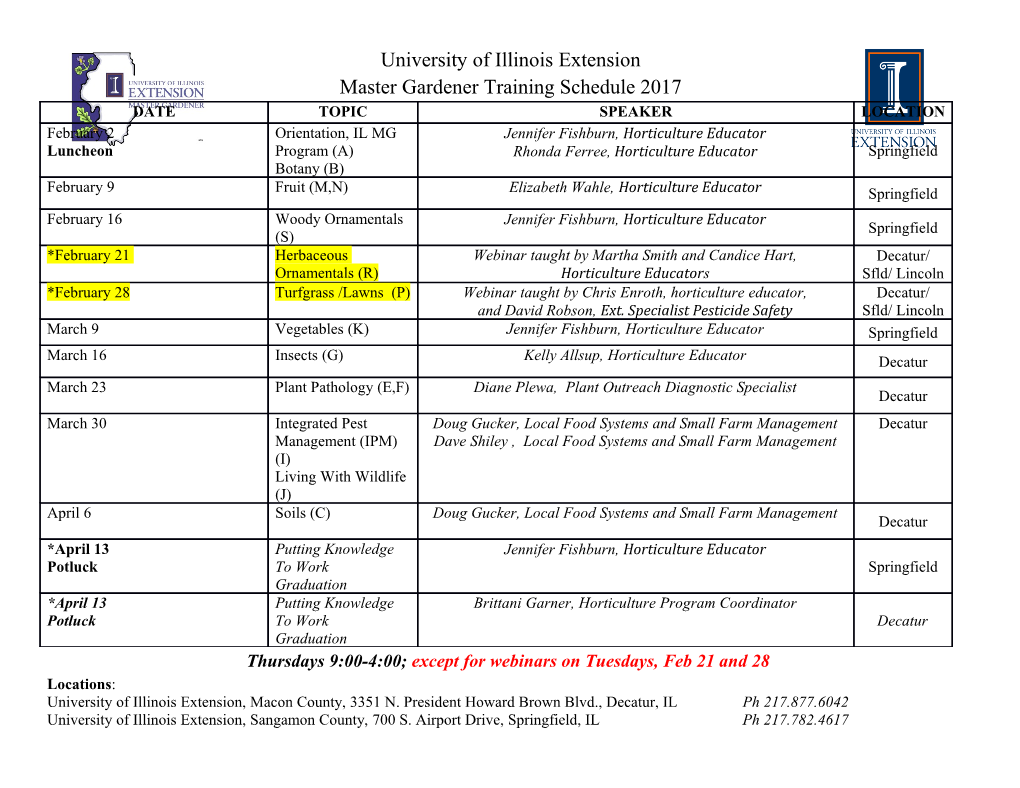
EURASIP Journal on Applied Signal Processing 2001:2, 82–88 © 2001 Hindawi Publishing Corporation The Use of Adaptive Frame for Speech Recognition Sam Kwong Department of Computer Science, City University of Hong Kong, 83 Tat Chee Avenue, Kowloon, Hong Kong Email: [email protected] Qianhua He Department of Electronic Engineering, South China University of Technology, China Email: [email protected] Received 19 January 2001 and in revised form 18 May 2001 We propose an adaptive frame speech analysis scheme through dividing speech signal into stationary and dynamic region. Long frame analysis is used for stationary speech, and short frame analysis for dynamic speech. For computation convenience, the feature vector of short frame is designed to be identical to that of long frame. Two expressions are derived to represent the feature vector of short frames. Word recognition experiments on the TIMIT and NON-TIMIT with discrete Hidden Markov Model (HMM) and continuous density HMM showed that steady performance improvement could be achieved for open set testing. On the TIMIT database, adaptive frame length approach (AFL) reduces the error reduction rates from 4.47% to 11.21% and 4.54% to 9.58% for DHMM and CHMM, respectively. In the NON-TIMIT database, AFL also can reduce the error reduction rates from 1.91% to 11.55% and 2.63% to 9.5% for discrete hidden Markov model (DHMM) and continuous HMM (CHMM), respectively. These results proved the effectiveness of our proposed adaptive frame length feature extraction scheme especially for the open testing. In fact, this is a practical measurement for evaluating the performance of a speech recognition system. Keywords and phrases: speech recognition, speech coding, adaptive frame, signal analysis. 1. INTRODUCTION measured over long periods of time (on the order of 0.2 sec- onds or more). For reducing the discontinuities associated To date, the most successful speech recognition systems with windowing, pitch synchronously speech processing may mainly use Hidden Markov Model (HMM) for acoustic mod- be utilized [9, 10]. This technique is mainly used for synthesis eling. HMM in fact dominates the continuous speech recog- of speech and rate-reduction speech coding. nition field [1]. In order to improve the performance of It is well known that the choice of analysis frame length speech recognition, a great deal of efforts had been made is fundamental to any transform-based audio coding meth- to study the training approaches for HMMs [2, 3, 4], or ods. A long transform length is most suitable for input sig- variations of the conventional HMM, such as the segment nals whose spectrum remains stationary or varies slowly with HMM [1], and the HMMs with state-conditioned second- time, such as the quasi-steady state voiced regions of speech. order nonstationary [5]. In general,frame-based feature anal- A long transform length provides greater frequency resolu- ysis for speech signals has been accepted as a very successful tion. On the other hand, a shorter transform length, pro- technique. In this method, time speech samples are blocked cessing greater time resolution, is more desirable for signals into frames of N samples, with adjacent frames separated that are changed rapidly in time. Therefore, the time versus by M samples. Then the spectral characteristic coefficients frequency resolution tradeoff should be considered when se- are calculated for each frame via some speech analysis meth- lecting a transform frame length. The traditional approach ods (coding procedure), such as LPC, FFT analysis, Gabor to solve this dilemma is to select a single transform frame expansion [6], or wavelets [7]. N is usually set to be the num- length that provides the best tradeoff of coding quality for ber of samples of 30–45 ms signal and M to be N/3, [8]. both stationary and dynamic signals. For example, the typi- This procedure based on the assumption that speech signal cal value of N and M are 300 and 100 when the sampling rate could be considered as quasi-stationary if speech signal is is 6.67 kHz [8]. It is well known that there are areas where examined over a sufficiently short period of time (between the speech signal is relatively constant and in other areas the 5 and 100 ms). However, this is not true when the signal is signal may change rapidly in time. Therefore, this scheme The use of adaptive frame for speech recognition 83 does not provide good choices for both stationary and dy- feature vectors of quasi-stationary signal are computed with namic signals. However, in practice, this is the situation in normal frames (N samples) and dynamic signal with short extracting spectral features for speech recognition. frames. For practical reasons, the length of short frame is set Another alternative to solve this dilemma is to apply the to be half of a normal frame, that