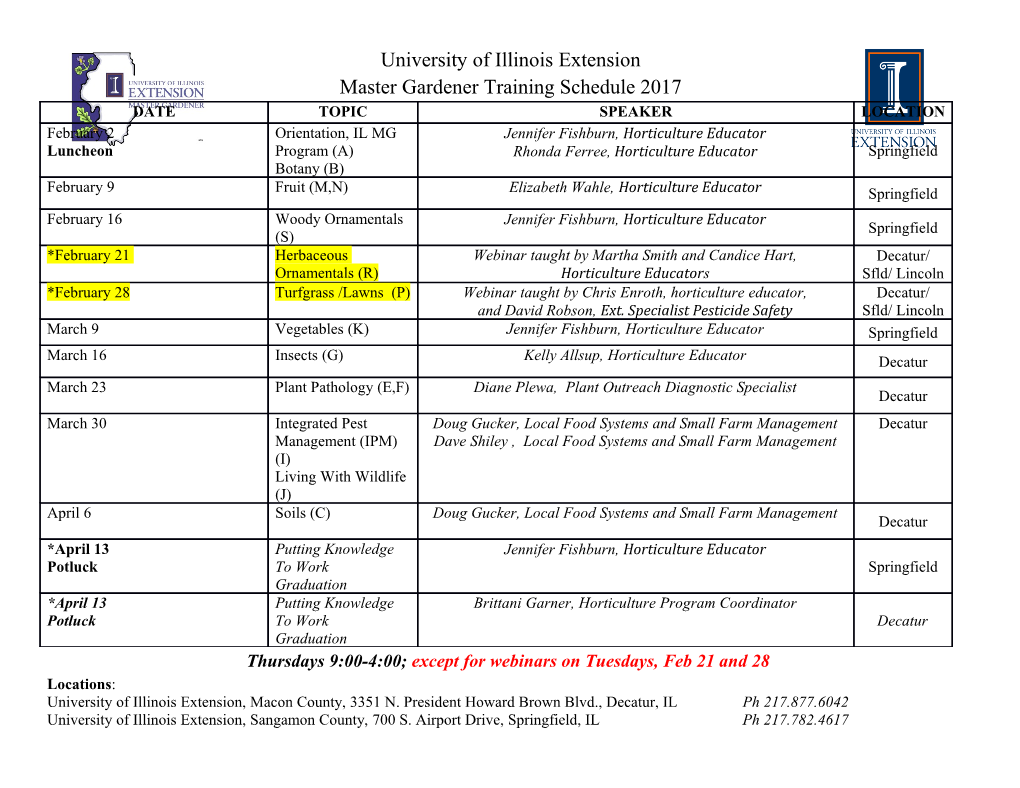
Bioinformatics Algorithms Multiple Sequence Alignment David Hoksza http://siret.ms.mff.cuni.cz/hoksza Outline • Motivation • Algorithms • Scoring functions • exhaustive • multidimensional dynamic programming • heuristics • progressive alignment • iterative alignment/refinement • block(local)-based alignment Multiple sequence alignment (MSA) • Goal of MSA is to find “optimal” mapping of a set of sequences • Homologous residues (originating in the same position in a common ancestor) among a set of sequences are aligned together in columns • Usually employs multiple pairwise alignment (PA) computations to reveal the evolutionarily equivalent positions across all sequences Motivation • Distant homologues • faint similarity can become apparent when present in many sequences • motifs might not be apparent from pairwise alignment only • Detection of key functional residues • amino acids critical for function tend to be conserved during the evolution and therefore can be revealed by inspecting sequences within given family • Prediction of secondary/tertiary structure • Inferring evolutionary history 4 Representation of MSA • Column-based representation • Profile representation (position specific scoring matrix) • Sequence logo Manual MSA • High quality MSA can be carried out automatic MSA algorithms by hand using expert knowledge • specific columns • BAliBASE • highly conserved residues • https://lbgi.fr/balibase/ • buried hydrophobic residues • PROSITE • secondary structure (especially in RNA • http://prosite.expasy.org/ alignment) • Pfam • expected patterns of insertions and • http://pfam.sanger.ac.uk/ deletions • TIGRFAM • http://www.jcvi.org/cgi- bin/tigrfams/index.cgi • Tedious, but • … (some databases are semi-automatic • high-quality source of family and many of the databases construct the information MSA from the structure information) • a benchmark for evaluation of Scoring • How to score an MSA? 푺 푨 = 푮 + 푪푺(푨풊) • 퐴푖 … 푖-th column • 퐶푆(퐴푖) … score of the 푖-th column • 퐺 … gap function (assumes linear or constant gap penalty) • the score assumes independent columns • Two score types are usually considered • minimum entropy (ME) • sum of pairs (SP) Minimum entropy (1) • ME aims to minimize entropy of each column • columns with low entropy (can be expressed with only few bits) are good for the alignment • the more bits we need to express a column, the more divers the column is Minimum entropy (2) • Probability of a column • assumption of independency between columns and residues within columns 풄풊풂 푷 푨풊 = ෑ 풑풊풂 풂 0 퐴푖 [푗] ≠ 푎 • 푐푖푎…observed counts for residue 푎 in 푖-th column 푐푖푎 = σ푗 ൝ 1 퐴푖 푗 = 푎 • 퐴푖 [푗]… 푗-th symbol in 푖-th column • 푝푖푎… probability of residue 푎 in column 푖 푪푴푬 푨풊 = − 풄풊풂 퐥퐨퐠 풑풊풂 푴푬 = 푪푴푬(푨풊) 풂 풊 • completely conserved column would score 0 Sum of pairs • Sum of scores of all possible pairs in a multiple alignment 푨 for a particular scoring matrix • Score for each column is computed as the sum of all pairs of position in that column • Column scores are then summed to get the SP-score |퐴| |퐴| 푆푃 퐴 = 퐶푆푃 퐴푖 = 휎(퐴푖 푘 , 퐴푖 푙 ) 푖=1 푖=1 푘<푙 • 퐴푖 [푘]… 푘-th symbol in 푖-th column • 휎(푥, 푦) … PAM or BLOSUM values for the residue 푥 and 푦 G K N SP - Example T R N S H E • BLOSUM 62 scoring matrix -1 +1 +6 6 SP score drawback • Alignment of 푵 sequences, all