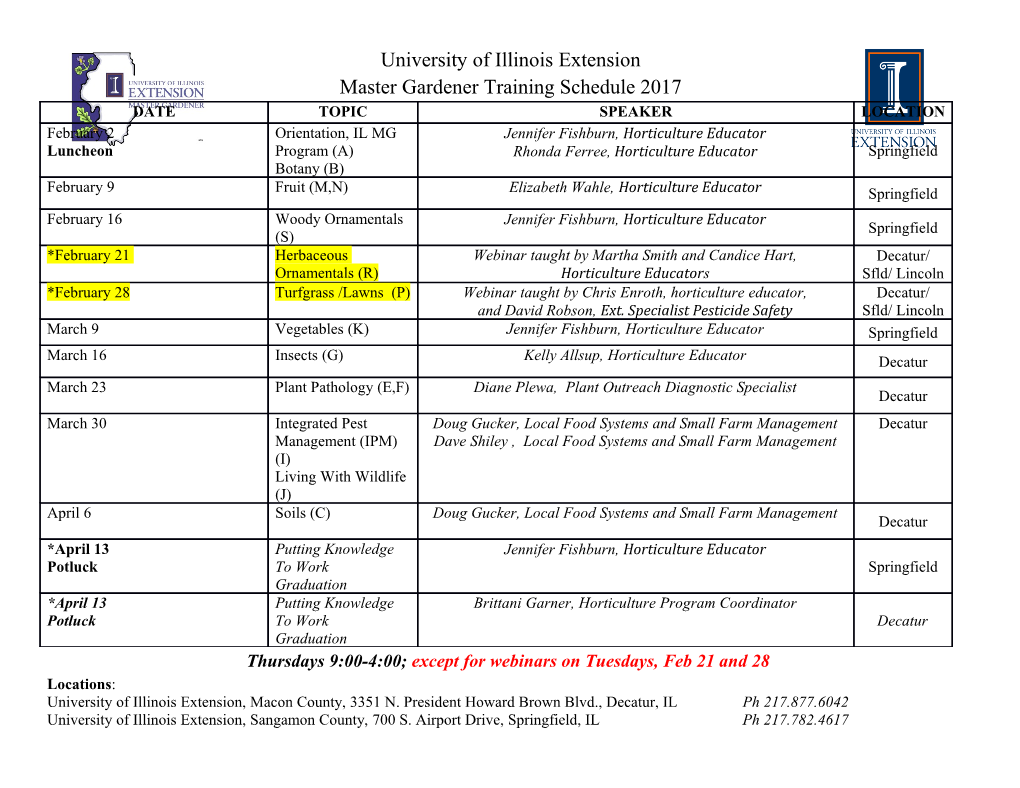
An Double Hidden HMM and an CRF for Segmentation Tasks with Pinyin’s Finals Huixing Jiang Zhe Dong Center for Intelligence Science and Technology Beijing University of Posts and Telecommunications Beijing, China [email protected] [email protected] Abstract inner features of Chinese Characters. And it nat- urally contributes to the identification of Out-Of- We have participated in the open tracks Vacabulary words (OOV). and closed tracks on four corpora of Chi- In our work, Chinese phonology information is nese word segmentation tasks in CIPS- used as basic features of Chinese characters in all SIGHAN-2010 Bake-offs. In our experi- models. For open tracks, we propose a new dou- ments, we used the Chinese inner phonol- ble hidden layers HMM in which a new phonol- ogy information in all tracks. For open ogy information is built in as a hidden layer, a tracks, we proposed a double hidden lay- new lexical association is proposed to deal with ers’ HMM (DHHMM) in which Chinese the OOV questions and domains’ adaptation ques- inner phonology information was used as tions. And for closed tracks, CRF model has been one hidden layer and the BIO tags as an- used , combined with Chinese inner phonology in- other hidden layer. N-best results were formation. We used the CRF++ package Version firstly generated by using DHHMM, then 0.43 by Taku Kudo1. the best one was selected by using a new In the rest sections of this paper, we firstly in- lexical statistic measure. For close tracks, troduce the Chinese phonology in Section 2. Then we used CRF model in which the Chinese in the Section 3, the models used in our tasks are inner phonology information was used as presented. And the experiments and results are features. described in Section 4. Finally, we give the con- clusions and make prospect on future work. 1 Introduction 2 Chinese Phonology Chinese language has many characteristics not possessed by other languages. One obvious is Hanyu Pinyin is the form of sound for Chi- that the written Chinese text does not have explicit nese text and the Chinese phonology informa- word boundaries like western languages. So word tion is explicit expressed by Pinyin. It is cur- segmentation became very significative for Chi- rently the most commonly used romanization sys- nese information processing, and is usually con- tem for Standard Mandarin. Hanyu means the sidered as the first step of any further processing. Chinese language, and Pinyin means “phonetics”, Identifying words has been a basic task for many or more literally, “spelling sound” or “spelled researchers who have devoted themselves on Chi- sound” (wikipedia, 2010). The system has been nese text processing. employed to teach Mandarin as home language The biggest characteristic of Chinese language or as second language by China, Malaysia, Sin- is its trinity of sound, form and meaning (Pan, gapore et.al. Pinyin has been the most Chinese 2002). Hanyu Pinyin is the form of sound for character’s input method for computers and other Chinese text and the Chinese phonology informa- devices. tion is explicit expressed by Pinyin which is the 1http://crfpp.sourceforge.net/ The romanization system was developed by a 3.1 Double hidden layers’ HMM government committee in the People’s Repub- For a given piece of Chinese sentence, X = lic of China, and approved by the Chinese gov- x1x2 : : : xT , where xi; i = 1;:::;T is a Chinese ernment on February 11, 1958. The Interna- character. Suppose that we can give each Chinese tional Organization for Standardization adopted character xi a Pinyin’s final yi. And suppose the pinyin as the international standard in 1982, and label sequence of X is S = s1s2 : : : sT , where since then it has been adopted by many other si 2 TS is the tag of xi. Then what we want to organizations(wikipedia, 2010). In this system, find is an optimal tag sequence S∗ which is de- pinyin is composed by initials(pinyin: shengmu), fined in (1). finals(pinyin: yunmu) and tones(pinyin: sheng- ∗ diao) instead of consonants and vowels used in S = arg max P (S; Y jX) S European language. For example, the Pinyin of = arg max P (XjS; Y )P (S; Y ) (1) ”中” is ”zhong1” composed by ”zh”, ”ong” and S ”1”. In which ”zh” is initial, ”ong” is final and The model is described in Fig. 1. For a given ”1” is the tone. piece of Chinese character strings, One hidden Every language has its rhythm and rhyme, so layer is label sequence S. Another