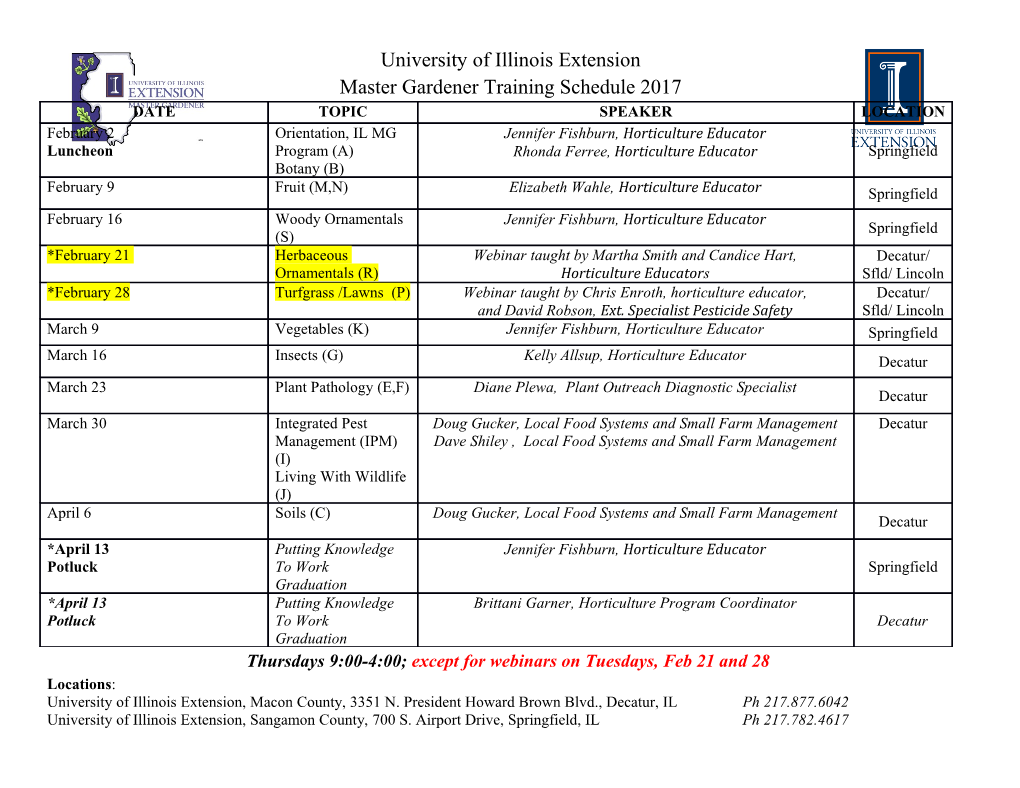
Proceedings of the 5th NA International Conference on Industrial Engineering and Operations Management Detroit, Michigan, USA, August 10 - 14, 2020 Ratio Estimator for Population Variations Using Additional Information on Simple Random Sampling Haposan Sirait, Stepi Karolin Mathematics Study Program, Faculty of Mathematics and Natural Sciences, Universitas Riau, Pekanbaru, Indonesia. [email protected] Sukono Department of Mathematics, Faculty of Mathematics and Natural Sciences, Universitas Padjadjaran, Bandung, Indonesia. [email protected] Kalfin Doctor Program of Mathematics, Faculty of Mathematics and Natural Sciences, Universitas Padjadjaran, Indonesia [email protected] Abdul Talib Bon Department of Production and Operations, Universiti Tun Hussein Onn Malaysia (UTHM) Parit Raja 86400, Johor, Malaysia [email protected] Abstract Variance is a parameter that is often estimated in a research, because variance can give a picture of the degree of homogeneity of a population. To estimate the variance for rare and population has been the main problem in survey sampling Variance obtained based on simple random sampling, there further modified by utilizing additional characters that are assumed to be related to the character under study. This article discusses the population mean estimator by utilizing additional character variances, and then the modified estimator will be compared to the mean square error to obtain an efficient estimator Keywords: Simple random sampling, Mena square error, efficient estimator 1. Introduction In manufacturing industries and pharmaceutical laboratories sometimes researchers are interested in the variation of their products. To measure the variations within the values of study variable y, the problem of estimating the population variance of of study variable Y received a considerable attention of the statistician in survey 2 sampling including Isaki (1983), Singh and Singh (2001, 2003), Jhajj et al. (2005), Kadliar and Cingi (2006), Singh et al. (2008), Grover (2010), Singh et al. (2011) and Singh and Solanki (2012) have suggested improved estimator for estimation of . 2 Simple random sampling is a method for taking n units of population of size N, where each element has the same opportunity to be taken as a sample member. Sampling can be done with a refund (with replacement) or without refund (without replacement). Cochran (1977) states that in simple random sampling without returning, the number of samples to be formed is . Let us consider a finite population = ( , , ,…, ) having N units and let Yand X are the study and auxiliary variable with means X and Y respectively. Let us suppose that a sample of size n is drawn from the 1 2 3 © IEOM Society International 2512 Proceedings of the 5th NA International Conference on Industrial Engineering and Operations Management Detroit, Michigan, USA, August 10 - 14, 2020 population using simple random sampling without replacement (SRSWOR) method. Let For a simple random sample 1 = ( ) , 1 2 2 is an unbiased estimate of � − � − =1 1 = ( ) , 1 2 2 � − � − =1 In other words, The usual sample variance estimator of the population variance was defined as: = 2 2 ̂ The variance of the usual unbiased estimator is given by : � = ( 1) 2 − 4 40 2 where = ( ) �̂ � 20 − 1 Ratio-type estimators take advantage of the correlation between the auxiliary variable, , Z and the study variable, −1 ∑=1� − ⃐�� − ⃐ . When information is available on the auxiliary variable that is positively correlated with the study variable, the ratio estimator is a suitable estimator to estimate the population variance. For ratio estimators in sampling theory, population information of the auxiliary variable, such as the variansi , is often used to increase the efficiency of the estimation for a population variance. Abid et al.(2016) suggested a number of new ratio estimators that were modified in simple random samples Yadav et al.(2013) proposed an estimator for variance with one additional variable, According to Perri (2007), additional variables are usually used in sample survey practices to get better designs and to achieve higher accuracy in estimating population parameters. Ismail et al.(2018) modified the estimator proposed by Isaki (1983) using two additional variables. Furthermore, it is shown that the new ratio is biased so that a square error (MSE) comparison is performed. Getting smaller. The more MSE obtained the more efficient the estimator. This method can be used to increase accuracy in searching for data security. The author is interested in reviewing a new method contained in the article Ismail et al.(2018). The existing ratio estimator for estimating the population variance Y , of the study variable Y is defined as : = 2 2 2 2 Bias and Mean square error of ratio type variancê estimator was : 1 ( ) 1 ( 1) and 2 2 �̂ � ≈ ��2 − � − 22 − � 1 ( ) 1 2( 1) + ( ) 1 2 4 Where = , ( )=� ̂ � ≈ ��2 − � − 22 − �2 − �� 40 � � 2 2 2 2 02 20 02 2. Proposed Modified Ratio Estimators Estimators for the population variance in the simple random sampling using some known auxiliary information on variance . They showed that their suggested estimators are more efficient than traditional ratio estimator in the estimation of the population variance. Ismail et al.(2018) estimators are given as : = + + (1 ) + . 2 2 2 2 2 and ̂1 � � �� 2� − − � 2� � 2 � � − = + + (1 ) 2 2 2 2 ̂2 � � �� 2� − � 2� � − © IEOM Society International 2513 Proceedings of the 5th NA International Conference on Industrial Engineering and Operations Management Detroit, Michigan, USA, August 10 - 14, 2020 Where = + , = ( + ) , = + , = ( + ) . Bias and Mean Square Error of Ratio Type Variance Estimator In order to drive bias of ratio type variance estimator by using 2, = , = ( ) ̂1 = ( ) was written as: 2 −2 2 2 −2 2 2 ̂1 0 1 −2 2 2 (1 + ) + � − � − 2 = ( (1 + ) + ) − ( + )2 2 − 2 1 1 0 2 ̂ � � � (1 + ) + +(1 ) ( + )2 2 1 2 − − � � (1 + ) + + . ( + )2 2 − 2 By suppose, /( + ) = and /( + ) = obtained � 2 � � − 1 2 = ( + + )( (1 + ) + (1 ) 2 2 − ̂1 0 1 1 (1 + ) + (1 + ) . − − By suppose ( ) = , let. − 11 221 � − 1 1 ( ) = , (1 + ) 1 ( ) = 1 1 , (1 + ) Furthermore, using the MacLaurin series approximation is obtained ( ) = (0) + (0) + (0) + + (0), 2!2 ! 