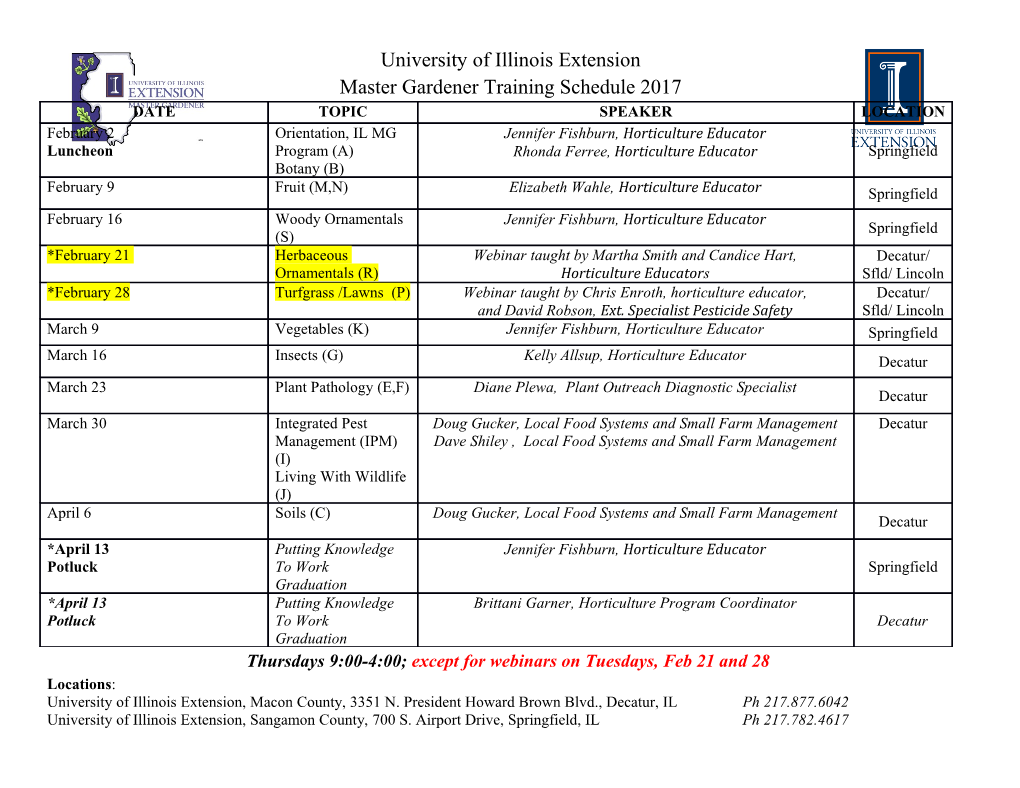
INTERSPEECH 2019 September 15–19, 2019, Graz, Austria Gender de-biasing in speech emotion recognition Cristina Gorrostieta, Reza Lotfian, Kye Taylor, Richard Brutti, John Kane Cogito Corporation fcgorrostieta,rlotfian,ktaylor,rbrutti,jkaneg @cogitocorp.com Abstract vertisement delivery [9], object classification [10] and image search results for occupations [11] . Machine learning can unintentionally encode and amplify neg- There are several factors which can contribute to produc- ative bias and stereotypes present in humans, be they conscious ing negative bias in machine learning models. One major cause or unconscious. This has led to high-profile cases where ma- is incomplete or skewed training data. If certain demographic chine learning systems have been found to exhibit bias towards categories are missing from the training data, models developed gender, race, and ethnicity, among other demographic cate- on this can fail to generalise when applied to new data contain- gories. Negative bias can be encoded in these algorithms based ing those missing categories. The majority of models deployed on: the representation of different population categories in the in modern technology applications are based on supervised ma- training data; bias arising from manual human labeling of these chine learning and much of the labeled data comes from people. data; as well as modeling types and optimisation approaches. In Labels can include movie reviews, hotel ratings, image descrip- this paper we assess the effect of gender bias in speech emotion tions, audio transcriptions, and perceptual ratings of emotion. recognition and find that emotional activation model accuracy is As people are inherently biased, and because models are an es- consistently lower for female compared to male audio samples. timate of people’s impressions, it follows that this bias will be Further, we demonstrate that a fairer and more consistent model implicitly encoded into these algorithms. As a result, there is accuracy can be achieved by applying a simple de-biasing train- the real risk that these systems can inadvertently perpetuate or ing technique. even amplify bias contained in the label data [12]. Given a large, appropriately sampled dataset and labels with 1. Introduction a minimal degree of bias, there is still the risk of introducing In recent times, machine learning via deep neural networks has bias from the features and modeling techniques used. This prob- led to rapid improvements in computer vision, language tech- lem is not new and in fact has been present in the area of speech nologies, and medical applications, among others. In tandem technology for many decades. Until recently, speech synthesis with these advances has come an increased focus on bias and and speech recognition favoured lower pitch voices, typically ethics in machine learning [1, 2]. In machine learning, discrim- present in adult males. As a result, speech recognisers pro- ination itself is not always negative: in fact, the objective of duced higher word-error rate scores for children and adult fe- training these algorithms is to find patterns and characteristics males [13]. One reason for this is fact that early speech analysis in the data that allow you to effectively separate the categories and measurement techniques were developed mainly with low- you care about (e.g., recognising classes of emotion). However, pitched male voices in mind. But such acoustic characterisation this should not be at the cost of introducing negative bias. of speech production did not sufficiently generalise to voices There have been some high profile cases of bias including with a higher pitch, where the spacing of the harmonics in the computer vision systems reportedly having a higher error rate audio spectrum is much wider and the assumptions of a linear for recognising dark skinned women [3]. Other research has time-invariant vocal system become seriously undermined. found that Black and Hispanic borrowers were treated far less Few studies have assessed the affect of gender bias in favourably than white borrowers by new credit worthiness tech- speech with respect to emotion. [14] assessed the perception nology [4, 5]. of vocalisations and did not find any effects of negative bias to- Language technology has also come under considerable wards gender. However, the stimuli consisted of acted speech scrutiny for gender bias. For many modern natural language samples and so the findings may not generalise to natural con- processing models, words are converted to numerical represen- versational speech. A later study [15] did in fact observe differ- tations known as word-embeddings. The embeddings encode ent identification accuracy rates in the perception of emotion for semantic information by assessing the context (or neighbour- male compared with female speakers. Some recent research has hood) in which a word occurs. These representations result approached the problem of mitigating negative bias in machine in words with similar semantics being close to each other in learning generally, with [16] proposing an adversarial training a mathematical sense and enable assessment of the relation- technique to attenuate different formulations of bias. A related ships between them. For instance, you can easily use word- paper [17] demonstrated how autoencoder models can be used embeddings to predict the word “queen” from an input “man is to learn representations free of “nuisance variables” (e.g., gen- to king, as woman is to?”