABWi is the total above-ground dry with the root mean square error (RMSE) of 0.38 (Fig. biomass accumulation (kg/hm2) of the i-th day from 2-A), which indicated that the data was comparatively the first emergence day to harvest.
Details
-
File Typepdf
-
Upload Time-
-
Content LanguagesEnglish
-
Upload UserAnonymous/Not logged-in
-
File Pages6 Page
-
File Size-