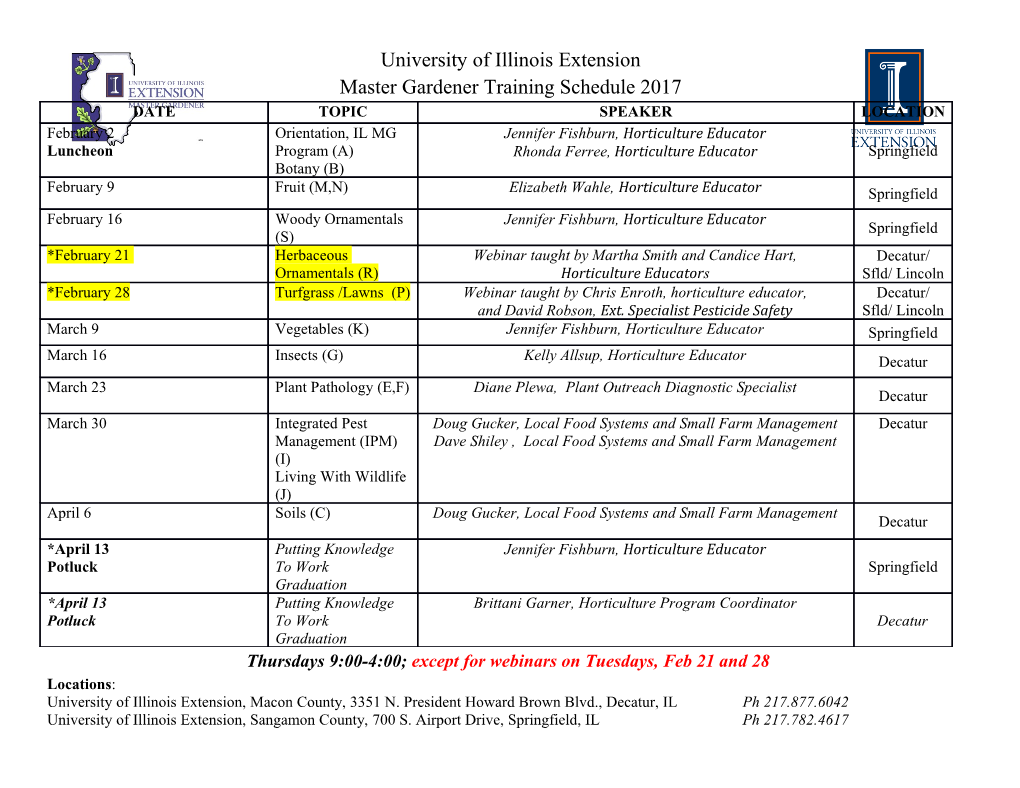
UCSF UC San Francisco Previously Published Works Title Predicting environmental chemical factors associated with disease-related gene expression data. Permalink https://escholarship.org/uc/item/1kj3j6m8 Journal BMC medical genomics, 3(1) ISSN 1755-8794 Authors Patel, Chirag J Butte, Atul J Publication Date 2010-05-06 DOI 10.1186/1755-8794-3-17 Peer reviewed eScholarship.org Powered by the California Digital Library University of California Patel and Butte BMC Medical Genomics 2010, 3:17 http://www.biomedcentral.com/1755-8794/3/17 RESEARCH ARTICLE Open Access PredictingResearch article environmental chemical factors associated with disease-related gene expression data Chirag J Patel1,2,3 and Atul J Butte*1,2,3 Abstract Background: Many common diseases arise from an interaction between environmental and genetic factors. Our knowledge regarding environment and gene interactions is growing, but frameworks to build an association between gene-environment interactions and disease using preexisting, publicly available data has been lacking. Integrating freely-available environment-gene interaction and disease phenotype data would allow hypothesis generation for potential environmental associations to disease. Methods: We integrated publicly available disease-specific gene expression microarray data and curated chemical- gene interaction data to systematically predict environmental chemicals associated with disease. We derived chemical- gene signatures for 1,338 chemical/environmental chemicals from the Comparative Toxicogenomics Database (CTD). We associated these chemical-gene signatures with differentially expressed genes from datasets found in the Gene Expression Omnibus (GEO) through an enrichment test. Results: We were able to verify our analytic method by accurately identifying chemicals applied to samples and cell lines. Furthermore, we were able to predict known and novel environmental associations with prostate, lung, and breast cancers, such as estradiol and bisphenol A. Conclusions: We have developed a scalable and statistical method to identify possible environmental associations with disease using publicly available data and have validated some of the associations in the literature. Background environmental chemical factor and genomic data may The etiology of many diseases results from interactions facilitate the discovery of these associations. between environmental factors and biological factors [1]. We desired to use pre-existing datasets and knowledge- Our knowledge regarding interaction between environ- bases in order to derive hypotheses regarding chemical mental factors, such chemical exposure, and biological association to disease without upfront experimental factors, such as genes and their products, is increasing design. Specifically, we asked what environmental chemi- with the advent of high-throughput measurement modal- cals could be associated with gene expression data of dis- ities. Building associations between environmental and ease states such as cancer, and what analytic methods and genetic factors and disease is essential in understanding data are required to query for such correlations. This pathogenesis and creating hypotheses regarding disease study describes a method for answering these questions. etiology. However, it is currently difficult to ascertain We integrated publicly available data from gene expres- multiple associations of chemicals to genes and disease sion studies of cancer and toxicology experiments to without significant experimental investment or large- examine disease/environment associations. Central to scale epidemiological study. Use of publicly-available our investigation was the Comparative Toxicogenomics Database (CTD) [2], which contains information about chemical/gene/protein interactions and chemical/gene/ * Correspondence: [email protected] 1 Department of Pediatrics, Stanford University School of Medicine, Stanford, disease relationships, and the Gene Expression Omnibus CA 94305, USA (GEO) [3], the largest public gene expression data reposi- Full list of author information is available at the end of the article © 2010 Patel and Butte; licensee BioMed Central Ltd. This is an Open Access article distributed under the terms of the Creative Com- BioMed Central mons Attribution License (http://creativecommons.org/licenses/by/2.0), which permits unrestricted use, distribution, and reproduc- tion in any medium, provided the original work is properly cited. Patel and Butte BMC Medical Genomics 2010, 3:17 Page 2 of 17 http://www.biomedcentral.com/1755-8794/3/17 tory. Information in the CTD is curated from the peer- [11]. Their method utilizes the Genetic Association Data- reviewed literature, while gene expression data in GEO is base (GAD) [12] to associate phenotypes to genetic path- uploaded by submitters of