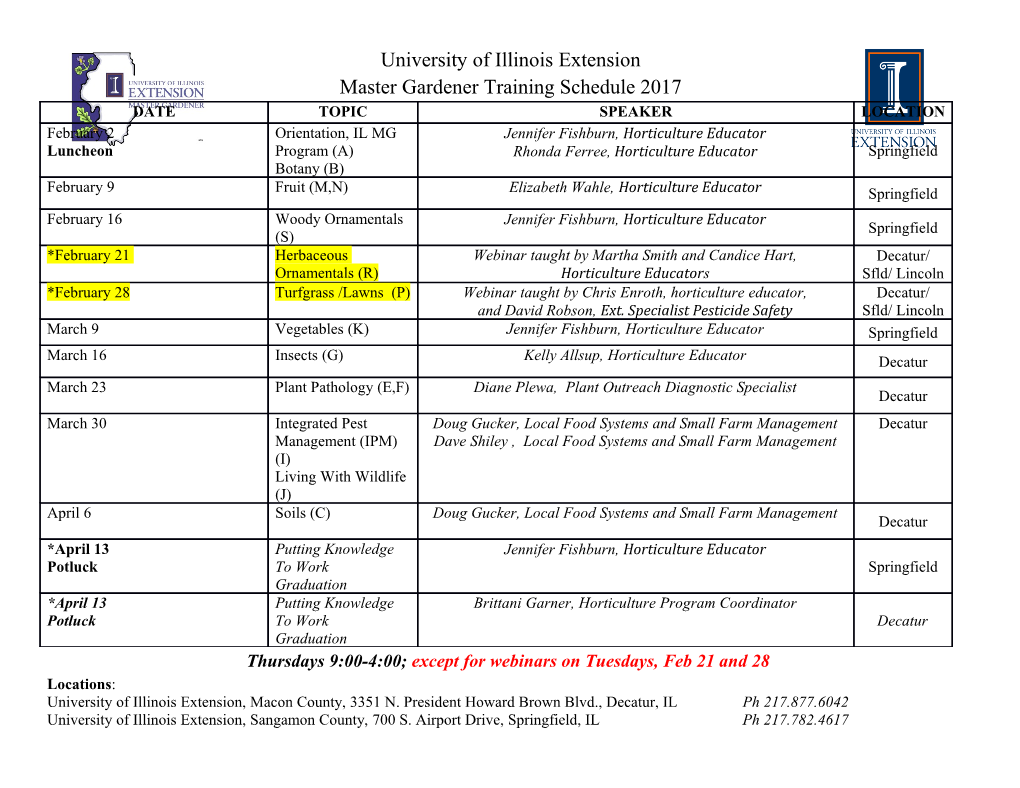
IEEE CSC & ESAS SUPERCONDUCTIVITY NEWS FORUM (global edition), September 2019. Invited presentation 1-QU-I-1 given at ISEC, 28 July-1 August 2019, Riverside, USA. High Coherence Quantum Annealing and Fast, High- Fidelity Flux Qubit Readout Joel Strand, James I. Basham, Jeffrey Grover, Sergey David K. Kim, Alexander Melville, Bethany M. Novikov, Steven Disseler, Zachary Stegen, David G. Niedzielski, Jonilyn L. Yoder Ferguson, Alex Marakov, Robert Hinkey, Anthony J. MIT Lincoln Laboratory Przybysz, Moe Khalil, Kenneth M. Zick Lexington, MA, USA Advanced Technology Laboratory Northrop Grumman Corporation Linthicum, MD, USA Daniel A. Lidar [email protected] University of Southern California Los Angeles, CA, USA Abstract—Quantum annealing is an interesting candidate for approximate optimization algorithm (QAOA) for use with providing a new computing capability for a wide variety of gate-based quantum computers [6]. QA architectures have combinatorial optimization problems. We have implemented much simpler control schemes than gate-based architectures, quantum annealing-capable flux qubits built using MIT Lincoln however, and can reach larger circuit sizes in the near term [7]. Laboratory’s capacitively-shunted flux qubit fabrication process. They may also be more robust to certain forms of decoherence These qubits take advantage of lower persistent currents to [8]. achieve lower noise sensitivity and increase quantum coherence. Qubits with persistent currents in the nA range present unique challenges for readout, and previous methods using rf-SQUID II. QUBIT COHERENCE tunable resonators were too slow for annealing applications. We While QA has not yet demonstrated a speed advantage over report on the theory and experimental results of a persistent classical computing on real-world applications, many current readout scheme using quantum flux parametrons as a important regions of QA design space have yet to be explored. current amplifier that provides fast, high-fidelity readout of the IARPA’s Quantum Enhanced Optimization (QEO) program is flux qubit state. exploring some of these regions, in particular areas with increased qubit coherence. Keywords—quantum annealing, quantum computing, qubit, coherence, readout The coherence of a quantum system is the time scale over which the system is expected to evolve in a quantum I. INTRODUCTION mechanical fashion. The coherence is further defined by two time scales, the relaxation time, T , and the dephasing time, T . Quantum annealing (QA) is an interesting candidate for 1 2 These times are measured with respect to a fixed Hamiltonian, new computing capability that began as a quantum-inspired but in QA systems the Hamiltonian is constantly changing classical optimization method in the 1980s [1] and was during the annealing process, and so it is important to eventually proposed as a type of analog quantum computing determine the basis in which the decoherence is occurring. [2][3][4]. The concept of operation typically involves Dephasing between energy eigenstates is harmless in QA, but initializing an isolated quantum system, applying analog dephasing in the computational basis is fatal to the computation controls such that the energy landscape represents the classical [8]. Dephasing events of the second kind are typically due to problem of interest, and then reading out the final state. If the thermal population of excited states and happen most often annealing operation is successful, the quantum system will when the temperature of the system is comparable to the have found the lowest energy state corresponding to the energy gaps and when the annealing operation time is solution to the original problem. In contrast with purely comparable to T . classical annealing, a quantum annealer should be able to take 1 advantage of quantum tunneling to navigate a complex energy Flux qubits [9][10] are a natural choice for implementing landscape and avoid being trapped in local minima. QA since the quantum states are characterized by persistent supercurrents flowing in opposite directions, and these currents There are several categories of problems where QA could can be mapped onto the binary spin variable used in QA make significant contributions, such as discrete combinatorial (Figure 1). optimization and in sampling applications such as those used in machine learning. For combinatorial optimization, there exist two other quantum approaches to compete with QA: a digital (Trotterized) variant of annealing [5] and the quantum This material is based upon work supported by the Intelligence Advanced Research Projects Activity (IARPA) through the Army Research Office (ARO) Contract No. W911NF-17-C-0050. Any opinions, findings and conclusions or recommendations expressed in this material are those of the author(s) and do not necessarily reflect the views of the Intelligence Advanced Research Projects Activity (IARPA) and the Army Research Office (ARO). Distribution Statement A: