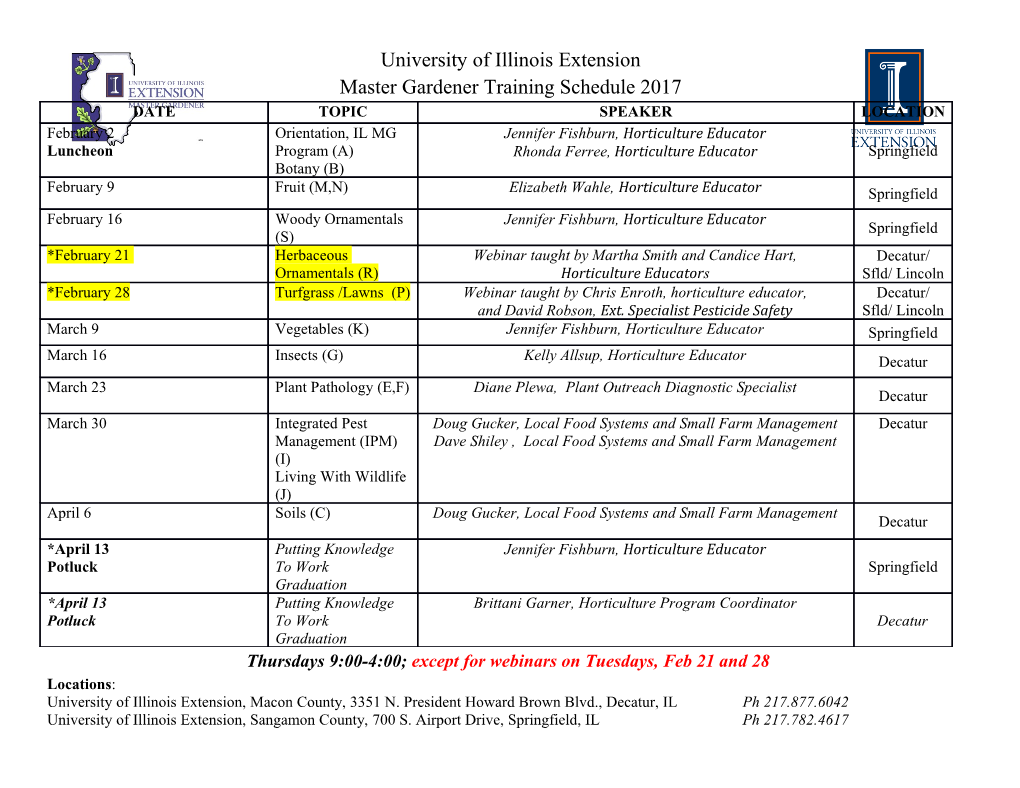
A Bayesian Approach to Adaptive Video Super Resolution Ce Liu Deqing Sun Microsoft Research New England Brown University (a) Input low-res (b) Bicubic up-sampling ×4 (c) Output from our system (d) Original frame Figure 1. Our video super resolution system is able to recover image details after×4 up-sampling. trary, the video may be contaminated with noise of unknown Abstract level, and motion blur and point spread functions can lead Although multi-frame super resolution has been exten- to an unknown blur kernel. sively studied in past decades, super resolving real-world Therefore, a practical super resolution system should si- video sequences still remains challenging. In existing sys- multaneously estimate optical flow [9], noise level [18] and tems, either the motion models are oversimplified, or impor- blur kernel [12] in addition to reconstructing the high-res tant factors such as blur kernel and noise level are assumed frames. As each of these problems has been well studied in to be known. Such models cannot deal with the scene and computer vision, it is natural to combine all these compo- imaging conditions that vary from one sequence to another. nents in a single framework without making oversimplified In this paper, we propose a Bayesian approach to adaptive assumptions. video super resolution via simultaneously estimating under- In this paper, we propose a Bayesian framework for lying motion, blur kernel and noise level while reconstruct- adaptive video super resolution that incorporates high-res ing the original high-res frames. As a result, our system not image reconstruction, optical flow, noise level and blur ker- only produces very promising super resolution results that nel estimation. Using a sparsity prior for the high-res image, outperform the state of the art, but also adapts to a variety flow fields and blur kernel, we show that super resolution of noise levels and blur kernels. Theoretical analysis of the computation is reduced to each component problem when relationship between blur kernel, noise level and frequency- other factors are known, and the MAP inference iterates wise reconstruction rate is also provided, consistent with between optical flow, noise estimation, blur estimation and our experimental results. image reconstruction. As shown in Figure 1 and later exam- ples, our system produces promising results on challenging 1. Introduction real-world sequences despite various noise levels and blur kernels, accurately reconstructing both major structures and Multi-frame super resolution, namely estimating the fine texture details. In-depth experiments demonstrate that high-res frames from a low-res sequence, is one of the fun- our system outperforms the state-of-the-art super resolution damental problems in computer vision and has been exten- systems [1, 23, 25] on challenging real-world sequences. sively studied for decades. The problem becomes particu- We are also interested in theoretical aspects of super res- larly interesting as high-definition devices such as HDTV’s olution, namely to what extent the original high-res infor- dominate the market. There is a great need for converting mation can be recovered under a given condition. Although low-res, low-quality videos into high-res, noise-free videos previous work [3, 15] on the limits of super resolution pro- that can be pleasantly viewed on HDTV’s. vides important insights into the increasing difficulty of re- Although a lot of progress has been made in the past 30 covering the signal as a function of the up-sampling factor, years, super resolving real-world video sequences still re- most of the bounds are obtained for the entire signal with mains an open problem. Most of the previous work assumes frequency perspective ignored. Intuitively, high frequency that the underlying motion has a simple parametric form, components of the original image are much harder to re- and/or that the blur kernel and noise levels are known. But cover as the blur kernel, noise level and/or up-sampling fac- in reality, the motion of objects and cameras can be arbi- tor increases. We use Wiener filtering theory to analyze the 209 Imaging pipeline Inverse inference Motion Observed low-res, noise Low-res, noise-free contaminated sequence Blurred high-res sequence sequence Add noise + Down-sample Filter Noise generator Sub-sampling grid Original high- Blur kernel Noise res sequence parameter Our video super Output estimates resolution algorithm Input Figure 2. Video super resolution diagram. The original high-res video sequence is generated by warping the source frame (enclosed by a red rectangle) both forward and backward with some motion fields. The high-res sequence is then smoothed with a blur kernel, down- sampled and contaminated with noise to generate the observed sequence. Our adaptive video super resolution system not only estimates the high-res sequence, but also