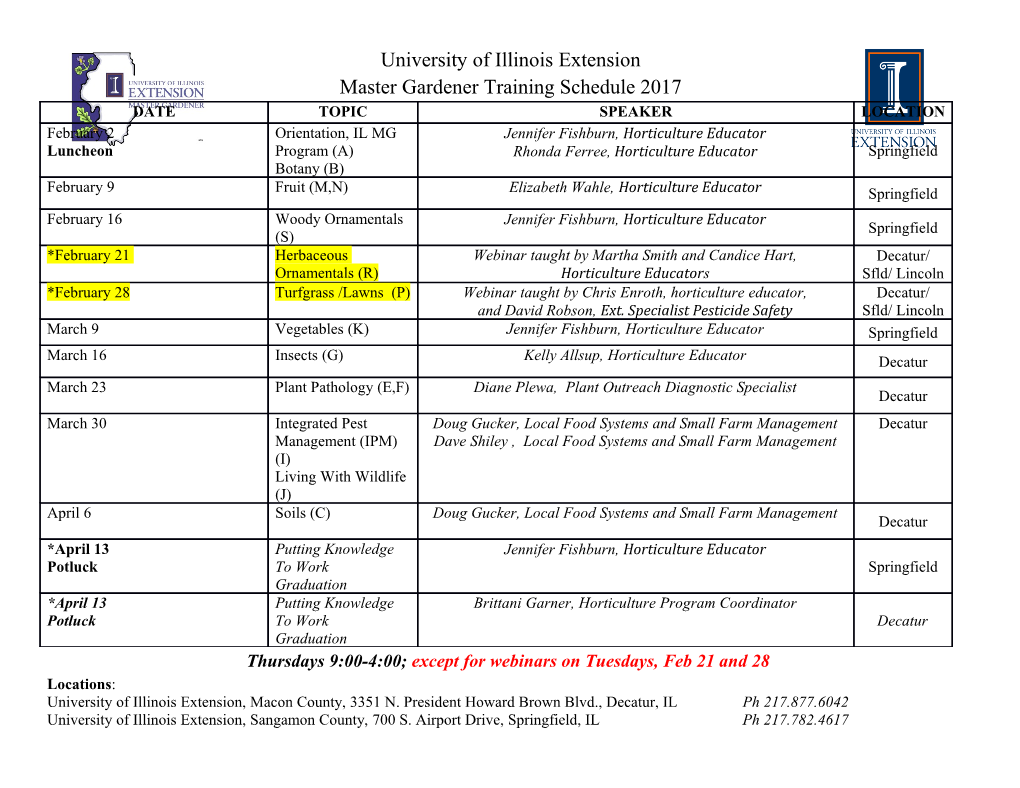
Hacettepe Journal of Mathematics and Statistics Volume 46 (5) (2017), 865 874 Investigating an overdetermined system of linear equations by using convex functions Zlatko Pavi¢ ∗ y and Vedran Novoselac z Abstract The paper studies the application of convex functions in order to prove the existence of optimal solutions of an overdetermined system of lin- ear equations. The study approaches the problem by using even convex functions instead of projections. The research also relies on some spe- cial properties of unbounded convex sets, and the lower level sets of continuous functions. Keywords: overdetermined system, convex function, global minimum. 2000 AMS Classication: 15A06, 26B25. Received : 30.08.2016 Accepted : 19.12.2016 Doi : 10.15672/ HJMS.2017.423 1. Introduction We consider a system of m linear equations with n unknowns over the eld of real numbers given by a11x1 + ::: + a1nxn = b1 . (1.1) . .. : am1x1+ ::: + amnxn = bm Including the matrices 2 a11 : : : a1n 3 2 x1 3 2 b1 3 . (1.2) 6 . .. 7 6 . 7 6 . 7 A = 4 . 5 ; x = 4 . 5 ; b = 4 . 5 ; am1 : : : amn xn bm the given system gets the matrix form (1.3) Ax = b: ∗Department of Mathematics, Mechanical Engineering Faculty in Slavonski Brod, University of Osijek, Croatia, Email: [email protected] yCorresponding Author. zDepartment of Mathematics, Mechanical Engineering Faculty in Slavonski Brod, University of Osijek, Croatia, Email: [email protected] 866 n m Identifying the matrix A with a linear operator from R to R , the column matrix x n m with a vector of R , and the column matrices Ax and b with vectors of R , the given system takes the operator form. If the vector b is not contained in the image R(A) of the operator A, then the equation in formula (1.3) has no solution. Respectively, the system in formula (1.1) is said to be inconsistent. A system of linear equations is said to be overdetermined if there are more equations than unknowns. The overdetermined system with linearly independent equations is evidently inconsistent. n m To summarize, employing a linear operator A : R ! R where n < m, and a vector m n b 2 R , we receive the equation Ax = b with unknown vectors x 2 R . If b 2 R(A), the set of solutions is the preimage of b as (−1) n (1.4) A (b) = fx 2 R : Ax = bg: If b2 = R(A), the equation has no solution. To include both cases, we use a norm on the m n space R , and the function f : R ! R dened by (1.5) f(x) = kAx − bk: Then we are looking for the global minimum points x of the function f. Expressed as an equation, it is n or f(x) = miny2R f(y) (1.6) kAx − bk = min kAy − bk; n y2R and its solutions are called optimal solutions of the equation Ax = b respecting the given norm. The above equation is reduced to Ax = b if b 2 R(A). The existence of the solution of the minimization problem in formula (1.6) is provided in the framework of the theory of projections. In order to remake it without using projections, we will utilize even convex functions. Methodical introduction to overdetermined systems of linear equations can be seen in [12]. The general insight into the problem of least absolute deviations can be found in [1]. Linear optimization problems were presented in [2]. 2. Anity and convexity, rays, lower level sets Let X be a real vector space. Let x1; x2 2 X be vectors, and let t1; t2 2 R be coecients. Then the linear combination t1x1 + t2x2 is said to be ane (convex) if t2 = 1 − t1 (0 ≤ t2 = 1 − t1 ≤ 1). A set S ⊆ X is said to be ane (convex) if it contains all binomial ane (convex) combinations of its vectors. (−1) n The set A (b) ⊆ R in formula (1.4) is ane. In general, if a set B ⊆ R(A) is ane (−1) n (convex), then its preimage A (B) ⊆ R is ane (convex) too. Let S be an ane (convex) set. A function f : S ! R is said to be ane (convex) if the equality (inequality) (2.1) f t1x1 + t2x2 = (≤) t1f(x1) + t2f(x2) holds for all ane (convex) combinations t1x1 + t2x2 of points x1; x2 2 S. If x1; x2 2 X are vectors, then the ray or half-line R from x1 passing through x2 is the subset of X dened as R = (1 − t)x1 + tx2 : t ≥ 0 : If f : S ! R is