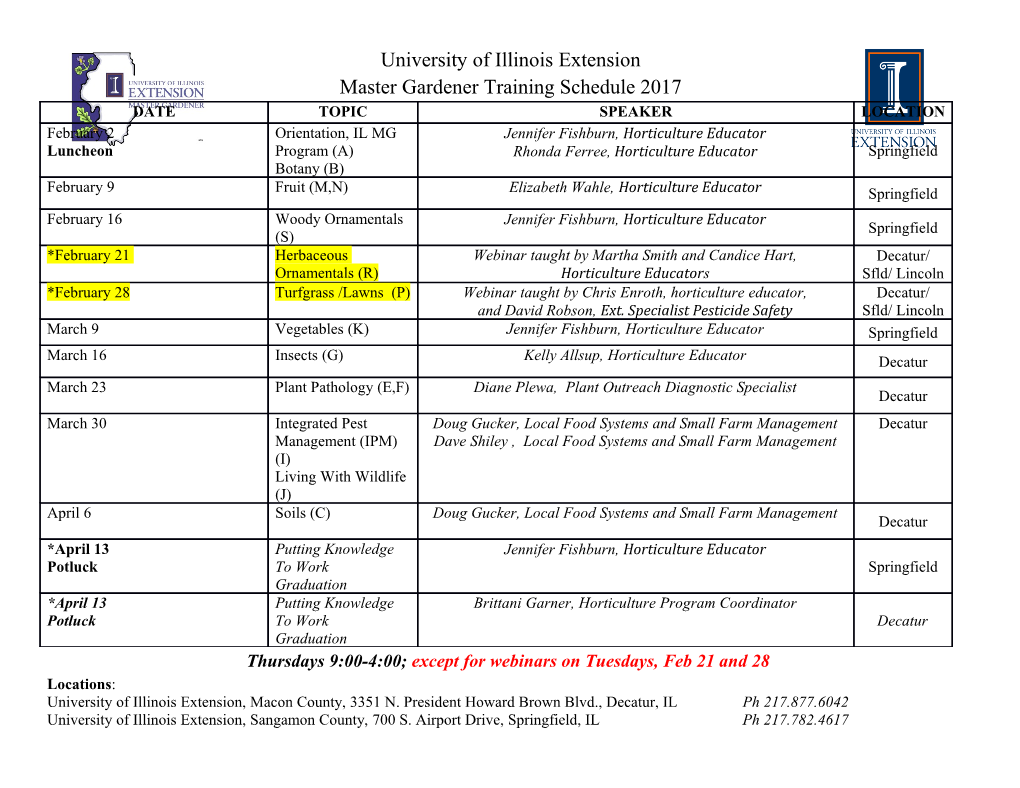
EDUCATION • ÉDUCATION METHODOLOGY Understanding linear and logistic regression analyses Andrew Worster, MD, MSc;*† Jerome Fan, MD;* Afisi Ismaila, MSc† SEE RELATED ARTICLE PAGE 105 egression analysis, also termed regression modeling, come). For example, a researcher could evaluate the poten- Ris an increasingly common statistical method used to tial for injury severity score (ISS) to predict ED length-of- describe and quantify the relation between a clinical out- stay by first producing a scatter plot of ISS graphed against come of interest and one or more other variables. In this ED length-of-stay to determine whether an apparent linear issue of CJEM, Cummings and Mayes used linear and lo- relation exists, and then by deriving the best fit straight line gistic regression to determine whether the type of trauma for the data set using linear regression carried out by statis- team leader (TTL) impacts emergency department (ED) tical software. The mathematical formula for this relation length-of-stay or survival.1 The purpose of this educa- would be: ED length-of-stay = k(ISS) + c. In this equation, tional primer is to provide an easily understood overview k (the slope of the line) indicates the factor by which of these methods of statistical analysis. We hope that this length-of-stay changes as ISS changes and c (the “con- primer will not only help readers interpret the Cummings stant”) is the value of length-of-stay when ISS equals zero and Mayes study, but also other research that uses similar and crosses the vertical axis.2 In this hypothetical scenario, methodology. the overlap of the line and the data points in Figure 2 Linear regression 80 The most common type of regression analysis is linear re- 70 gression which, as its name implies, assumes that a linear 60 relation exists between the dependent variable (i.e., the 50 variable that the researchers are trying to predict, also known as the response or outcome variable) and the inde- 40 pendent variable(s) that the researchers choose to evaluate 30 (i.e., the known or hypothesized predictor variable[s]). Lin- Outcome Variable 20 ear regression produces a mathematical equation (or 10 “model”) for a “best fit” line to describe the relation. This can be portrayed visually by a scatter plot with a line run- 0 0 20 40 60 80 100 ning through it as shown in Figure 1.2 To be suitable for linear regression, the outcome of inter- Predictor Variable est must be a "continuous" variable (that is, a variable with Fig. 1. A scatter plot depicting the linear relation between a continuous numerical range rather than a categorical out- the outcome and predictor variables. From the *Division of Emergency Medicine, McMaster University, Hamilton, Ont. and the †Department of Clinical Epidemiology, McMaster University, Hamilton, Ont. Received: Feb. 1, 2007; accepted: Feb. 2, 2007 This article has not been peer reviewed. Can J Emerg Med 2007;9(2):111-3 March • mars 2007; 9 (2) CJEM • JCMU 111 Downloaded from https://www.cambridge.org/core. IP address: 170.106.33.42, on 29 Sep 2021 at 12:28:05, subject to the Cambridge Core terms of use, available at https://www.cambridge.org/core/terms. https://doi.org/10.1017/S1481803500014883 Worster et al demonstrate a perfect positive relation between the 2 vari- • coefficient of determination (R2), another dimension- ables, indicating that ISS can be used as a single predictor less quantity ranging from 0% to 100%, that measures of ED length-of-stay. Suppose, however, that ISS has no the proportion of variation in the outcome variable ex- impact on ED length-of-stay. The line would then have a plained by the regression model (R2 = 100% in Figure slope of zero, as shown in Figure 3, and the intersection of 2, R2 = 0% in Figure 3, and R2 = 8% in Figure 4) and; the line itself with the Y axis would represent the mean • analysis of variance (ANOVA), a global test of signifi- length-of-stay value (c = 14.3). Figure 4 represents a situa- cance of linear association in which p < 0.05 generally tion in between these 2 extremes and suggests that while implies a linear association between outcome and pre- ISS likely impacts ED length-of-stay, the scatter of data dictor variables. points around the best fit line makes it is difficult to know Linear regression analysis also provides estimates of re- if the apparent magnitude of the relations is real. Linear re- gression variables and test of significance for each variable. gression analysis addresses this by measuring the propor- tion of the variability in ED length-of-stay that the model, Logistic regression represented by the fitted line, explains.3 Simple linear regression is used when there is only a sin- Logistic regression is similar to multivariate linear regres- gle continuous predictor variable and a single continuous sion in that it creates a model to describe the impact of outcome variable. Multivariate (or multiple) linear regres- multiple predictors