hidden layer is Chinese is no exception. The rhythm system are Pinyin’s finals sequence Y . The observation layer the driving force from the unconscious habit of is the given piece of Chinese characters X. language(Edward, 1921). And the Pinyin’s finals contribute the Chinese rhythm system, Which is the basic assumption our research based on. 3 Algorithms Generally the task of segmentation can be viewed as a sequence labeling problem. We first define a tag set as TS = fB, I, E, Sg, shown in Table 1. Figure 1: Double Hidden Markov Model For transition probability, second-order Markov Table 1: The tag set used in this paper. model is used to estimate probability of the double Label Explanation hidden sequences as described in (2). Y B beginning character of a word P (S; Y ) = p(st; ytjst−1; yt−1) (2) I inner character of a word t E end character of a word For emission probability, we keep the first- S a single character as a word order Markov assumption as shown in (5). Y P (XjS; Y ) = p(xtjst; yt) (3) For the piece ”是 英 国 前 王 妃 戴 安 娜” of t the example described in the experiments section, 3.1.1 Nbest results firstly, the TS tags are labeled to it. And its re- Based on the work of (Jiang, 2010), a word lat- sult is ”是/S 英/B 国/E 前/S 王/B 妃/E 戴/B 安/I tice is also built firstly, then in the second step, the 娜/E”. Then the tags are combined sequentially to backward A∗ algorithm is used to find the top N get the finally result ”是 英国 前 王妃 戴安娜”. results instead of using the backward viterbi al- In this section, A novel HMM solution is pre- gorithm to find the top one. The backward A∗ sented firstly for open tracks. Then the CRF solu- search algorithm is described as follow (Wang, tion for closed tracks is introduced. 2002; Och, 2001). 3.1.2 Reranking with a new lexical statistic tation(Lafferty, 2001; Zhao, 2006). In the closed measure tracks of the paper, we also use it. Given two random Chinese characters X and Y and assume that they appears in an aligned region 3.2.1 Feature templates of the corpus. The distribution of the two random We adopted two main kinds of features: n-gram Chinese characters could be depicted by a 2 by 2 features and Pinyin’s finals features. The n-gram contingency table shown in Fig. 2(Chang, 2002). feature set is quite orthodox, they are, namely, C- 2, C-1, C0, C1, C2, C-2C-1, C-1C0, C0C1, C1C2. The Pinyin’s finals feature set is the same as n- gram feature set. They are described in Table. 2. Figure 2: A 2 by 2 contingency table Table 2: Feature templates In Fig. 2, a is the counts of X and Y co-occur; b is the counts of the cases that X occurs but Y does Templates Category not; c is the counts of the cases that X does not occur but Y does; d is the counts of the cases that C-2, C-1, C0, C1, C2 N-gram: Unigram both X and Y do not occur. The Log-likelihood C-2C-1, C-1C0, C0C1, C1C2 N-gram: Bigram rate is calculated by (4). P-2, P-1, P0, P1, P2 Phonetic: Unigram P-2P-1, P-1P0, P0P1, P1P2 Phonetic: Bigram a · N LLR(x; y) = 2(a · log (a + b) · (a + c) b · N + b · log (a + b) · (b + d) 4 Experiments and Results c · N + c · log (c + d) · (a + c) 4.1 Dataset d · N + d · log ) (4) We build a basic words dictionary for DHHMM (c + d) · (b + d) and a Pinyin’s finals dictionary for both DHHMM For the N-best result described in sec. 3.1.1, and CRF from The Grammatical Knowledge-base they can be re-ranked by (5). of Contemporary Chinese(Yu, 2001). For the fi- nals dictionary, we give each Chinese character a XK final extracted from its Pinyin. When it comes to ∗ λ S = arg min(scoreh(S)+ LLR(xk; yk)) a polyphone, we just combine its all finals simply S K k=1 to one. For example, ”中fongg”, ”差fa&ai&ig”. (5) The training corpus (5,769 KB) we used is the where scoreh is the negative log value of P (S; Y jX). K is the number of breaks in X and Labeled Corpus provided by the organizer. We firstly add the Pinyin’s finals to each Chinese xk is the left Chinese character of the k break character of it, then we train the parameters of and yk is the right Chinese character of the k break. λ is the regulatory factor(in our experi- DHHMM and CRF model on it. ments λ = 0:45). And the test corpus contains four domains: Lit- Bigger value of LLR(xk; yk) means stronger erature (A), Computer (B), Medicine (C) and Fi- ability in combining of the two characters xk and nance(D).
Details
-
File Typepdf
-
Upload Time-
-
Content LanguagesEnglish
-
Upload UserAnonymous/Not logged-in
-
File Pages5 Page
-
File Size-