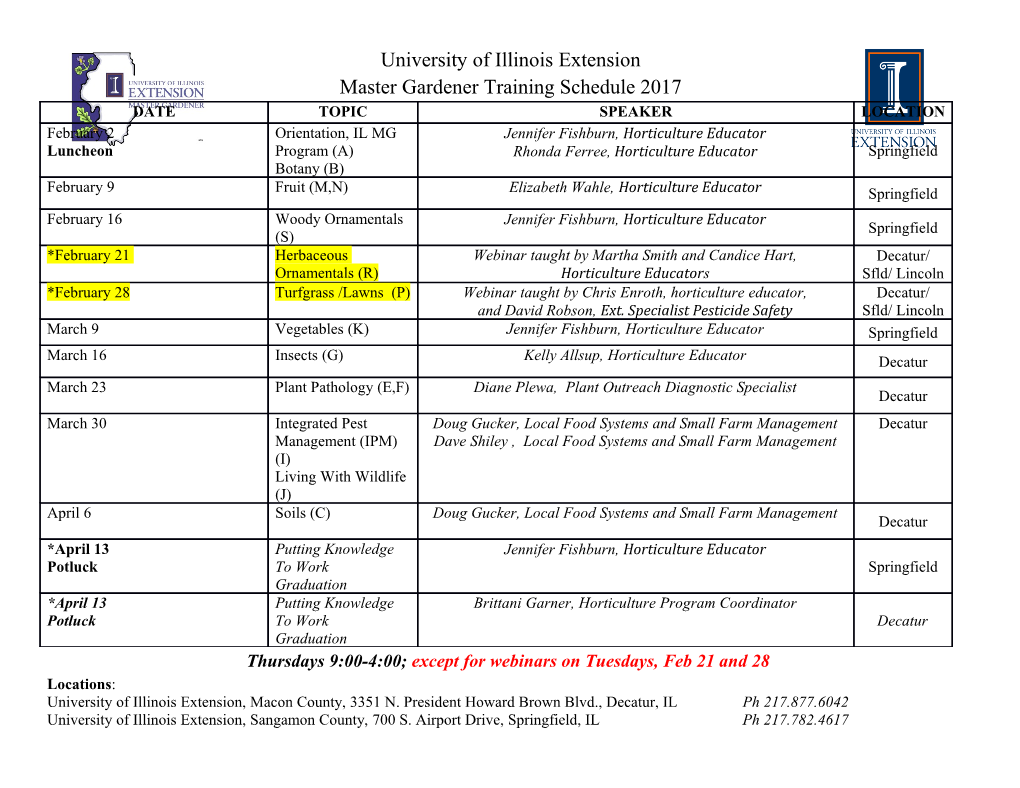
Proceedings of the Society for Computation in Linguistics Volume 3 Article 34 2020 What do you mean, BERT? Assessing BERT as a Distributional Semantics Model Timothee Mickus Université de Lorraine, CNRS, ATILF, [email protected] Denis Paperno Utrecht University, [email protected] Mathieu Constant Université de Lorraine, CNRS, ATILF, [email protected] Kees van Deemter Utrecht University, [email protected] Follow this and additional works at: https://scholarworks.umass.edu/scil Part of the Computational Linguistics Commons Recommended Citation Mickus, Timothee; Paperno, Denis; Constant, Mathieu; and van Deemter, Kees (2020) "What do you mean, BERT? Assessing BERT as a Distributional Semantics Model," Proceedings of the Society for Computation in Linguistics: Vol. 3 , Article 34. DOI: https://doi.org/10.7275/t778-ja71 Available at: https://scholarworks.umass.edu/scil/vol3/iss1/34 This Paper is brought to you for free and open access by ScholarWorks@UMass Amherst. It has been accepted for inclusion in Proceedings of the Society for Computation in Linguistics by an authorized editor of ScholarWorks@UMass Amherst. For more information, please contact [email protected]. What do you mean, BERT? Assessing BERT as a Distributional Semantics Model Timothee Mickus Denis Paperno Mathieu Constant Kees van Deemter Universite´ de Lorraine Utrecht University Universite´ de Lorraine Utrecht University CNRS, ATILF [email protected] CNRS, ATILF [email protected] [email protected] [email protected] Abstract based on BERT. Furthermore, BERT serves both as a strong baseline and as a basis for a fine- Contextualized word embeddings, i.e. vector tuned state-of-the-art word sense disambiguation representations for words in context, are nat- urally seen as an extension of previous non- pipeline (Wang et al., 2019a). contextual distributional semantic models. In Analyses aiming to understand the mechanical this work, we focus on BERT, a deep neural behavior of Transformers in general, and BERT in network that produces contextualized embed- particular, have suggested that they compute word dings and has set the state-of-the-art in several representations through implicitly learned syntac- semantic tasks, and study the semantic coher- tic operations (Raganato and Tiedemann, 2018; ence of its embedding space. While showing Clark et al., 2019; Coenen et al., 2019; Jawa- a tendency towards coherence, BERT does not fully live up to the natural expectations for a har et al., 2019, a.o.): representations computed semantic vector space. In particular, we find through the ‘attention’ mechanisms of Transform- that the position of the sentence in which a ers can arguably be seen as weighted sums of word occurs, while having no meaning corre- intermediary representations from the previous lates, leaves a noticeable trace on the word em- layer, with many attention heads assigning higher beddings and disturbs similarity relationships. weights to syntactically related tokens (however, contrast with Brunner et al., 2019; Serrano and 1 Introduction Smith, 2019). A recent success story of NLP, BERT (Devlin et al., Complementing these previous studies, in this 2018) stands at the crossroad of two key innova- paper we adopt a more theory-driven lexical se- tions that have brought about significant improve- mantic perspective. While a clear parallel was es- ments over previous state-of-the-art results. On tablished between ‘traditional’ noncontextual em- the one hand, BERT models are an instance of con- beddings and the theory of distributional seman- textual embeddings (McCann et al., 2017; Peters tics (a.o. Lenci, 2018; Boleda, 2019), this link is et al., 2018), which have been shown to be subtle not automatically extended to contextual embed- and accurate representations of words within sen- dings: some authors (Westera and Boleda, 2019) tences. On the other hand, BERT is a variant of the even explicitly consider only “context-invariant” Transformer architecture (Vaswani et al., 2017) representations as distributional semantics. Hence which has set a new state-of-the-art on a wide we study to what extent BERT, as a contextual em- variety of tasks ranging from machine translation bedding architecture, satisfies the properties ex- (Ott et al., 2018) to language modeling (Dai et al., pected from a natural contextualized extension of 2019). BERT-based models have significantly in- distributional semantics models (DSMs). creased state-of-the-art over the GLUE benchmark DSMs assume that meaning is derived from use for natural language understanding (Wang et al., in context. DSMs are nowadays systematically 2019b) and most of the best scoring models for represented using vector spaces (Lenci, 2018). this benchmark include or elaborate on BERT. Us- They generally map each word in the domain of ing BERT representations has become in many the model to a numeric vector on the basis of cases a new standard approach: for instance, all distributional criteria; vector components are in- submissions at the recent shared task on gendered ferred from text data. DSMs have also been com- pronoun resolution (Webster et al., 2019) were puted for linguistic items other than words, e.g., 350 Proceedings of the Society for Computation in Linguistics (SCiL) 2020, pages 350-361. New Orleans, Louisiana, January 2-5, 2020 word senses—based both on meaning inventories sentence coherence. In section 4, we test whether (Rothe and Schutze¨ , 2015) and word sense induc- we can observe cross-sentence coherence for sin- tion techniques (Bartunov et al., 2015)—or mean- gle tokens, whereas in section 5 we study to what ing exemplars (Reisinger and Mooney, 2010; Erk extent incoherence of representations across sen- and Pado´, 2010; Reddy et al., 2011). The default tences affects the similarity structure of the seman- approach has however been to produce represen- tic space. We summarize our findings in section 6. tations for word types. Word properties encoded by DSMs vary from morphological information 2 Theoretical background & connections (Marelli and Baroni, 2015; Bonami and Paperno, 2018) to geographic information (Louwerse and Word embeddings have been said to be ‘all- Zwaan, 2009), to social stereotypes (Bolukbasi purpose’ representations, capable of unifying the et al., 2016) and to referential properties (Herbe- otherwise heterogeneous domain that is NLP (Tur- lot and Vecchi, 2015). ney and Pantel, 2010). To some extent this claim spearheaded the evolution of NLP: focus recently A reason why contextualized embeddings have shifted from task-specific architectures with lim- not been equated to distributional semantics may ited applicability to universal architectures requir- lie in that they are “functions of the entire input ing little to no adaptation (Radford, 2018; Devlin sentence” (Peters et al., 2018). Whereas tradi- et al., 2018; Radford et al., 2019; Yang