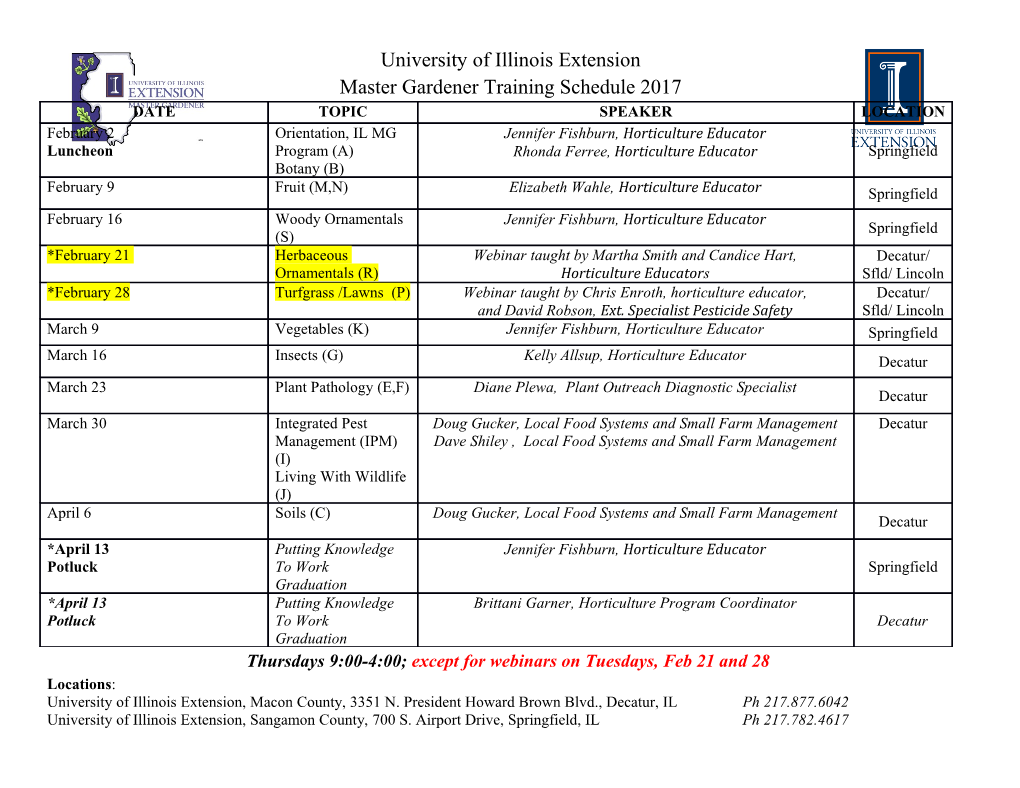
Marco António Morais Veloso ANALYSIS OF TAXI DATA FOR UNDERSTANDING URBAN DYNAMICS Doctor of Philosophy Thesis in Sciences and Information Technologies, supervised by Professor Carlos Lisboa Bento and Professor Santi Phithakkitnukoon, and submitted to the Department of Informatics Engineering of the Faculty of Sciences and Technology of the University of Coimbra September 2016 ii Marco António Morais Veloso ANALYSIS OF TAXI DATA FOR UNDERSTANDING URBAN DYNAMICS PhD Thesis submitted to fulfill the requirements of the Doctoral program in Sciences and Information Technologies Department of Informatics Engineering, Faculty of Sciences and Technology, University of Coimbra Coimbra, September 2016 iii iv Supervisors Professor Carlos Lisboa Bento Associate Professor with Aggregation Department of Informatics Engineering Faculty of Sciences and Technology, University of Coimbra, Portugal Professor Santi Phithakkitnukoon Associate Professor Department of Computer Engineering Chiang Mai University, Thailand v vi Acknowledgments This study is the result of a long and difficult journey spanning several years, and was not possible without the valuable contribution of various people and institutions, which I would like to thank. Firstly, I would like to express my gratitude to my supervisors: Prof. Carlos Bento and Prof. Santi Phithakkitnukoon. Their guidance, expertise, and motivation were fundamental throughout the entire project. They have spare considerable amounts of their personal time to discuss and review my work. They provided solutions and guidance when they were most needed, from setting the initial goals, all the way to the final validation. And above all, they have believed in me and my work, and have been at my side throughout this entire academic path. No contribution or finding produced from this study was possible without the richness of the data set and the knowledge it possesses. Therefore, data providers are a key player in this study – as they are on every research. Besides collecting and providing data, data providers were available to share their knowledge and assist during the initial interpretation of the data sets. I would like to thank all the data providers that made this study possible: Geotaxi, TMN (currently rebranded as MEO), APA (Agência Portuguesa do Ambiente), CCDR-LVT (Comissão de Coordenação e Desenvolvimento Regional de Lisboa e Vale do Tejo), INE (Statistics Portugal), Lisbon Municipality, Sapo Maps, and Weather Undergroud. The Doctoral Program was conducted concurrently with a full-time job. My sponsoring institution’s comprehension and flexibility was essential, allowing me the space to conciliate my research with my professional obligations. Thus, I would like to thank the management and my colleagues at the College of Management and Technology of Oliveira do Hospital and the Polytechnic Institute of Coimbra. In research, the support of a group to discuss and share our approaches and results is essential. They help us validate our work, alert us to any obstacles unforeseen by us, and sometimes identify new paths or courses of action. Also relevant is the companionship that emerges from this community, composed by empathetic fellows sharing similar experiences. A double thank you to all my lab vii colleagues at the Ambient Intelligence Laboratory (AmILab) of Centre for Informatics and Systems of the University of Coimbra (CISUC) for all the experiences we have shared and for their time. Friendship is a major pillar in one’s life. Those who know us best are able to understand us without a word being said and help us without any help being asked. We share life experiences, good and bad, helping each other in difficult times. Thank you to all my friends, to whom I owe a debt of gratitude. But to a few of them, who rose above all others, I give special thanks: Nuno Gil, Ricardo, and Carla. They have been an important support system during this academic step. Our frequent meetings and encounters were both cathartic and a source of motivation. A special thanks to you three. Finally and most importantly, family. Just like true friends, they are fundamental in one’s life - an everlasting source of motivation. They are with us before we have started a new path, and they will stick with us long after it is concluded, regardless of the results. Most of all, they support us during difficult times and share our joys during good times. A very special thanks to my mother, father, brother Luis, sister Ana, and my life partner Sofia. Thank you everyone. This study is just as much the result of my work as it is of yours. viii Abstract The growth of urban areas poses both challenges and opportunities. Challenges due to the increase in demand for resources and services needed. However, it also allows the opportunity for the development of new services