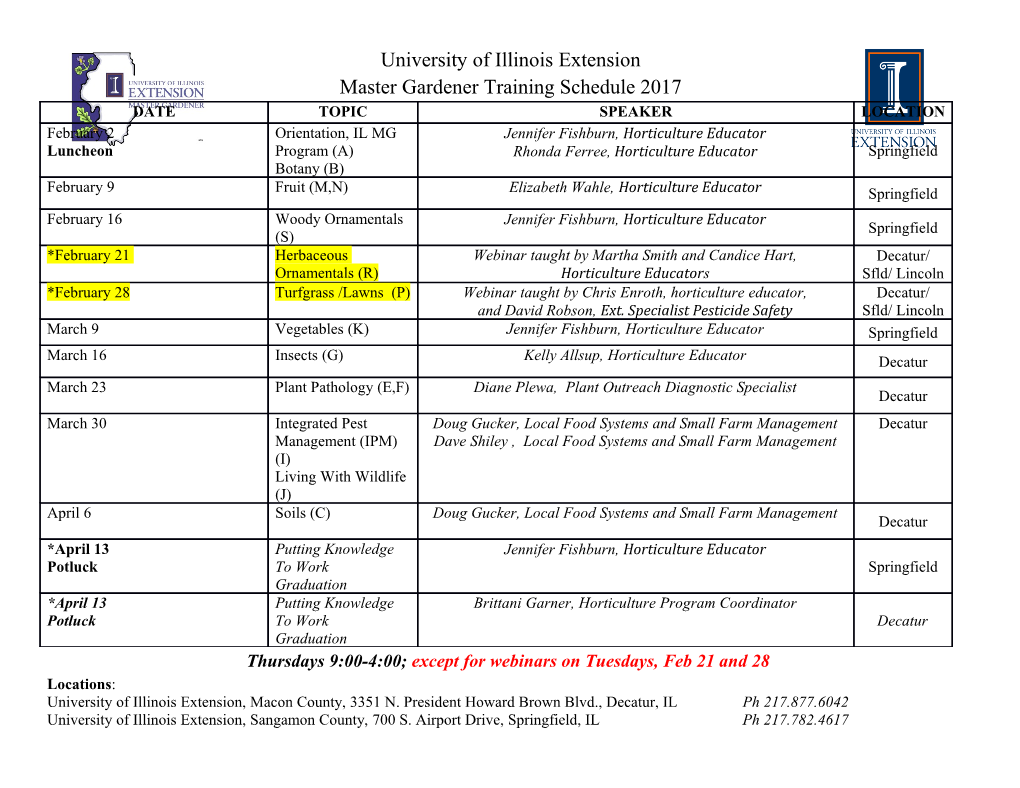
bioRxiv preprint doi: https://doi.org/10.1101/364323; this version posted July 11, 2018. The copyright holder for this preprint (which was not certified by peer review) is the author/funder, who has granted bioRxiv a license to display the preprint in perpetuity. It is made available under aCC-BY-NC-ND 4.0 International license. Deep Learning Based Tumor Type Classification Using Gene Expression Data Boyu Lyu Anamul Haque Virginia Tech Virginia Tech Blacksburg, Virginia Blacksburg, Virginia [email protected] [email protected] ABSTRACT Pan-Cancer Atlas. Where, as to each tumor sample, we could ac- Differential analysis occupies the most significant portion ofthe cess its RNA-Seq expression data. These data are beneficial when standard practices of RNA-Seq analysis. However, the conventional trying to identify potential biomarkers for each tumor. method is matching the tumor samples to the normal samples, which As to biomarkers, most analyses tried to find the differentially are both from the same tumor type. The output using such method expressed genes. However, they didnt consider expressions of other would fail in differentiating tumor types because it lacks the knowl- tumors. Also, during the study, models are constructed to mimic edge from other tumor types. Pan-Cancer Atlas provides us with the expression data. However, such models are very data specific, abundant information on 33 prevalent tumor types which could which would fail in dealing with data from other samples or other be used as prior knowledge to generate tumor-specific biomarkers. tumor types. So, it is highly needed to build a method which can In this paper, we embedded the high dimensional RNA-Seq data include knowledge from multiple tumor types into the analysis. into 2-D images and used a convolutional neural network to make On the other hand, tumor type classification can contribute to classification of the 33 tumor types. The final accuracy wegotwas a faster and more accurate diagnosis. However, research on tumor 95.59%, higher than another paper applying GA/KNN method on type classification is currently rare, which is partially due to thedif- the same dataset. Based on the idea of Guided Grad Cam, as to each ficulty in dealing with high dimensionality of the genomic dataset. class, we generated significance heat-map for all the genes. By do- In Pan-Cancer Atlas, the normalized mRNA-seq gene expression ing functional analysis on the genes with high intensities in the data contains information from more than 20k genes. Within that heat-maps, we validated that these top genes are related to tumor- many genes, a lot of genes are actually of no specific effect on specific pathways, and some of them have already been usedas the tumor. These genes are weak features. Using generic machine biomarkers, which proved the effectiveness of our method. As far learning methods such as KNN wont work due to the curse of di- as we know, we are the first to apply convolutional neural network mension. Even though classification of tumor type is still inits on Pan-Cancer Atlas for classification, and we are also the first beginning, deep learning has been vastly used in image classifi- to match the significance of classification with the importance of cation/ recognition, some famous architectures like Resnet [9] and genes. Our experiment results show that our method has a good inception[17] have excellent performance. In the Imagenet 2017 performance and could also apply in other genomics data. challenge, the winner obtained a top-5 error rate of 2.251%[10] us- ing a convolutional neural network. Besides, to understand how KEYWORDS deep neural network works, in computer vision field, various vi- sualization methods have been invented such as deep Taylor de- Deep Learning, Tumor Type Classification, Pan-Cancer Atlas, Con- composition, layer-wise decomposition, and Grad-Cam[16]. They volutional Neural Network generate heat-maps in the input image to indicate the contribu- tion of each pixel to the classification. Since the gene expression 1 INTRODUCTION data contains more than 10k samples in the atlas, it is promising to have good accuracy with the deep neural network. At the same The invention of Next Generation Sequencing methods has mostly time, assuming that the importance of genes equals to the signifi- boosted the analysis of human genomics due to the improvement cance of genes in classification, we could borrow the interpretation in the efficiency and accuracy. To better understand the causeof methods of the deep neural network to discover top genes for each various tumors, a large volume of tumor tissues has been sequenced tumor. In this way, each gene will be assigned a confidence score, and managed by The Cancer Genome Atlas (TCGA). With these and genes with high scores are considered to be the top genes or samples, TCGA further analyzed