1 ′ ( + 1) ′′ = 1 + ( ) ⋯ (0). (1 + ) 2 ! 2 11 Then, the same way is done for 1/(1 + 1 1 ) , obtained1 1 1 1 − − ⋯ − 1 −( + 1) = 1 + 1 1 ( ) (0). (1 + ) 2 ! 2 11 and for 1/(1 + ) obtained− 1 1 1 1 1 1 − − ⋯ − 1 ( + 1) 1 1 = 1 + ( ) (0). (1 + ) 2 ! 2 22 assumption | | < 1 and | | < 1, so2 that2 − 22 − ⋯ − 22 ( + 1) 1 1 ( 2 +2 )(1 + ) 1 ( ) 2 2 2 �̂1� ≈ � 00 � −( + 1)1 � 1 − 11 � +(1 ) ( ) 2 2 − − �( 1+1 1−) 11 � ( ) . 2 2 −2 � 2 − 22 � − � Apply expectation on both side of ,so that , ( + 1) ̂1 ( + )(1 + ) 1 ( ) 2 2 2 �̂1� ≈ � 00 � − 1 � 1 − 11 � ( + 1) +(1 ) ( ) 2 2 − − �11 − 11 � © IEOM Society International 2514 Proceedings of the 5th NA International Conference on Industrial Engineering and Operations Management Detroit, Michigan, USA, August 10 - 14, 2020 ( + 1) ( ) 2 2 or it can be written in form, −2 � 2 − 22 � − � = ( + )(1 + ) (1 + ) + (1 ) 2 (1 + ) ) − ̂1 0 0 1 1 � � � − 1 1 and using ( ) = 0, ( ) = 0 ,E( ) = 0 we obtained, − 0 1 2 ( ( ) +(1 ) 2 ′ 2 2 ̂1 2 1 � � ≈ + −( )( + (1 − ) − ) ( 2 )) ′ 2 ′ 2 1 1 Finally, − − − ( ( ) +(1 ) ′ 2 2 ̂1 2 1 � � + ≈ ( )( + (1− − ) ) ( 2 )) In order to drive mean square error of , ′ 2 ′ 2 − − 1 1 − ̂1 = . 2 2 �̂1� �̂1 − � The ratio estimator is , expressed as a function ( , , ) and the estimated parameter is 2 2 2 2 ̂1 ( , , ), so that : 2 2 2 ( , , ) = , , , , . 2 2 2 2 2 2 2 2 2 2 by approximating to ( , �, ) with the� Taylor �� series and � ignoring − � degrees �� greater than one, obtained : 2 2 2 = = ( , , ) , , = , , 2 = 2 + ( ) 2 = 2 2 2 2 2 2 2 2 2 2 2 = 2 2 2 2 = 2 � � � � � � − 2 � 2 2 2 2 = ( , , ) +( ) 2 = 2 2 2 2 2 2 2 = 2 2 − �2 2 = , , +( ) 2 = 2 2. 2 2 2 2 2 � � 2 = 2 2 − �2 2� Equivalent to = ( , , ) , , = ( , , ) + ( ) 2 = 2 2 2 2 2 2 2 2 2 2 2 2 2 = 2 � � � − 2 � 2 2 = ( , , ) +( ) 2 = 2 2 2 2 2 2 2 = 2 − 2 � 2 2 © IEOM Society International 2515 Proceedings of the 5th NA International Conference on Industrial Engineering and Operations Management Detroit, Michigan, USA, August 10 - 14, 2020 = , , +( ) 2 = 2 2 2 2 2 2 2 � � 2 = 2 2 − �2 2� Thus. = ( , , ) 2 2 ( , , ) ( , , ) = ( ) 2 2 2 = 2 2 2 2 2 2 2 2 2 2 = 2 � − � � − 2 � 2 2 = ( , , ) +( ) 2 = 2 2 2 2 2 2 2 = 2 2 − �2 2 = , , +( ) 2 = 2 2 2 2 2 2 2 � � 2 = 2 2 − �2 2� Apply expectation on both side, so that, = , , ( , , ) = 2 = 2 2 ( ) 2 2 2 2 2 2 � � 2 = 2 2 2 2 � � �� 2 � � − � 2 2 = , , + 2 = 2 2 ( ) 2 2 2 � � 2 = 2 2 2 2 �� 2 � � − � 2 2 = , , + 2 = 2 2 ( ) 2 2 2 � � 2 = 2 2 2 2 �� 2 � � − � 2 2 = , , +2 2 = 2 2 2 2 � � 2 = 2 2 �� �2 2� = , , 2 = 2 ( ) 2 2 2 � � 2 = 2 2 2 2 � �2 2� � − = , , ( )) + 2 2 = 2 2 2 2 2 2 � � 2 = 2 2 − � �� �2 2� = , , 2 = 2 (( ) 2 2 2 � � 2 = 2 2 2 � 2 � � − 2 2 © IEOM Society International 2516 Proceedings of the 5th NA International Conference on Industrial Engineering and Operations Management Detroit, Michigan,
Details
-
File Typepdf
-
Upload Time-
-
Content LanguagesEnglish
-
Upload UserAnonymous/Not logged-in
-
File Pages10 Page
-
File Size-