Approved for Public Release; Distribution is Unlimited; #19-1061; Dated 06/13/19 1 IEEE CSC & ESAS SUPERCONDUCTIVITY NEWS FORUM (global edition), September 2019. Invited presentation 1-QU-I-1 given at ISEC, 28 July-1 August 2019, Riverside, USA. Flux Qubit Schematic and Energy Levels Flux Qubit Readout (a) |↑⟩ |↑⟩ |↓⟩ |↓⟩ μW rf-SQUID Resonator Qubit feedline (b) Circulating currents in the qubit Z loop Figure 1 – A schematic of a tunable flux qubit showing the two control fluxes, ΦX and ΦZ, and the two persistent current directions corresponding to the ground and excited qubit state. Also shown are representations of the qubit energy potential both before (top) and after (bottom) the (c) annealing operation using ΦX. The qubits are first prepared in a state with a single potential well and no persistent current, and during the anneal a double well is created and the direction of the persistent current is determined by the effective bias due to the coupling with Figure 2 – (a) Schematic of a flux qubit readout method using an rf-SQUID to neighboring qubits. The coherence time of a flux qubit is sense the persistent current in the flux qubit and cause a corresponding shift strongly dependent on the magnitude of the persistent current in the frequency of an attached resonator. (b) Example of resonator 2 transmission data versus probe frequency and qubit Z bias. At integer values (Ip), and scales as T1~1/(Ip) and T2~1/Ip [13][14]. of Φ0 the frequency is extremely sensitive to small changes in persistent current. (c) New schematic that inserts a Quantum Flux Parametron (QFP) D-Wave Systems has performed much of the pioneering between the flux qubit and the rf-SQUID resonator. This acts as a current work in this field [11][12], but their work relies on very high amplifier and filter to enable faster readout. persistent currents (Ip ~ 3 µA), which severely limits the coherence time of their qubits. This work is performed using capacitively-shunted flux qubits [15] fabricated at MIT Lincoln flux loops, one controlling the anneal of the energy barrier and Laboratory that are designed to have very small persistent one where the persistent current is created, but the energy scale currents (Ip ~ 90 nA) and are optimized for long coherence is much larger than that of the flux qubit. The QFP junctions times [16][17]. are ten times the size of the flux qubit junctions, with a corresponding increase in the persistent current when it is annealed. III. QUBIT READOUT One of the chief difficulties in using qubits with such small The readout operation is performed in several sequential persistent currents is reading out the qubit state at the end of steps (Figure 3). Initially, both the flux qubit and the QFP are the computation. Previous work with these qubits used a in a single-well potential with minimal circulating current. The resonator terminated in an rf-SQUID, which acted as a flux qubit has no X flux bias and only a very small Z bias from magnetometer to sense the field created by the persistent neighboring coupled qubits and its local Z control flux, which current in the flux qubit [17]. Modulation of the magnetic flux will determine its final state. The QFP has an X bias of 0.5 Φ0, in the SQUID changed the effective inductance terminating the which causes the Josephson inductance to diverge and the resonator, which in turn shifted the resonator’s frequency magnetic susceptibility of the overall loop to go to zero. This (Figure 2). This technique worked well for giving a high- is critical because it effectively isolates the flux qubit from the fidelity readout (99.99%), but the resonator had to have a very rf-SQUID and readout resonator, allowing us to use a much high quality factor (Q ~ 10,000) in order to avoid detrimental lower Q resonator without decohering the qubit during the effects on the qubit’s coherence, resulting in a very slow computation phase. readout (~10 µs). The flux qubit X bias is then ramped to 1 Φ0, creating a We have since added a new modification to our readout double well potential and projecting the qubit state onto either method designed to preserve the high single-shot fidelity but in left or right circulating current. That current creates an a much faster timescale. By inserting a Quantum Flux effective Z bias on the QFP. Next, the QFP X bias is ramped Parametron (QFP) in between the flux qubit and the rf-SQUID to 1 Φ0 and it creates a persistent current whose direction is resonator (Figure 2), we can amplify the persistent current determined by the flux qubit, but whose amplitude is an order signal and also isolate the qubit from the resonator [18]. The of magnitude larger. The QFP is no longer isolating the qubit QFP is very similar in design to the flux qubit, in that it has two from the resonator at this point, but the flux qubit is in a protected classical state at the end of the computation.
Details
-
File Typepdf
-
Upload Time-
-
Content LanguagesEnglish
-
Upload UserAnonymous/Not logged-in
-
File Pages3 Page
-
File Size-