on a single response variable. However, sion is used to produce a model with 2 or more continuous in logistic regression, the outcome variable must be cate- or categorical predictor variables and a continuous out- gorical (usually dichotomous, i.e., with 2 possible out- come variable. For example, a researcher might also want to determine whether sex (a categorical variable) and age 25 (a continuous variable) are predictors of ED length-of-stay. By incorporating these variables, along with ISS, multi- 20 variate linear regression can produce a more complete S 15 model and, thus, a better understanding of the independent 10 impact of different predictor variables on the outcome, as ED LO well as any potential interaction between the predictor 5 ED LOS = 14.29 variables themselves.4 R2 =0% Some of the important terms in the statistical outputs of 0 a linear regression analysis include: 010203040 5060 70 80 ISS • coefficient of correlation (R), a dimensionless quantity ranging from –1 to +1 that describes the strength of the Fig. 3. With a slope of 0, the Injury Severity Score has no im- association between 2 variables (R = 1 in Figure 2, R = pact on emergency department length-of-stay (LOS). 0 in Figure 3, and R = 0.28 in Figure 4); 40 100 35 90 ED LOS = 1.2(ISS) + 1 2 80 R = 100% 30 70 S 25 S 60 50 20 40 ED LO ED LO 15 30 ED LOS = 0.0716(ISS) + 23.961 20 10 R2 =8.0% 10 5 0 0 010203040 5060 70 80 0 102030405060708090 ISS ISS Fig. 2. A perfect positive relation between the Injury Sever- ity Score (ISS) and emergency department (ED) length-of- Fig. 4. This data set suggests that while Injury Severity Score stay (LOS) (i.e., the ISS can be used as a single predictor of likely has an impact on emergency department length-of-stay ED LOS). (LOS), the apparent magnitude of the relation is uncertain. 112 CJEM • JCMU March • mars 2007; 9 (2) Downloaded from https://www.cambridge.org/core. IP address: 170.106.33.42, on 29 Sep 2021 at 12:28:05, subject to the Cambridge Core terms of use, available at https://www.cambridge.org/core/terms. https://doi.org/10.1017/S1481803500014883 Linear and logistic regression analyses comes, such as death or survival, although special tech- variable. To answer this they used logistic regression. niques allow further categorized data to be modelled). A Again, the researchers found that TTL has no significant continuous outcome variable can be converted to a cate- impact (p = 0.58). This was further demonstrated by the gorical one in order for logistic regression to be used, but ORs for each of the TTL categories, the confidence inter- collapsing continuous variables in this manner is generally vals of which included a value of one. discouraged as it reduces precision. If this is done, the cat- In considering the Cummings and Mayes study, the au- egories should not be arbitrary but rather must make clini- thors appear to have correctly identified which variables cal sense. Unlike linear regression, the predictor variables were predictors and which were outcomes. However, it re- in logistic regression do not need to be linearly related, mains conceivable that there might be other predictor vari- normally distributed or have equal variance within each ables, not included in the model, that have a statistically group. Because the relation between the predictor and out- significant impact on the response variables. For example, come variables is not presumed to be a linear function, the hospital overcrowding, resource availability and quality of measure of association between the outcome of interest care indicators could all be influential. As previously men- and the predictor variable is represented by an odds ratio tioned, failure to include all possible predictors in a study (OR) instead of a multiplicative factor. Comparisons of can weaken a regression model. ORs between predictor variables help determine the factors of greatest importance, while their confidence intervals in- Summary dicate their statistical significance. While the mathematical concepts and details of statistical Limitations tests can appear complex, most of the issues involved are straightforward. By understanding some basic statistical Readers should be cautious when interpreting the results of concepts and the role of the different statistical tests, one regression analyses, and several issues must be considered. can usually determine whether the method of analysis for First, researchers occasionally mistake a predictor variable any given study was appropriate. Regression analysis is a for the outcome variable or vice versa (and so the model powerful statistical method to determine which variables must make clinical sense).5 Second, the determination of a are predictors of an outcome and the magnitude of that re- statistically significant relation between the predictor and lation.
Details
-
File Typepdf
-
Upload Time-
-
Content LanguagesEnglish
-
Upload UserAnonymous/Not logged-in
-
File Pages3 Page
-
File Size-