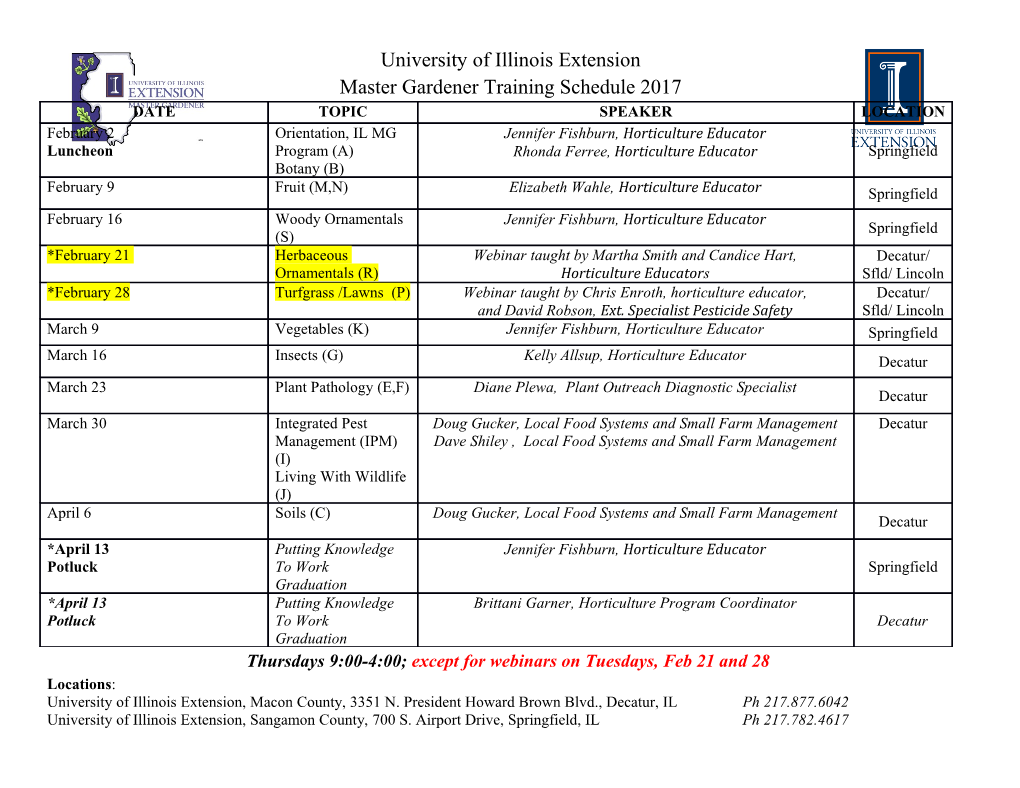
THÈSE DE DOCTORAT DE L’UNIVERSITE DE RENNES 1 COMUE UNIVERSITE BRETAGNE LOIRE Ecole Doctorale N°601 Mathématique et Sciences et Technologies de l’Information et de la Communication Spécialité : Signal, Image, Vision Par « Clémentine SHAO » « Images and models for decision support in aortic dissection surgery » «Images et modèles pour l’aide à la décision clinique de la chirurgie de la dissection aortique» Thèse présentée et soutenue à RENNES , le 16/12/19 Unité de recherche : LTSI, Inserm U1099 Thèse N° : Rapporteurs avant soutenance : Frans Van De Vosse, PR, Eindhoven University of Technology (TU/e), Netherlands Alain Lalande, MCU-PH, Université de Bourgogne Franche-Comté, France Composition du jury : Président : Examinateurs : Alain Lalande, MCU-PH, Université de Bourgogne Franche-Comté, France Nadjia Kachenoura, CR INSERM, Sorbonne Universités, France Frans Van De Vosse, PR, Eindhoven University of Technology (TU/e), Netherlands Jean-Philippe Verhoye, PU-PH, CHU de Rennes, France Dir. de thèse : Pascal Haigron, PR, Université de Rennes 1, France Co-dir. de thèse : Gabriele Dubini, PR, Politecnico di Milano, Italie Invité(s) Michel Rochette, Directeur Technique, Ansys France, France ACKNOWLEDGEMENT Je tiens à remercier I would like to thank. my parents.. J’adresse également toute ma reconnaissance à .... .... i LIST OF ABBREVIATIONS AA Ascending Aorta AD Aortic Dissection BC Boundary Condition BT Brachiocephalic Trunk CFD Computational Fluid Dynamics CT Computed Tomography CVS Cardiovascular System DA Descending Aorta DOE Design Of Experiment DNS Direct Numerical Simulation DSC Dice Similarity Coefficient FL False Lumen FSI Fluid-Structure Interaction HD Hausdorff Distance HED Holisticallynested Edge Detect LCC Left Common Carotid LES Large Eddy Simulation LS Left Subclavian MRI Magnetic Resonance Imaging PC MRI Phase-Contrast Magnetic Resonance Imaging RANS Reunold Averaged Navier-Stokes RBC Red Blood Cell RMS Root Mean Square RNG Renormalization Group ROM Reduced Order Model SAS Scale Adaptative Simulation TL True Lumen WSS Wall Shear Stress iii TABLE OF CONTENTS List of abbreviations iii List of Figures ix List of Tables xvii Résumé en Français xix Introduction 1 1 Clinical context 5 1.1 Cardiovascular system . 5 1.1.1 The heart . 6 1.1.2 Pulmonary and systemic circulations . 8 1.1.3 Aorta anatomy . 11 1.2 Aortic dissection . 12 1.2.1 Pathology, symptoms and causes . 13 1.2.2 Classification of ADs . 14 1.2.3 Treatment . 15 1.3 Conclusion . 16 2 Image segmentation of aortic dissection 19 2.1 Context and difficulties of the segmentation of type B AD . 19 2.2 State of the art . 22 2.3 Semi-automatic method for type B aortic segmentation . 24 2.3.1 Pre-processing . 25 2.3.2 Initialization of the two lumina . 27 2.3.3 Segmentation of the two lumina . 30 2.3.4 Post processing . 32 2.3.5 From segmentation to surface mesh . 33 v TABLE OF CONTENTS 2.3.6 Implementation of the method . 34 2.4 Results . 36 2.4.1 CT Image acquisition . 