containing leucine at given position from functional reasons • BLOSUM62 matrix 흈 푳, 푳 = ퟒ → 푺푷 푨풊 = ퟒ × 푵(푵 − ퟏ)/ퟐ • Let us replace one of the leucines with glycine (incorrect alignment) 흈 푳, 푮 = −ퟒ → the score decreases by ퟖ × (푵 − ퟏ) • 푺푷 푨풊 is worse by a fraction of 8×(푁−1) ퟒ = 4×푁(푁−1)/2 푵 • Relative difference in score between the correct alignment and incorrect alignment decreases with the number of sequences in the alignment • BUT increasing the number of sequences (evidence) should give us more increased relative difference Multidimensional dynamic programming (1) • Generalization of pairwise dynamic programming • 3 sequences: ATGC, AATC,TTGC 0 1 1 2 3 4 x coordinate A - T G C 0 1 2 3 3 4 y coordinate A A T - C 0 0 1 2 3 4 z coordinate - T T G C • Resulting path • (0,0,0) → (1,1,0) → (1,2,1) → (2,3,2) → (3,3,3) → (4,4,4) Multidimensional dynamic programming (2) • Let us assume linear gap penalty model (not affine) • 훾 푔 = 푔푑 for a gap of length 푔 and gap cost 푑 • initialization and backtracking are analogous with the 2D case Multidimensional dynamic programming (3) • 3 edges • 7 edges Computational complexity of MDP • Computation of each cell of the DP matrix takes ퟐ푵 − ퟏ (all possible combinations of gaps column) • Let us assume all the sequences have approximately the same length 푳 • Memory complexity 푶 푳푵 • Time complexity 푶 ퟐ푵푳푵 MDP - exercise • Let’s have sequence of length 50 • Comparison of a pair of sequences using DP takes 0,1s • What is the time needed to compare 4 sequences? • Let’s say we have 1000 years and average sequence length is 50. • How many sequence can afford to compare? Heuristic Algorithms • Progressive alignment methods • iterative building of the alignment • Block-based alignment • Feng & Doolittle • local alignment built by identifying • ClustalW, Clustal Omega blocks of ungapped MSA identified and assembled • Consistency-based methods • DIALIGN • T-Coffee • Mix of approaches • Iterative refinement • MAFFT, MUSCLE • alignment built and then refined be realigning the constituent sequences • Barton & Sternberg Progressive alignment • Framework • First, two sequences are aligned using standard pairwise alignment • The remaining sequences are taken one by one and aligned to the previous ones • Repeated until all sequences are aligned • Parameters • The order in which the sequences are be aligned • Whether only one alignment is kept and sequences are added to it or whether also an alignment can be aligned to another alignment (as if a tree was being built) • The process used to align and score sequences or alignments against the existing ones Star alignment • N sequences 풔ퟏ, … , 풔푵 to be aligned 1. Pick 풔풊 as a starting sequence – center 2. Compute all optimal global alignments between 풔풊 and 풔풋, 푗 ≠ 푖 3. Successively merge sequences into the arising MSA • once a gap always a gap rule • if a gap is introduced into the MSA it stays there forever SA – example (1) S1: ATTGCCATT ATTGCC-ATT-- S2: ATGGCCATT ATTGCCATT ATGGCC-ATT-- S3: ATCCAATTTT ATGGCCATT ATTGCCGATT-- S4: ATCTTCTT ATCTTC--TT-- S5: ATTGCCGATT ATC-CA-ATTTT ATTGCC-ATT ATTGCCATT-- ATTGCCATT ATTGCCGATT ATC-CAATTTT ATTGCCATT ATCTTC-TT credit: Xingquan Zhu, Florida Atlantic University SA – example (2) pairwise alignment multiple alignment ATTGCCATT ATTGCCATT 1. ATGGCCATT ATGGCCATT ATTGCCATT-- ATTGCCATT-- 