manuscripts. ways and the CTD to link pathways to environmental Most approaches to date to associate environmental factors. This method has proved its utility, allowing for chemicals with genome-wide changes can be put into 2 production of hypotheses for chemicals associated with categories. These approaches either 1.) have tested a diseases categorized as metabolic or neuropsychiatric small number of chemicals on cells and measured disorders. However, in its current configuration, their responses on a genomic scale, or 2.) used existing knowl- method is dependent on the GAD, which contains stati- edge bases, such as Gene Ontology, to associate anno- cally annotated phenotypes in relation to genes contain- tated pathways to environmental insult. ing variants; such DNA changes are not likely to be The first method involves measuring physiological reflective of molecular profiles of tissues being suspected response on a gene expression microarray. This approach for environmental influence. Unlike this method, our allows researchers to test chemical association on a proposed approach is tissue- and data-driven in that the genomic scale, but the breadth of discoveries is con- phenotype is determined by the individual measurements strained by the number of chemicals tested against a cell of gene expression in cells and tissues, allowing for the line or model organism. These experiments are not dynamic capture of phenotypes. intended for hypothesis generation across hundreds of The approach we propose here is agnostic to experi- potential chemical factors with multiple phenotypic ment protocol, such as cell line or chemical agent tested, states. Only a few chemicals can be tractably tested for and provides for a less resource-intensive screening of association to gene activity [4,5], or disease on cell lines chemicals to biologically validate. Our methodology [6], or on model organisms, including rat and mouse [7]. essentially combines the best features of these current In rare cases, this approach has reached the level of a approaches. We start by compiling "chemical signatures" hundred or thousand chemical compounds, such as the in a scalable way using the CTD. These chemical signa- Connectivity Map, developed by Lamb, Golub, and col- tures capture known changes in gene expression second- leagues [8], which attempts to associate drugs with gene ary to hundreds of environmental chemicals. In a manner expression changes. After measuring the genome-wide similar to how Gene Ontology categories are tested for effect on gene expression after application of hundreds of over-representation, we then calculate the genes differen- drugs at various doses, drug signatures are calculated and tially expressed in disease-related experiments and deter- are then queried with other datasets for which a potential mine which chemical signatures are significantly over- therapeutic is desired. While this has proven to be an represented. We first verified the accuracy of our meth- excellent system to find chemicals that essentially reverse odology by analyzing microarray data of samples with the genome-wide effects seen in disease, the approach of known chemical exposure. After these verification stud- measuring gene expression and calculating signatures ies yielded positive results, we then applied the method to across tens of thousands of environmental chemicals is predict disease-chemical associations in breast, lung, and not always feasible or scalable. Although other data- prostate cancer datasets. We validated some of these pre- driven approaches have been described [9], few have dictions with curated disease-chemical relations, war- given insight into external causes of disease. ranting further study regarding pathogenesis and A second approach has been to use knowledge bases, biological mechanism in context of environmental expo- such as Gene Ontology [10] to aid in the interpretation of sure. Our method appears to be a promising and scalable genomic results. For example, Gene Ontology analysis of way to use existing datasets to predict environmental a cancer experiment might elucidate a molecular mecha- associations between genes and disease. nism related to an environmental chemical. Unfortu- nately, there is still a lack of methodology to derive Methods hypotheses for environmental-genetic associations in dis- Method to Predict Environmental Associations to Gene ease pathogenesis, as Gene Ontology and general gene- Expression Data set based approaches have limited information on envi- The Comparative Toxicogenomics Database (CTD) ronmental chemicals. includes manually-curated, cross-species relations In contrast to the previous approaches, we claim that between chemicals and genes,
Details
-
File Typepdf
-
Upload Time-
-
Content LanguagesEnglish
-
Upload UserAnonymous/Not logged-in
-
File Pages18 Page
-
File Size-