a function, then the lower level set L of the function f with a height l 2 R is the subset of S dened as L = fx 2 S : f(x) ≤ lg: 867 The lower level sets of a convex function are convex. The following lemma can be found in [3, Theorem 1, page 23]. We oer the proof by using convex combinations. n 2.1. Lemma. Let S ⊆ R be an unbounded closed convex set, and let s 2 S be a point. Then there is a ray from s belonging to S. n Proof. Without loss of generality, using the translation in the space R by the vector −s, we can assume that the set S contains the origin o. So, we are looking for a ray from o contained in S. Since S is unbounded, we can pick out a sequence (yk)k of points yk 2 S such that for every . kykk = k k 2 N Since S is convex, each line segment between o and yk is contained in S. Then it follows that the convex combination k − 1 1 1 x = o + y = y k k k k k k belongs to for every . Each satises . We have the sequence of S k 2 N xk kxkk = 1 (xk)k points belonging to the intersection of the set and the unit sphere in n. The xk S0 S R set S0 is compact because it is closed and bounded, and therefore the sequence (xk)k has a subsequence converging to some point . (xrk )k x0 2 S0 Let R be the ray from o passing through x0. We will verify that R is contained in S. Since R = ftx0 : t ≥ 0g, we have to show that each ray point tx0 is in S. Let t ≥ 0 be a nonnegative coecient. Since S is convex, the points t rk − t t txrk = yrk = o + yrk rk rk rk are in S for rk ≥ t. The above points approach tx0 if k approaches innity. Since S is closed, the limit is in . tx0 S The proof of Lemma 2.1 is illustrated in Figure 1, and the same is still applicable to n an unbounded convex set of R and points of its interior. 2 Figure 1. A ray in the unbounded convex set of R Statements similar to the next lemma and corollary are exposed in [11, Theorem 8.4, Theorem 8.5 and Theorem 8.6, pages 30-31]. Bounded sets play a decisive role for the global minimum existence. Considering lower level sets and the global minimum of a continuous function, one can nd the following. 868 n 2.2. Lemma. Let f : R ! R be a continuous function whose lower level sets are bounded. Then f has the global minimum. n Proof. First we take a point x0 2 R , and a number l ≥ f(x0). Then we specify the lower level set L of the function f with the height l, which is nonempty. The set L is bounded by the assumption, and closed by the continuity of f. Thus L is compact, and ∗ ∗ ∗ there is a point x 2 L such that f(x ) = minx2L f(x). The value f(x ) is the global minimum, which is easy to demonstrate as follows. n ∗ Let x 2 R be a point. If x 2 L, then applies f(x ) ≤ f(x) ≤ l. If x2 = L, then we ∗ have f(x ) ≤ l < f(x). n A convex function on the space R approaching innity on all rays from the origin being examined. n 2.3. Corollary. Let f : R ! R be a convex function such that (2.2) lim f(tx) = 1 t!1 n for every x 2 R n fog. Then f has the global minimum. n Proof. Since f is convex on R , it is also continuous (a convex function on an open domain is continuous). Respecting the continuity of f in the context of Lemma 2.2, it is sucient to prove that the lower level sets of f are bounded. Let L be the lower level set of f with a height l ≥ f(o). The set L contains the origin o, and it is closed and convex. If L is not bounded, then it contains some ray R from the origin o by Lemma 2.1. Thus, a point x0 2 L n fog exists such that the ray R = ftx0 : t ≥ 0g. Since R ⊆ L, for each t ≥ 0 we have that f(tx0) ≤ l: n It follows that the condition in formula (2.2) does not apply to x0 2 R n fog. Hence the lower level set L must be bounded. Consequently, all lower level sets of the function f are bounded. Very well written and motivated book in [9] can be recommended as an introduc- tory course to the analysis of convex functions. One chapter of this book refers to the optimization. The usage of ane and convex combinations is important in the eld of mathematical inequalities.
Details
-
File Typepdf
-
Upload Time-
-
Content LanguagesEnglish
-
Upload UserAnonymous/Not logged-in
-
File Pages10 Page
-
File Size-