over 11,000 tumors from 33 most potential biomarkers since their existence affect the classification prevalent forms of cancer, which fostered the accomplishment of most. Based on the deep neural network and the visualization meth- ods, we first filter out the genes with small variance across allthe Permission to make digital or hard copies of part or all of this work for personal or classroom use is granted without fee provided that copies are not made or distributed samples. Then, we embedded the high-dimension expression data for profit or commercial advantage and that copies bear this notice and the full citation (10381x1) into a 2-D image (102x102) to fit for the convolutional on the first page. Copyrights for third-party components of this work must be honored. For all other uses, contact the owner/author(s). layers. Next, we constructed a three-layer convolution neural net- ACM-BCB ’18, August 2018, Washington, D.C., USA work and used 10-fold cross-validation to test the performance. © 2018 Copyright held by the owner/author(s). With the trained neural network, we followed the idea of Guided ACM ISBN 978-x-xxxx-xxxx-x/YY/MM. https://doi.org/10.1145/nnnnnnn.nnnnnnn bioRxiv preprint doi: https://doi.org/10.1101/364323; this version posted July 11, 2018. The copyright holder for this preprint (which was not certified by peer review) is the author/funder, who has granted bioRxiv a license to display the preprint in perpetuity. It is made available under aCC-BY-NC-ND 4.0 International license. Grad-Cam[16] and generated heat-maps for all the classes show- Log2 transform ing prominent pixels (genes). By functional analysis, we validated that the top genes selected in this way are biologically meaningful for corresponding tumors, which proves the effectiveness of our Preprocessing Filter based on an work. annotation file and variance The remaining of this paper is organized as follows. In section 2, we reviewed the related work of tumor type classification and Tumor type classification visualization of the deep neural network. In section 3, we described using CNN Embedding to 2-D image the data we used, and the process of we applied for tumor type classification. Also, the steps to generate heat map for each sample are also explained. Finally, in section 4, The accuracy of the tumor Generate Heatmap with 10 fold cross Guided Grad Cam validation type classification is discussed and compared with the result of GA/KNN method in paper[11]. Also, top genes are picked from the heat-maps and validated by functional analysis. Validation of top genes 2 RELATED WORK Binary classification (identification) of tumors has been found in Figure 1: The workflow of our method some papers, but only paper [11] researched multiple tumor type classification using gene expression data. The authors applied GA/ KNN method to iteratively generate the subset of the genes (fea- this method to trace the significant pixels (genes) in the input, so tures) and then use KNN method to test the accuracy. After hun- that we could extract substantial genes. The localization map of dreds of iterations, they obtained the feature set corresponding to Grad Cam is generated by first calculating the activation map in the best accuracy. In this way, this method achieved an accuracy of the forward propagation and the gradient map in the backward 90% across 31 tumor types, and also generated a set of top genes for propagation. Since the deeper the convolutional layer, the higher all the tumor type. GA/KNN method is a straightforward method level feature it contains. And after sending into the fully connected which could obtain an optimal feature set in the end, but it requires layer, all the spatial information will be lost. So, Grad-Cam con- running a lot of iterations and also fixes the size of feature setat structs the localization map of the last convolutional layer and re- first. However, using one single feature set to make classification sizes it to the input size. However, as stated by the [5], the heat map overlooked the heterogeneity among different tumor types. Also, shows the importance of each class, but the resolution is low, due top genes for various tumor types could be varied a lot. to the resizing process. To solve this problem, a pixel-space gra- Deep learning method was also used to identify top genes and dient visualization method, guided back propagation, was used to make cancer identification of one single type. In paper [5], theau- refine the heat map. The output of guided backpropagation isthe thor used a stacked auto-encoder first to extract high-level features gradient of each pixel in the input image, which can be obtained from the expression values and then input these features into a sin- through the backpropagation.
Details
-
File Typepdf
-
Upload Time-
-
Content LanguagesEnglish
-
Upload UserAnonymous/Not logged-in
-
File Pages8 Page
-
File Size-