is, a normal frame is di- variable frame rate (VFR) analysis techniques. Those are vided into two short frames. A frame is analyzed in short or well-established methods for data reduction in speech coding normal frame based on whether a transient could be detected and have been used in various speech recognition systems in that particular frame or not. If a transient is found, we [11]. Ponting and Peeling [12] gave the first demonstration call it as transient frame. The transient detection algorithm and showed that VFR could successfully improve the perfor- is presented first, and then two expressions are suggested for mance of speech recognition. Based on a similarity measure the feature vectors of transient frame. between two feature vectors, the VFR algorithm is designed to retain all the input feature vectors when they are changing 2.1. Transient detection rapidly and remove a large number of vectors when they Transients are detected for each normal frame in order to are relatively constant. VFR results in considerable saving decide whether a frame is analyzed in two short frames or not. of computational load by reducing the number of frames The pre-emphasized version of the speech signal is examined processed. Specifically, if D(i, j) is the distance between the for an increase in energy from one subframe time-segment previously selected frame j and the current frame i, and the to the next. Subframes are examined in different time scales. threshold is T , then the rule is to select frame i as the next If a transient is detected in the second half of a speech frame, output frame if D(i, j) ≥ T , [12]. Furthermore, Besacier that frame is then analyzed using short frames. et al. [13] used the frame pruning (a variation of VFR) for Transient detection could be done in three steps: speaker identification, and significant improvement was achieved on the TIMIT and NON-TIMIT databases. An (1) segmentation of the frame into subframes in different evident shortcoming of the VFR is that the discarded feature time scales, vectors have no contribution to the recognition procedure. (2) peak amplitude detection for each subframe segment, For speech signals, some regions are relatively constant (3) threshold comparison. The transient detector will out- (stationary speech) while others may change rapidly in time put a flag to indicate whether this frame is analyzed in (dynamic speech). Under a constraint of fixed (average) short for normal frame. frame rate, a practical approach is to code the dynamic speech (1) Frame segmentation: normal frame of N pre- with higher time resolution by analyzing it with shorter frame, emphasized samples are segmented into a hierarchical tree and code stationary speech in higher frequency resolution by in which level 1 represents two N/2 length frames and level analyzing it with longer frame. 2 is four segments of length N/4. This paper proposes an adaptive frame length selection (2) Peak detection: the sample with the largest magnitude approach (AFL) that can adapt to the frequency/time res- is identified for each segment on every level of the hierarchical olutions of the transform depending upon the spectral and tree. Let P[j][k] be the peaks of kth segment of level j,where temporal characteristics of the signal being processed. During j = 1, 2 is the hierarchical level number, k is the segment the feature extraction process, each speech frame is examined number within level j. at different time scales. If a transient is detected in the sec- (3) Threshold comparison: the relative peak levels of suc- ond half of a speech frame, then this frame will be analyzed cessive segments on each level of the hierarchical tree are with short frame (a normal frame is divided into two short checked. If any of the following inequalities is true, then tran- frame of same length in the experiments). Thus, more ac- sient is found in current frame: curate speech coding can be achieved by using the adaptive frame length method. It is expected that this accurate cod- P[1][2] ∗ T[1]>P[1][1] (1) ing of speech could result in better recognition performance, which are confirmed with the experiments carried out in this or study. This paper is organized as follows. Transient detection P[2][k] ∗ T[2]>P[2][k − 1], k = 3, 4, (2) method and the analysis approaches for transient frame are described in Section 2, which is the major contribution of where T[j]is the pre-defined threshold for level j. Since there this work. In Section 3, experimental results on TIMIT and is no perfect theoretical way for determining a good setting NON-TIMIT databases are presented to demonstrate the ef- for T[j], these thresholds are determined experimentally. We fectiveness of the AFL on recognition performance.
Details
-
File Typepdf
-
Upload Time-
-
Content LanguagesEnglish
-
Upload UserAnonymous/Not logged-in
-
File Pages7 Page
-
File Size-