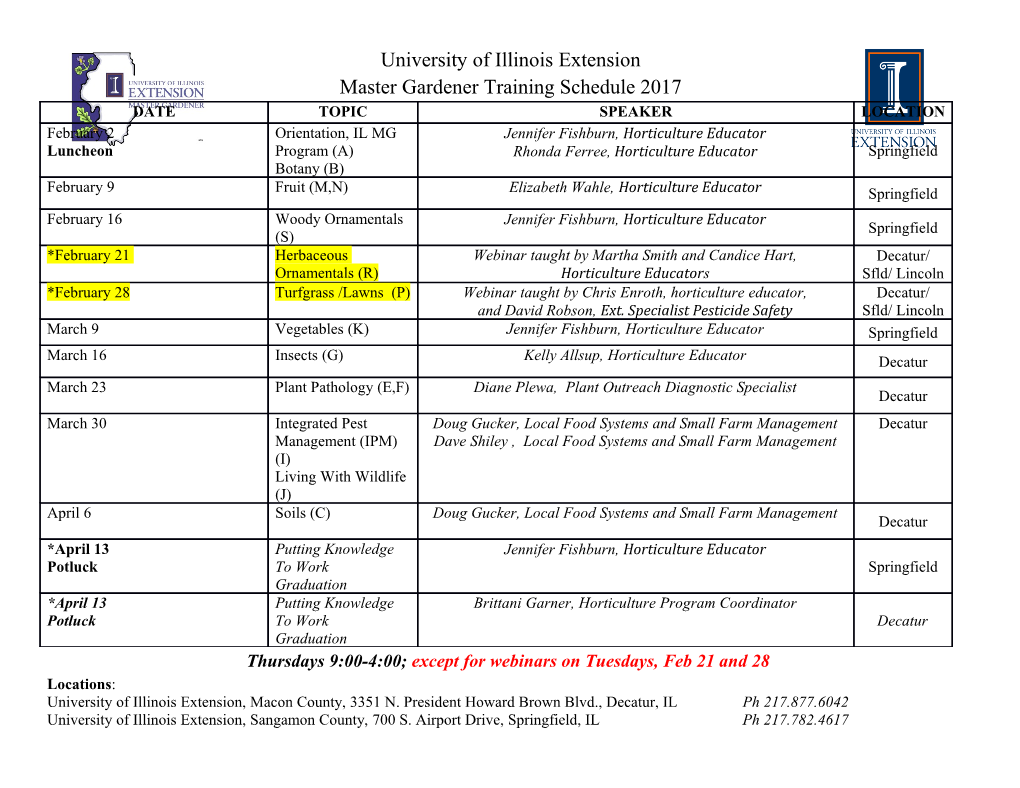
No d’ordre : No attribué par la bibliothèque : -UNIVERSITÉ PARIS-SUD - Laboratoire de Recherche en Informatique - UMR 427 - LRI THÈSE en vue d’obtenir le grade de Docteur de l’Université Paris-Sud Spécialité : Informatique au titre de l’École Doctorale Informatique Paris-Sud présentée et soutenue publiquement le 11 Février 2013 par Amal KHABOU Dense matrix computations : communication cost and numerical stability. Directeur de thèse : Docteur Laura GRIGORI Après avis de : Professeur Nicholas J. HIGHAM Rapporteur Professeur Yves ROBERT Rapporteur Devant la commission d’examen formée de : Professeur Iain DUFF Examinateur Professeur Yannis MANOUSSAKIS Examinateur Enseignant-chercheur Jean-Louis ROCH Examinateur ii Abstract: This dissertation focuses on a widely used linear algebra kernel to solve linear systems, that is the LU decomposition. Usually, to perform such a computation one uses the Gaussian elimination with partial pivoting (GEPP). The backward stability of GEPP depends on a quantity which is referred to as the growth factor, it is known that in general GEPP leads to modest element growth in practice. However its parallel version does not attain the communication lower bounds. Indeed the panel factorization rep- resents a bottleneck in terms of communication. To overcome this communication bottleneck, Grigori et al [60] have developed a communication avoiding LU factorization (CALU), which is asymptotically optimal in terms of communication cost at the cost of some redundant computation. In theory, the upper bound of the growth factor is larger than that of Gaussian elimination with partial pivoting, however CALU is stable in practice. To improve the upper bound of the growth factor, we study a new pivoting strategy based on strong rank revealing QR factorization. Thus we develop a new block algorithm for the LU factorization. This algorithm has a smaller growth factor upper bound compared to Gaussian elimination with partial pivoting. The strong rank revealing pivoting is then combined with tournament pivoting strategy to produce a communication avoiding LU factorization that is more stable than CALU. For hierarchical systems, multiple levels of parallelism are available. However, none of the previously cited methods fully exploit these hierarchical systems. We propose and study two recursive algorithms based on the communication avoiding LU algorithm, which are more suitable for architectures with multiple levels of parallelism. For an accurate and realistic cost analysis of these hierarchical algo- rithms, we introduce a hierarchical parallel performance model that takes into account processor and network hierarchies. This analysis enables us to accurately predict the performance of the hierarchical LU factorization on an exascale platform. Keywords: LU factorization, Gaussian elimination with partial pivoting, growth factor, strong rank re- vealing QR factorization, minimizing the communication cost, parallel algorithms, hierarchi- cal systems, performance models, pivoting strategies. Résumé : Cette thèse traite d’une routine d’algèbre linéaire largement utilisée pour la résolution des systèmes li- néaires, il s’agit de la factorisation LU. Habituellement, pour calculer une telle décomposition, on utilise l’élimination de Gauss avec pivotage partiel (GEPP). La stabilité numérique de l’élimination de Gauss avec pivotage partiel est caractérisée par un facteur de croissance qui est reste assez petit en pratique. Toutefois, la version parallèle de cet algorithme ne permet pas d’atteindre les bornes inférieures qui ca- ractérisent le coût de communication pour un algorithme donné. En effet, la factorisation d’un bloc de colonnes constitue un goulot d’étranglement en termes de communication. Pour remédier à ce problème, Grigori et al [60] ont développé une factorisation LU qui minimise la communication(CALU) au prix de quelques calculs redondants. En théorie la borne supérieure du facteur de croissance de CALU est plus grande que celle de l’élimination de Gauss avec pivotage partiel, cependant CALU est stable en pratique. Pour améliorer la borne supérieure du facteur de croissance, nous étudions une nouvelle stra- tégie de pivotage utilisant la factorisation QR avec forte révélation de rang. Ainsi nous développons un nouvel algorithme pour la factorisation LU par blocs. La borne supérieure du facteur de croissance de cet algorithme est plus petite que celle de l’élimination de Gauss avec pivotage partiel. Cette stratégie de pivotage est ensuite combinée avec le pivotage basé sur un tournoi pour produire une factorisation LU qui minimise la communication et qui est plus stable que CALU. Pour les systèmes hiérarchiques, plusieurs niveaux de parallélisme sont disponibles. Cependant, aucune des méthodes précédemment ci- tées n’exploite pleinement ces