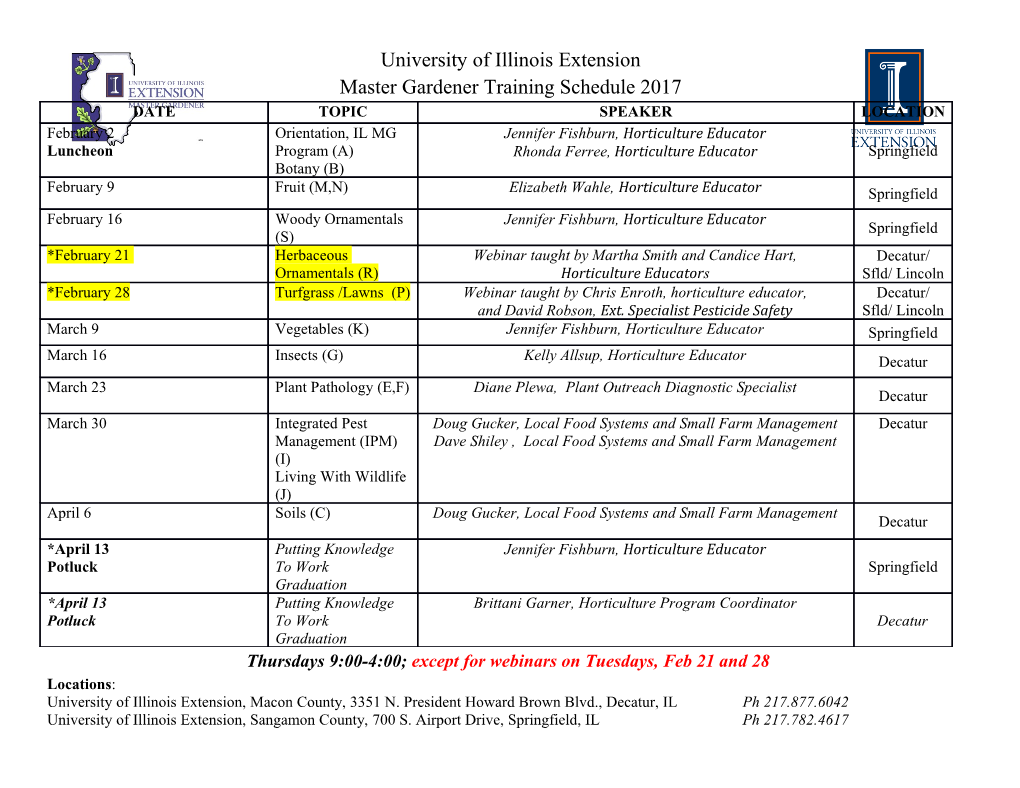
Senior Staff Software Engineer, Google Google I/O Extended Boulder 8-May-2018 #io18extended ...like space :) #spaceishard Spaceflight is unforgiving and complicated. But it’s not a miracle. It can be done #intelligenceishard ● Fundamentals of machine learning are complicated; ML is easily misapplied ● Doing “toy” things with ML is easy. ○ Doing useful things is a lot harder How to get there from here? ● Start small, but real ● Measure. Be rigorous. Make sure you’re helping ● Have a compelling UX ● Launch. Iterate. Improve. ● Never forget the user #io18extended Drive Quick Access Main Idea: Prominently show the user's documents and files they likely want to open right now Benefit 1: Save Users Time ● Quick Access gets users to their files 50% faster… and with less cognitive friction Benefit 2: Enable users to make better business decisions ● Show users relevant documents to their pending business decisions, including documents they may not be aware of. (The right information at the right time.) #io18extended Drive Quick Access: Mobile (Android and iOS) #io18extended What we’ve learned: Quick Access Feature Intelligence works ● Training and using Machine Learning models results in improved metrics beyond a simple “Most Recently Used” across all metrics Quick Access saves users time ● About 50% of opens come from QA, each one saves 50% on “finding time” Starting Point for Future Work ● Quick Access has proven out our machine learning infrastructure for Drive and provides a framework for future intelligence features. #io18extended Quick Access is a Large-Scale Project Clients Web, Android, iOS Drive API Prediction service Experiment Evaluation pipeline Quick Access ML system framework BigQuery Retrieve predictions Compute predictions Manage alternatives Compute accuracy System Components ● TensorFlow, mapreduce Activity service Other inputs ML system ML Model Metrics ● Experiment framework Deep network Bigtable backed ● Google BigQuery ● Servers and deployment ● Load balancing Data extraction Training ● APIs and protocols Flume pipeline Training data Flume pipeline ● Dashboards Collect data Build model ● Statistical evaluation #io18extended Features and Data (a.k.a. “Inputs,” “Predictors,” “Signals”) Features are the signals extracted from data to train models and to make predictions Example feature types 1. Frequency and Recency: Ranks of documents by frequency and recency of access 2. Periodicity: Time of day, time of week an activity was performed on a document Feature engineering: Create useful derived signals; e.g., histograms Post-processing: Minimal post-processing done; some scaling, etc. Feature Data Source: Activity Service, which receives and records events for documents ● E.g., when an item was created, shared, opened, edited, commented on, etc. ● Used during both model training time and model evaluation time #io18extended Model: Deep Neural Network ● Framework: TensorFlow -- Open source ML toolkit ● Training: Conventional Back-Propagation / SGD (asynchronous stochastic gradient descent) ○ Distributed, parallel mapreduce task w/ 200+ workers ● Features: Approximately 20,000 - 40,000 features in use ● Setup: Two-class classification problem ○ Positive training examples ■ Documents the user opened (and their features) ○ Negative training examples ■ Documents the user did not open ● Evaluation: Model evaluated when user visits Drive ● Model output: probability_open from a candidate set of N docs #io18extended Quality Metrics are how we optimize the model 1. Hit rate ○ Measures utility of Quick Access in getting users to their doc 2. Accuracy ○ Measures efficacy of machine learning predictions 3. Click-Through Rate (CTR) ○ Measures general engagement of users with Quick Access #io18extended Production metrics track performance of the service QPS (queries Per second) Latency (milliseconds per query) ● Slow Rollout ● Parallelization of backend calls ● Load Testing ● Increased capacity for reduced ● Platform growth overall load #io18extended Primary Metrics Time-Savings Metrics #io18extended Experiment Framework for Continuous Improvement Question: How do we improve a system in a principled way? Answer: Science (experiments) ● You have an existing system ● You have an idea for how to make it better hypothesis( ) ● How will you know if it improves the system? (Or if it makes it worse?) Approach ● Create an experiment with Experiment Framework ● Rigorously test the hypothesis ● Evaluate outcome, compare with hypothesis. Ship the improvements! Result: Model accuracy, user benefit improves; increased domain understanding #io18extended Improving Model Accuracy: One Idea ● Hypothesis: Actions on documents I own from my boss are more relevant, should be boosted higher ● Method: Introduce new features (signals) and see if we can improve model metrics through an experiment ● Feature Ideas: ○ Basic: ACTOR_WAS_BOSS = {True|False} ○ Extensed: ACTOR_CATEGORY = {Coworker|Report|Manager} ○ Generalized: Assign continuously valued weight to each user ● Run an experiment, test hypothesis, and ship the improvements! #io18extended When it all goes right... When we… ● Gather the correct signals ● Train correct models (apply ML properly) ● Measure the right thing ● Optimize for those correct metrics ● Build out the infrastructure ● Scale the system ● Create a beautiful, usable UX ● Make it all super-fast ● Methodically run experiments to constantly improve quality... The Result: Magic. An intelligence feature that delivers real value to the user and to the business. #io18extended Next Steps Get your learn on with Kaggle… ...then participate in competitions kaggle.com #io17extended ● Hands-on Data Science Education ● Lessons in ML, Data visualization, SQL, R, Deep learning, and more #io18extended ● Kaggle Competitions -- real-world practice! ● Build a model, make a submission ● See your model’s performance scored live on a leaderboard #io18extended Thank you! Next up: Ali Beatty on Apigee Edge Questions (5mins)? #io17extended.
Details
-
File Typepdf
-
Upload Time-
-
Content LanguagesEnglish
-
Upload UserAnonymous/Not logged-in
-
File Pages18 Page
-
File Size-