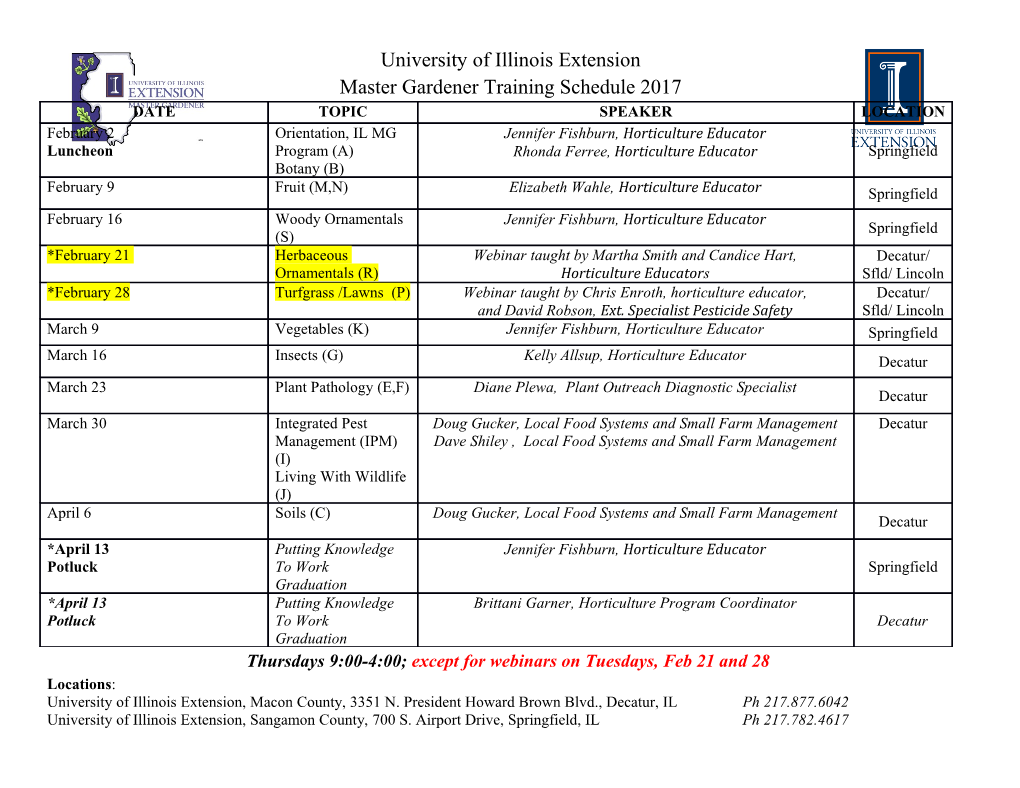
Practical Machine Learning Neural Network Structure Sven Mayer Neuronal Network Structure TensorFlow Syntax for a 2-Layer Model model = tf.keras.Sequential() x = tf.keras.layers.InputLayer((400,), name = "InputLayer") model.add(x) x = tf.keras.layers.Dense(14, name = "HiddenLayer1", activation = 'relu') model.add(x) model.add(tf.keras.layers.Dense(8, name = "HiddenLayer2", activation='relu')) model.add(tf.keras.layers.Dense(2, name = "OutputLayer", activation = 'softmax')) 2 Sven Mayer Layers to Pick from tf.keras.layers.* § AbstractRNNCell § ConvLSTM2D § GlobalAveragePooling2D § MaxPool2D § SpatialDropout2D Activation Convolution1D GlobalAveragePooling3D MaxPool3D § § § § § SpatialDropout3D ActivityRegularization Convolution1DTranspose GlobalAvgPool1D MaxPooling1D § § § § § StackedRNNCells § Add § Convolution2D § GlobalAvgPool2D § MaxPooling2D § Subtract § AdditiveAttention § Convolution2DTranspose § GlobalAvgPool3D § MaxPooling3D § ThresholdedReLU § AlphaDropout § Convolution3D § GlobalMaxPool1D § Maximum § TimeDistributed § Attention § Convolution3DTranspose § GlobalMaxPool2D § Minimum § UpSampling1D § Average § Cropping1D § GlobalMaxPool3D § MultiHeadAttention § UpSampling2D § AveragePooling1D § Cropping2D § GlobalMaxPooling1D § Multiply § AveragePooling2D § Cropping3D § GlobalMaxPooling2D § PReLU § UpSampling3D § AveragePooling3D § Dense § GlobalMaxPooling3D § Permute § Wrapper § AvgPool1D § DenseFeatures § InputLayer § RNN § ZeroPadding1D § AvgPool2D § DepthwiseConv2D § InputSpec § ReLU § ZeroPadding2D AvgPool3D Dot LSTM RepeatVector § § § § § ZeroPadding3D § BatchNormalization § Dropout § LSTMCell § Reshape § Bidirectional § ELU § Lambda § SeparableConv1D § Concatenate § Embedding § Layer § SeparableConv2D § Conv1D § Flatten § LayerNormalization § SeparableConvolution1D § Conv1DTranspose § GRU § LeakyReLU § SeparableConvolution2D § Conv2D § GRUCell § LocallyConnected1D § SimpleRNN § Conv2DTranspose § GaussianDropout § LocallyConnected2D § SimpleRNNCell § Conv3D § GaussianNoise § Masking § Softmax § Conv3DTranspose § GlobalAveragePooling1D § MaxPool1D § SpatialDropout1D 3 Sven Mayer Neuronal Network Structure InputLayer HiddenLayer1 HiddenLayer2 OutputLayer InputLayer Dense Dense Dense ⋮ 2 8 400 14 4 Sven Mayer Neuronal Network Structure InputLayer HiddenLayer1 HiddenLayer2 OutputLayer InputLayer Dense Dense Dense