ressources. Nous proposons et étudions alors deux algorithmes récursifs qui utilisent les mêmes principes que CALU mais qui sont plus appropriés pour des architectures à plu- sieurs niveaux de parallélisme. Pour analyser d’une façon précise et réaliste les coûts de ces algorithmes hiérarchiques, nous introduisons un modèle de performance hiérarchique parallèle qui prend en compte aussi bien l’organisation hiérarchique des processeurs et la hiérarchie réseau. Cette analyse nous permet de prédire d’une manière réaliste la performance de ces factorisations hiérarchiques sur une plate-forme exascale. Mots-clés : Factorisation LU, élimination de Gauss avec pivotage partiel, facteur de croissance, facto- risation QR avec forte révélation de rang, minimisation de la communication, algorithmes parallèles, systèmes hiérarchiques, modèles de performance, stratégies de pivotage. Contents Introduction i 1 High performance numerical linear algebra1 1.1 Introduction.........................................1 1.2 Direct methods.......................................1 1.2.1 The LU factorization................................2 1.2.2 The QR factorization................................4 1.3 Communication "avoiding" algorithms...........................6 1.3.1 Communication...................................7 1.3.2 A simplified performance model.........................7 1.3.3 Theoretical lower bounds..............................8 1.3.4 Motivation case: LU factorization as in ScaLAPACK (pdgetrf)..........9 1.3.5 Communication avoiding LU (CALU)....................... 10 1.3.6 Numerical stability................................. 11 1.4 Parallel pivoting strategies................................. 12 1.5 Principles of parallel dense matrix computations...................... 13 1.5.1 Parallel algorithms................................. 14 1.5.2 Tasks decomposition and scheduling techniques.................. 14 1.6 Parallel performance models................................ 18 1.6.1 PRAM model.................................... 19 1.6.2 Network models.................................. 19 1.6.3 Bridging models.................................. 19 1.6.4 Discussion..................................... 21 2 LU factorization with panel rank revealing pivoting 23 2.1 Introduction......................................... 23 2.2 Matrix algebra........................................ 24 2.2.1 Numerical stability................................. 29 2.2.2 Experimental results................................ 30 2.3 Conclusion......................................... 35 3 Communication avoiding LU factorization with panel rank revealing pivoting 37 3.1 Introduction......................................... 37 3.2 Communication avoiding LU_PRRP............................ 39 3.2.1 Matrix algebra................................... 39 3.2.2 Numerical Stability of CALU_PRRP....................... 43 3.2.3 Experimental results................................ 45 iii iv CONTENTS 3.3 Cost analysis of CALU_PRRP............................... 53 3.4 Less stable factorizations that can also minimize communication............. 56 3.5 Conclusion......................................... 59 4 Avoiding communication through a multilevel LU factorization 61 4.1 Introduction......................................... 61 4.2 LU factorization suitable for hierarchical computer systems................ 63 4.2.1 Multilevel CALU algorithm (2D multilevel CALU)............... 64 4.2.2 Numerical stability of multilevel CALU...................... 66 4.3 Recursive CALU (1D multilevel CALU)......................... 75 4.3.1 Recursive CALU algorithm............................ 75 4.4 Performance model of hierarchical LU factorizations................... 77 4.4.1 Cost analysis of 2-level CALU.......................... 78 4.4.2 Cost analysis of 2-recursive CALU........................ 79 4.5 Multilevel CALU performance............................... 80 4.5.1 Implementation on a cluster of multicore processors............... 80 4.5.2 Performance of 2-level CALU........................... 81 4.6 Conclusion......................................... 84 5 On the performance of multilevel CALU 87 5.1 Introduction......................................... 87 5.2 Toward a realistic Hierarchical Cluster Platform model (HCP).............. 88 5.3 Cost analysis of multilevel Cannon algorithm under the HCP model........... 93 5.4 Cost analysis of multilevel CALU under the HCP model................. 95 5.5 Performance predictions.................................. 102 5.5.1 Modeling an Exascale platform with HCP..................... 104 5.5.2 Performance predictions of multilevel CALU................... 105 5.6 Related work.......................................
Details
-
File Typepdf
-
Upload Time-
-
Content LanguagesEnglish
-
Upload UserAnonymous/Not logged-in
-
File Pages181 Page
-
File Size-