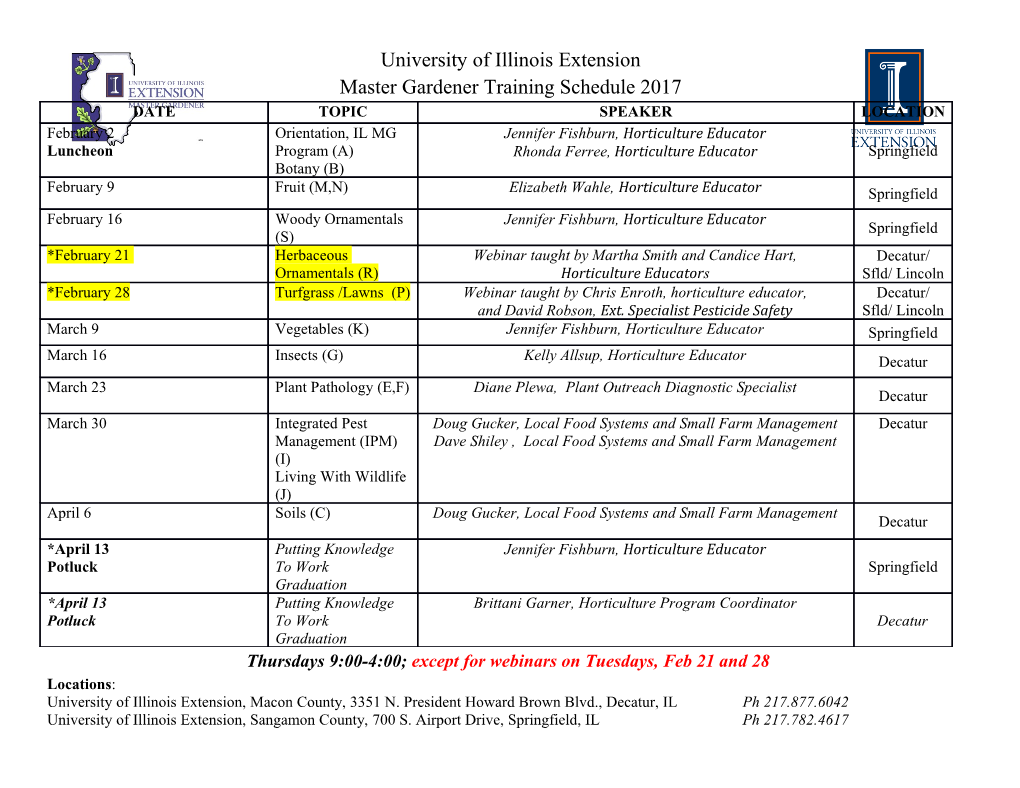
CS6640 Computational Photography 15. Matting and compositing © 2012 Steve Marschner 1 Final projects • Flexible group size • This weekend: group yourselves and send me: a one-paragraph description of your idea if you are fixed on one one-sentence descriptions of 3 ideas if you are looking for one • Next week: project proposal one-page description plan for mid-project milestone • Before thanksgiving: milestone report • December 5 (day of scheduled final exam): final presentations Cornell CS6640 Fall 2012 2 Compositing ; DigitalDomain; vfxhq.com] DigitalDomain; ; Titanic [ Cornell CS4620 Spring 2008 • Lecture 17 © 2008 Steve Marschner • Foreground and background • How we compute new image varies with position use background use foreground [Chuang et al. / Corel] / et al. [Chuang • Therefore, need to store some kind of tag to say what parts of the image are of interest Cornell CS4620 Spring 2008 • Lecture 17 © 2008 Steve Marschner • Binary image mask • First idea: store one bit per pixel – answers question “is this pixel part of the foreground?” [Chuang et al. / Corel] / et al. [Chuang – causes jaggies similar to point-sampled rasterization – same problem, same solution: intermediate values Cornell CS4620 Spring 2008 • Lecture 17 © 2008 Steve Marschner • Partial pixel coverage • The problem: pixels near boundary are not strictly foreground or background – how to represent this simply? – interpolate boundary pixels between the fg. and bg. colors Cornell CS4620 Spring 2008 • Lecture 17 © 2008 Steve Marschner • Alpha compositing • Formalized in 1984 by Porter & Duff • Store fraction of pixel covered, called α A covers area α B shows through area (1 − α) – this exactly like a spatially varying crossfade • Convenient implementation – 8 more bits makes 32 – 2 multiplies + 1 add per pixel for compositing Cornell CS4620 Spring 2008 • Lecture 17 © 2008 Steve Marschner • Alpha compositing—example [Chuang et al. / Corel] / et al. [Chuang Cornell CS4620 Spring 2008 • Lecture 17 © 2008 Steve Marschner • Creating alpha mattes • Compositing is ubiquitous in film production merge separately shot live action merge visual effects with live action merge visual effects from different studios/renderers • Also useful in photography, graphic design composite photos [wired cover] photos as non-rectangular design elements [newsweek cover] • The alpha channel can be called a “matte” (dates from matte paintings, painted on glass to allow backgrounds to show through when photographed) • Getting a matte for a photographic source is tricky and getting it right is crucial to good results leads to hours and hours of manual pixel-tweaking Cornell CS6640 Fall 2012 9 Matting • Someone