et al., 2019; tional DSMs match word types with numeric vec- Liu et al., 2019, a.o.). tors, contextualized embeddings produce distinct Word embeddings are linked to the distribu- vectors per token. Ideally, the contextualized na- tional hypothesis, according to which “you shall ture of these embeddings should reflect the seman- know a word from the company it keeps” (Firth, tic nuances that context induces in the meaning of 1957). Accordingly, the meaning of a word can be a word—with varying degrees of subtlety, rang- inferred from the effects it has on its context (Har- ing from broad word-sense disambiguation (e.g. ris, 1954); as this framework equates the meaning ‘bank’ as a river embankment or as a financial of a word to the set of its possible usage contexts, institution) to narrower subtypes of word usage it corresponds more to holistic theories of meaning (‘bank’ as a corporation or as a physical building) (Quine, 1960, a.o.) than to truth-value accounts and to more context-specific nuances. (Frege, 1892, a.o.). In early works on word em- Regardless of how apt contextual embeddings beddings (Bengio et al., 2003, e.g.), a straightfor- such as BERT are at capturing increasingly finer ward parallel between word embeddings and dis- semantic distinctions, we expect the contextual tributional semantics could be made: the former variation to preserve the basic DSM properties. are distributed representations of word meaning, Namely, we expect that the space structure en- the latter a theory stating that a word’s meaning is codes meaning similarity and that variation within drawn from its distribution. In short, word embed- the embedding space is semantic in nature. Simi- dings could be understood as a vector-based im- lar words should be represented with similar vec- plementation of the distributional hypothesis. This tors, and only semantically pertinent distinctions parallel is much less obvious for contextual em- should affect these representations. We connect beddings: are constantly changing representations our study with previous work in section 2 be- truly an apt description of the meaning of a word? fore detailing the two approaches we followed. More precisely, the literature on distributional First, we verify in section 3 that BERT embeddings semantics has put forth and discussed many math- form natural clusters when grouped by word types, ematical properties of embeddings: embeddings which on any account should be groups of similar are equivalent to count-based matrices (Levy and words and thus be assigned similar vectors. Sec- Goldberg, 2014b), expected to be linearly depen- ond, we test in sections 4 and 5 that contextualized dant (Arora et al., 2016), expressible as a unitary word vectors do not encode semantically irrelevant matrix (Smith et al., 2017) or as a perturbation features: in particular, leveraging some knowledge of an identity matrix (Yin and Shen, 2018). All from the architectural design of BERT, we address these properties have however been formalized for whether there is no systematic difference between non-contextual embeddings: they were formulated BERT representations in odd and even sentences using the tools of matrix algebra, under the as- of running text—a property we refer to as cross- sumption that embedding matrix rows correspond 351 to word types.
Details
-
File Typepdf
-
Upload Time-
-
Content LanguagesEnglish
-
Upload UserAnonymous/Not logged-in
-
File Pages13 Page
-
File Size-