37 2.4.2 Parameters setup . 37 2.4.3 Segmentation error criteria . 38 2.4.4 Quantitative analysis . 38 2.4.5 Qualitative analysis . 43 2.4.6 Comparison with deep-learning based methods . 45 2.4.7 Comparison of CFD results using different smoothing methods . 48 2.5 Discussion . 51 2.6 Conclusion . 53 3 Patient specific CFD simulation of the aorta 55 3.1 State of the arts of CFD for AD . 55 3.1.1 Fluid modeling . 56 3.1.2 Model of the aorta wall . 58 3.1.3 Boundary conditions . 59 3.1.4 Summary . 60 3.2 0D models of the CVS as BCs for 3D CFD . 62 3.2.1 0D model of the systemic and pulmonary circulation . 63 3.2.2 0D model of the heart . 65 3.2.3 Multiscale coupling . 65 3.2.4 Personalization of the 0D model . 66 3.3 Method . 67 3.3.1 Geometry and meshes . 68 3.3.2 Creation of the hybrid 0D model of the aorta . 69 3.3.3 Initialization of the 0D model of the CVS . 75 3.3.4 Personalization of the 0D model of the CVS . 80 3.3.5 Setup of the 3D patient specific CFD . 83 3.4 Results . 84 3.4.1 Response surface from the static simulations . 84 3.4.2 Optimization processes . 85 3.4.3 3D transient fluid simulations of the AD . 88 3.4.4 Focus on the tear regions of the AD . 97 vi TABLE OF CONTENTS 3.5 Discussion . 97 3.6 Conclusion . 101 4 Dynamic ROM based on CFD 103 4.1 State of the arts . 103 4.2 Method . 105 4.2.1 SVD to obtain 3D dynamic ROM . 105 4.2.2 Dynamic ROM method . 107 4.2.3 Generation of the learning and validation data for the ROMs . 108 4.2.4 Integration of the ROM in the 0D model of the full CVS . 109 4.2.5 Computation of different clinical scenarios . 109 4.3 Results . 111 4.3.1 0D dynamic ROMs . 112 4.3.2 Final coupled 0D models . 115 4.3.3 ROMs computing the pressure at the wall . 119 4.3.4 ROMs computing the WSS . 126 4.3.5 ROMs computing the velocity vectors . 130 4.3.6 Simulation of clinical scenarios for AD . 135 4.4 Discussion . 139 4.5 Conclusion . 141 Conclusion 143 Bibliography 167 vii LIST OF FIGURES 1 Stucture de l’aorte. a) Différentes parties de l’aorte. b) Couches de la paroi de l’aorte. xx 2 Dissection aortique. a) Déchirure de l’intima. b) Classification de Stan- ford. xx 3 Schéma des différentes étapes de la méthodes de segmentation. xxii 4 Schéma des différentes étapes de la méthode de simulation dynamique spécifique patient. xxiv 5 Résultat de la simulation dynamique de l’aorte au pic de la systole. a) Pression à la paroi. b) Contraintes de cisaillement. c) Champs de vi- tesse. xxv 6 Schéma de l’approche basée sur les modèles d’ordre réduit pour le cas de la dissection aortique. xxvii 1.1 Scheme of the cardiovascular system. 6 1.2 Heart anatomy. 7 1.3 Cardiac cycle phases. 8 1.4 Vessels composing the pulmonary and systemic circulations. 9 1.5 Structure of the wall of the veins and arteries. 10 1.6 Structure of blood vessels. The values for medium arteries, arterioles, venules, and veins are merely illustrative because the dimensions can range widely. The drawings of the vessels are not to scale. 