. However, a 2016 NeurIPS paper [6] der) by incorporating orthogonal components to the network. found that such encodings led to biased and sexist output. For Several publications have investigated gender de-biasing in nat- example, the input “man is to computer programmer as woman ural language processing (see for instance [10, 18]). is to?” resulted in the output “home-maker”. Recent studies Although some previous research has investigated model- show that word-embeddings capture common stereotypes be- ing emotion and gender separately [19, 20], to the best of our cause these implicit biases typically exist in large text databases knowledge the present paper is the first to investigate gender [7, 8]. Discrimination has also been reported in systems ad- de-biasing directly in speech emotion recognition, and its main Copyright © 2019 ISCA 2823 http://dx.doi.org/10.21437/Interspeech.2019-1708 contributions are as follows: variable (i.e. any category in the population which is at risk for negative bias). 1. We assess the effect of gender bias in a standard speech The approach involves jointly training two models: a re- emotion recognition model on a large, naturalistic and gression predictor and an adversary. The adversary is a low- well-known dataset complexity model which takes the continuous scalar output of 2. We evaluate the efficacy of two de-biasing training tech- the predictor and the binary label variable as inputs and is niques to mitigate the effect of gender bias trained to optimally classify the binary protected variable. In this study we need to binarise the continuous emotional activa- 3. We highlight challenges associated with training with tion label in order to be compatible with this adversarial tech- de-biasing nique for achieving “Equality of odds”. More formally, the ad- versary can be written as: 2. De-biasing emotion recognition models 2.1. Defining fairness z^ = w2 s; sybin; s(1 − ybin) + b s = σ(1 + jcj)^y (2) Before experimenting with techniques to mitigate the effects of where y^ is the regression predictor output prior to any trans- unwanted bias, it is important to start by quantifying fairness. formation, c and b learnable scalars, w2 a learnable vector, σ() We adopt the definitions of fairness with respect to machine indicates the sigmoid activation function, and ybin is the bina- learning models outlined in [5] and [21]. The definitions we rised activation label: use are described below. We use the term “protected variable” ( to refer to some categorisation of the population (e.g., age, gen- 0; if y ≤ θ der, demographics, ethnicity etc.). ybin = (3) 1; “Demographic parity” requires that the prediction of a otherwise model be independent of the protected variable (e.g., gender). with θ being a constant threshold (set in this study to 3.5, which In [5] the authors give the example of a loan application and is the centre of the target variable’s range). The adversary model state that for demographic parity to exist in this case the deci- is trained to minimise the loss, LA. sion on whether or not to grant a loan should have no correlation The regression predictor model is trained to predict y given with being a member of certain protected variable class (e.g., an input feature matrix, x. The loss term associated with pre- being of some specific ethnicity). [5], however, highlights ma- dicting the primary emotional activation target, y, is LP . The jor flaws with this particular definition of fairness. Taking again model is trained by optimizing its prediction of y while simulta- the loan example. forcing demographic parity can result in ap- neously being penalised as the adversary gets better at predict- plications being inappropriately accepted for certain classes of ing the protected variable. The key idea here is to avoid trans- the protected variable. ferring any information which is useful for predicting the pro- Another definition of fairness is “Equality of odds”. In or- tected variable beyond what is already provided in the binarised der for this definition to hold, a model prediction (for example emotional activation ground truth. Hence, the loss function is loan application acceptance) should have the same true positive a weighted combination of LP and LA and the loss function is rate, with respect to the ground truth, for all elements of the pro- updated with the following gradient: tected variable. So if the protected variable is gender, and we make the assumption that gender is strictly binary (which admit- rW LP − α0rW LA (4) tedly may not be an entirely valid assumption), the model true where W is the set of model predictor weights and α0 a is hy- positive rate for males is the same as that for females.
Details
-
File Typepdf
-
Upload Time-
-
Content LanguagesEnglish
-
Upload UserAnonymous/Not logged-in
-
File Pages5 Page
-
File Size-