2. ATGGCCATT-- ATC-CAATTTT ATC-CAATTTT ATTGCCATT-- ATTGCCATT ATGGCCATT-- 3. ATCTTC-TT ATC-CAATTTT ATCTTC-TT-- ATTGCC-ATT-- ATGGCC-ATT-- ATTGCC-ATT 4. ATC-CA-ATTTT ATTGCCGATT ATCTTC--TT-- ATTGCCGATT-- SA - choosing the center • Compute all pairwise alignment and pick sequence 풔풊 with maximum σ풋≠풊 풔(풔풊, 풔풋) • Choosing the sequence which is most similar to all the rest • Compute all pairwise alignments and compute MSA for every 풔풊 and pick the best SA – time complexity • Average sequence length 퐿 • One global alignment computation in 퐎(푳ퟐ) • 푘 sequences → 퐎(풌ퟐ푳ퟐ) pairwise computations • 푙 … upper bound on the MSA length → 퐎(풍풌) for MSA construction 푂 푘2퐿2 + 푙푘 = 푶(풌ퟐ푳ퟐ) SA - exercise • Compute SP for the constructed MSA • Compute SA for the previous example but add sequences to the MSA in different order. Does the order of addition impacts the score? • Compute MSA starting with S5. Does the score change? ATTGCC-ATT ATGGCC-ATT AT--CCAATTTT AT--CTTCTT ATTGCCGATT ATTGCCGATT ATTGCCGATT-- ATTGCCGATT Feng & Doolittle (1) 푆 푎,푎 +푆 푏,푏 • 푆 푎, 푏 = 푚푎푥 2 1. Calculate a distance matrix from all-to-all pairwise • 푆푟푎푛푑 is an expected score alignments (푁(푁 − 1)/2) obtained by randomization • 푆푒푓푓 can be viewed as normalized 2. Convert raw alignment scores into (evolutionary) distances percentage similarity which decreases roughly exponentially to 0 with increasing evolutionary distance. • –log makes the measure linear with 푆표푏푠−푆푟푎푛푑 • 퐷 = − log 푆푒푓푓 × 100 = − log × 100 evolutionary distance 푆푚푎푥−푆푟푎푛푑 3. Construct a guide tree from the distance matrix using Fitch & Margoliash algorithm 4. Align child nodes of each parent (can be sequence- sequence, sequence-MSA, MSA-MSA) in the order they were added to the tree source: Feng, Da-Fei, and Russell F. Doolittle. "Progressive sequence alignment as a prerequisitetto correct phylogenetic trees." Journal of molecular evolution 25.4 (1987): 351-360. Feng & Doolittle (2) • Sequence-sequence is aligned using classical dynamic programming • Sequence-MSA – sequence is aligned with each sequence in the group and the highest scoring alignment defines how the sequence is added to the group • MSA-MSA – as in previous case but all pairs of sequences are tested • When a sequence is added to a group, neutral symbol X is introduced instead of the gap position • allows to align gap positions • neutral – anything aligned with X scores 0 • side effect – the gaps in two MSAs tend to come together in the resulting MSA Profile/MSA Alignment • When adding a sequence to a group it is desirable to take into account the MSA built so far • mismatches at highly conserved positions should be penalized more • 2 MSA (profiles) of 푁 sequences, one from 1. 푛, second 푛 + 1. 푁 푺 푨 풊 = 흈(푨풌 풊 , 푨풍 풊 ) 풊 풊 풌<풍≤푵 = 흈(푨풌 풊 , 푨풍 풊 ) + 흈(푨풌 풊 , 푨풍 풊 ) + 흈(푨풌 풊 , 푨풍 풊 ) 풊 풌<풍≤풏 풊 풏<풌<풍≤푵 풊 풌≤풏,풏<풍≤푵 • The score of the σ푖 σ푘<푙≤푁 휎(퐴푘 푖 , 퐴푙 푖 ) consists of the in-group scores plus between group scores • when aligning the profiles we can use standard dynamic programming where columns are aligned against columns using the in-between scores • → using position-specific information from the group’s multiple alignment ClustalW • Similar to Feng & Doolittle but uses profile-based building 1.
Details
-
File Typepdf
-
Upload Time-
-
Content LanguagesEnglish
-
Upload UserAnonymous/Not logged-in
-
File Pages66 Page
-
File Size-