11 1.7 Anatomy of the aorta. a) Structure of the aorta. b) Branches of the aorta. 12 1.8 AD with a zoom on the entry tear. 13 1.9 Stanford and De Bakey AD classifications. 15 1.10 Type B dissection and endovascular devices (Stent-graft and delivery system) [105] . 16 ix LIST OF FIGURES 2.1 CT slices of ADs with various shapes. a) Typical case. b) Difference of contrast between the two lumina. c) Noise giving the flap blurry. d) True lumen completely surrounded by the false lumen. e) Moving flap. f) Partially thrombosed false lumen. g) Aneurysm in the aortic arch. h) Aneurysm and separated lumina. i) Stent in one lumen. 21 2.2 Image processing pipeline of the segmentation method. 25 2.3 Preprocessing steps of the image. a) Ct-scan image. b) Smoothed image. c) Gradient image. d) Sigmoid image. 27 2.4 Pathlines of the two lumina : initialization points and different parts of TL. 28 2.5 Segmentation steps of the two lumina. a) Result from the fast-marching method for the true lumen. b) Result from the active contour geodesic method for the true lumen. c) Result from the fast-marching method for the false lumen. d) Result from the active contour geodesic method for the false lumen. 32 2.6 Steps of the Laplacian smoothing. a) Original points. b) Center of gravity calculation G. c) Moving the initial point in the direction of G by a fraction λ of the distance. d) New point position. 33 2.7 Screenshot of the interface of 3D Slicer. 35 2.8 Screenshot of the graphic user interface of the developed segmentation module. 35 2.9 Screenshot of the final segmentation results. 36 2.10 Example of the uncertainty of the manual segmentation on a CT slice with noise. a) CT scan slice. b) First example of segmentation. c) Second example of segmentation. 39 2.11 Distance error for patient 2. a) Absolute distance between the manually segmented dissection and the semi-automatically extracted one. b) Seg- mentation results on the slice with the highest distances. c) Correspon- ding CT slice. 41 2.12 Distance error of patient 4.1. a) Absolute distance between the manually segmented dissection and the semi-automatically extracted one. b) Seg- mentation results on slice 1. c) CT slice of slice 1. d) Segmentation re- sults on slice 2. e) CT slice of slice 2. 42 x LIST OF FIGURES 2.13 Distance error of patient 7.1. a) Absolute distance between the manually segmented dissection and the semi-automatically extracted one. b) Seg- mentation results on slice 1. c) CT slice of slice 1. d) Segmentation re- sults on slice 2. e) CT slice of slice 2. 43 2.14 CT slice of the case where the true lumen was too thin and the segmen- tation failed. 44 2.15 Segmentation on various geometries of the lumina. a) Typical AD slice b) Case where three lumina appear on the same slice. c) Case with a partially thrombosed FL. d) Case with an aneurysm in the aortic arch. e) Case with an aneurysm and a stent.
Details
-
File Typepdf
-
Upload Time-
-
Content LanguagesEnglish
-
Upload UserAnonymous/Not logged-in
-
File Pages201 Page
-
File Size-