the underlying motion (on the lattice of original sequence), blur kernel and noise level. frequency-wise bound, which is further verified through ex- cent advances in optical flow have resulted in much more periments. reliable methods based on sparsity priors e.g. [5]. Accurate motion estimation despite strong noise has inspired Liu and 2. Related Work Freeman [17] to develop a high quality video denoising sys- tem that removes structural noise in real video sequences. In Since the seminal work by Tsai and Huang [26], signif- this paper, we also want to incorporate recent advances in icant progress has been made in super resolution. We refer optical flow for more accurate super resolution. readers to [20] for a comprehensive literature review. Inspired by the successful non-local means method for Early super resolution work focused on dealing with the video denoising, Takeda et al. [25] avoided explicit sub- ill-posed nature of reconstructing a high-res image from a pixel motion estimation and used 3D kernel regression sequence of low-res frames [10]. The lack of constraints is to exploit the spatiotemporal neighboring relationship for often addressed by spatial priors on the high-res image [22]. video up-sampling. However, their method still needs to Hardie et al. [8] jointly estimated the translational motion estimate a pixel-wise motion at regions with large motion. and the high-res image, while Bascle et al. [4] also con- In addition, its data model does not include blur and so its sidered the motion blur using an affine motion model. But output needs to be postprocessed by a deblurring method. these motion models are too simple to reflect the nature of While most methods assume the blur kernel is known, real-world sequences. some work considers estimating the blur kernel under sim- To deal with the complex motion of faces, Baker and ple settings. Nguyen et al. [19] used the generalized Kanade [2] proposed to use optical flow for super reso- cross-correlation method to identify the blur kernel using lution, although in fact a parametric motion model was quadratic formulations. Sroubek et al. [24] estimated the adopted. Fransens et al. [6] proposed a probabilistic for- image and the blur kernel under translational motion mod- mulation and jointly estimated the image, flow field and els by joint MAP estimation. However, their models can Gaussian noise statistics within an EM framework. They barely generalize to real videos due to the oversimplified assumed that the blur kernel was known, and used Gaussian motion models. priors for both images and flow fields. However, Gaussian Significant improvements on blur estimation from real priors tend to over-smooth sharp boundaries in images and images have been made in the blind deconvolution commu- flows. nity. Levin et al. [14] showed that joint MAP estimation of While most of these motion-based super resolution mod- the blur kernel and the original image favors a non-blur ex- els use somewhat standard flow estimation techniques, re- planation, i.e. , a delta blur function and the blurred image. 210 Their analysis assumes no spatial prior on the blur kernel, ∇ while Joshi et al. [11] used a smoothness prior for the blur kernel and obtained reliable estimates. Moreover, Shan et al. [23] applied the recent advances in image deconvolution I to super resolution and obtained promising improvement, ∇ ∇ ∇ ∇ but their method only works on a single frame and does not ∇ w−2 w−1 w1 w2 estimate the noise statistics. F F FF On the theory side, there has been important work on K the limit of super resolution as the up-sampling factor in- ∗ ∗ ∗ ∗ ∗ creases [3, 15]. Their analysis focused on the stability of θ−2 θ−1 θ0 θ1 θ2 linear systems while ignoring the frequency aspects of the S S S S S limit. In fact, many useful tools have been developed in the + R−2 + R−1 + R0 + R1 + R2 signal processing community to analyze the performance of linear systems w.r.t. a particular frequency component. J−2 J−1 J0 J1 J2 Though our super resolution system is nonlinear, we apply these analysis tools to a simplified linear problem and obtain Figure 3. The graphical model of video super resolution. The cir- how the blur kernel and noise level affect the reconstruction cular nodes are variables (vectors), whereas the rectangular nodes at each frequency. are matrices (matrix multiplication). We do not put priors η, λ, ξ, α and β on I, wi, K, and θi for succinctness. 3. A Bayesian Model for Super Resolution {J } Sparsity on derivative filter responses is used to model Given the low-res sequence t , our goal is to re- I w {I } the priors of image , optical flow field i and blur kernel cover the high-res sequence t . Due to computational K issues, we aim at estimating It using adjacent frames 1 Jt−N , ··· ,Jt−1,Jt,Jt+1, ··· ,Jt+N .
Details
-
File Typepdf
-
Upload Time-
-
Content LanguagesEnglish
-
Upload UserAnonymous/Not logged-in
-
File Pages8 Page
-
File Size-