and, collectively, urban areas can produce data to help better understand urban mobility. The taxi can be perceived as a probe for traffic conditions. Additionally, its flexibility and ubiquity can be used to retrieve large data sets of information, essential for studying urban mobility. In this study we explore a data set of taxi-GPS traces, collected in Lisbon, Portugal, to understand to what extent can taxi data represent urban mobility. More specifically, in this study we aimed to answer three research questions: (A) Is it possible to develop a model to estimate the taxi demand throughout the city? (B) Are urban data sources correlated among them? More specifically, is taxi activity correlated with mobile phone activity, two of the major urban data sources? (C) Can taxi data be used as a probe to infer the concentrations of exhaust gases in urban areas? To aid the analysis, additional data sets were collected for the same spatiotemporal period, regarding mobile phone activity, information on atmospheric pollutants and meteorological conditions. In order to develop a model to estimate taxi demand, an exploratory analysis was performed. The study was able to visualize the spatiotemporal variation, identifying the main pick-up and drop-off locations and busy hours, and observe that trip distance and duration follow Gamma and Exponential distributions. The study was also able to identify the link between pick-up and drop-off locations, observing strong links between public transportation hubs. Additionally, an analysis of taxi driver behavior during downtime was performed. The analysis of taxi-GPS from top drivers have shown specific strategies used to maximize their profit. Either by waiting for passengers in locations related with main public transportation hubs, during specific hours of the day, or by avoiding traveling great distances to the next pick-up location. The inference analysis explored the possibility of estimating the next pick-up area given the current location (last drop-off), day of the week, hour, weather conditions and area type (characterized by points of interest). The inference engine is based on a naïve Bayesian classifier, achieving 56.3% of accuracy of the training sample. Current ix location turned out to be the main contributor to the algorithm, contrary to weather conditions which is the variable with the least weight in the calculation. The investigation of the relationship between taxi and mobile phone activity started by performing an exploratory analysis of the mobile phone call intensity. The study showed a fairly regular pattern, consistent throughout the day and during the entire time series. During data analysis, a significant correlation between the taxi volume and mobile phone call intensity was found, with a coefficient of determination of 0.8047. The strongest correlation was achieved over active hours of the day (8 AM- 10 PM) and active days of the week (weekdays), in areas with medium and high taxi activity. Moreover, mobile phone call intensity had a significant correlation with taxi volume of the previous two hours. Furthermore, we found that this inter-predictability could be modeled with a linear function and varied across different times of the day. To model and estimate the concentration of exhaust gases, taxi activity and meteorological conditions (temperature, wind, humidity, and weather conditions) were considered. The study revealed the daily and seasonal patterns of exhaust gases, how they are correlated with the weather conditions, and how nitrogen dioxide - a marker for atmospheric pollution - is strongly correlated with other exhaust gases. Using a multilayer perceptron, with 15 hidden layers and a sigmoid activation function, we were able to estimate the nitrogen dioxide concentrations, with a coefficient of correlation of 0.7869, showing a relationship between the exhaust gas concentration and other urban variables, especially on traffic stations. The multicollinearity analysis was applied to ensure non-correlated predictor variables and avoid overfitting of the model. This study contributes to a better comprehension of the complex interactions between the diversity of urban data sources. Our findings, to some extent, unveil the relationships between different urban data sources, especially the role of taxi service as a predictor variable for other urban variables. x Resumo O crescimento das áreas urbanas apresenta tanto desafios como oportunidades. Desafios devido à crescente exigência de recursos e serviços necessários. No entanto, também permite oportunidades para o desenvolvimento de novos serviços e, colectivamente, as áreas urbanas podem produzir dados para auxiliar a melhor compreender a mobilidade
Details
-
File Typepdf
-
Upload Time-
-
Content LanguagesEnglish
-
Upload UserAnonymous/Not logged-in
-
File Pages273 Page
-
File Size-