Perceptron ⋮ 2 8 400 14 5 Sven Mayer Neuronal Networks What can be trained? Frank Rosenblatt. "The perceptron: a probabilistic model for information storage and organization in the brain." Psychological review 65, no. 6 (1958): 386. DOI: https://psycnet.apa.org/doi/10.1037/h0042519 6 Sven Mayer What is a Perceptron? Single-Layer Perceptron Bias Input � Output �! �! ∑ �(�) � ⋮ ⋮ Activation function �" �" Weights 7 Sven Mayer What can be trained? Single-Layer Perceptron Input Output � � = � Model 8 Sven Mayer What can be trained? Single-Layer Perceptron Input �+ � � = � ⋮ = � � ' � + � = � �, Model Output 9 Sven Mayer What can be trained? Single-Layer Perceptron Weights Input Bias �+ � � = � ⋮ = � � ' � + � = � �, Model Activation function Output 10 Sven Mayer What can be trained? Single-Layer Perceptron Weights Input Bias �+ � � = � ⋮ = � � ' � + � = � �, Model Activation function Output , = � + �-�- + � -.+ 11 Sven Mayer Activation Function Rectified Linear Unit (ReLU) � �� � > 0 � �� � ' � + � > 0 � � = max 0, � = 2 = 2 0 ��ℎ������ 0 ��ℎ������ 12 Sven Mayer Neuronal Network Structure InputLayer HiddenLayer1 HiddenLayer2 OutputLayer �! �! � ⋮ / ⋮ + � �" �" Perceptron ⋮ 2 8 400 14 13 Sven Mayer Neuronal Network Structure InputLayer HiddenLayer1 HiddenLayer2 OutputLayer Each neuron is � � with a independed � and � ⋮ 2 8 400 14 14 Sven Mayer Combining Perceptions Why is it all about fast matrix multiplication? � � / �! + �! = �! � � / �$ + �$ = �$ 2 8 �!,! �!,$ … �!,% �!,& � � � � ! + ! = ! �$,! �$,$ … �$,% �!,& �$ �$ �$ �!,! ⋯ �!,' �! �! �! � ⋮ ⋱ ⋮ ⋮ + ⋮ = ⋮ �",! ⋯ �",' �" �" �" 15 Sven Mayer Parameter ⋮ 2 8 400 14 § Layers 400, 14, 8, and 2 § => weights: 400 * 14 + 14 * 8 + 8 * 2 = 5,728 § => biases: 14 + 8 + 2 = 24 § => trainable parameter: 5,728 + 24 = 5,752 § Trainable parameters can raise fast § Layers 400, 100, 40, 2 => Parameter: 44,222 § (one model from the walkthough) 16 Sven Mayer Conclusion Neural Network Structure § Perceptron § Weights § Biases § Combining multiple Perceptron § Trainable Parameter § Activation Function (e.g. ReLu) 17 Sven Mayer License This file is licensed under the Creative Commons Attribution-Share Alike 4.0 (CC BY-SA) license: https://creativecommons.org/licenses/by-sa/4.0 Attribution: Sven Mayer Sven Mayer.
Details
-
File Typepdf
-
Upload Time-
-
Content LanguagesEnglish
-
Upload UserAnonymous/Not logged-in
-
File Pages18 Page
-
File Size-