has computed C = F over B and lost F and B, and we are supposed to recover F (including α) and B. The Hobbit promotional image The Hobbit promotional When you can arrange it, it’s much easier if B is some very unlikely color… Cornell CS6640 Fall 2012 10 Strategy • Simple approaches used for analog and early digital chroma- key devices ↵ =1 clamp(a (C a C )) for a blue background (bluescreen) [Smith & Blinn 1996] Formula from − 1 b − 2 g and other more complicated schemes • More principled approach: Bayesian matting based on statistical models for colors of F and B compute per-pixel statistical estimate of each pixel’s F and α Cornell CS6640 Fall 2012 11 Trimap • Someone has to specify which part is supposed to be extracted • Trimap: label pixels as definitely F, definitely B, or not sure Mishima Knockout Ruzon-Tomasi Bayesian Input [Chuang et al. 2001] (a) (b) (c) (d) gmentation Se r ΣF F _ P(F) F 12 F Cornell CS6640 Fall 2012 F α 1-αr C P(F) 1-α 1-α ΣF αr C α Σ C σC _ P(C) B C C B' C P(C) Composite α α P(B) P(B) B _ _ B B αg 1-αg g Figure 2 Summary of input images and results. Input images (top row): a blue-screen mattingΣB example of a toy lion, a syntheticΣB “n a t u r a l image” of the same(e)lion (for which the exact solution is(f)known), and two real natural images,(g) (a lighthouse and a woman).(h)Input segmentation (middle row): conservative foreground (white), conservative background (black), and “u n k n o wn” (grey). The leftmost seFigurgmentatione 1 Summarywas computedof algorithms.automaticallyEach(seeoftext),thewhilealgorithmsthe rightmostshownthreein thiswerefigurespecifirequires ed by hand.someCompositingspecifi cationresultsof(bottombackgroundrow): and foreground thepixresultsels. Mishima’of compositings algorithmthe fore(a)groundusesimagesthese andsamplesmattestoextractedform athroughglobal ourdistribBayesianution,mattingwhereasalgorithmKnockoutover(b),newRuzon-Tbackgroundomasi (c), and our scenes.new Bayesian(Lighthouseapproachimage and(d) analyzethe backgroundunknownimagespixelsin compositeusing localcourtesydistribPhiliputions.Greenspun,The darkhttp://philip.greenspun.com.gray area in (c) correspondsWomanto a segment within imagethe unknowas obtainedwn regionfromthatCorelwillKnockbe eout’valuateds tutorial,usingCoptheyrightstatistics!c 2001deriCorel.vedAllfromrightsthereservsquareed.) region’s overlap with the labeled foreground and background. Figures (e)-(h) show how matte parameters are computed using the Mishima, Knockout, Ruzon-Tomasi, and our Bayesian approach, respectively. method s fractionally interpolated covariance ΣC , as depicted in Fig- lace [12] provided an alternative solution that was indepen- ure 1(g). The optimal alpha is the one that yields an inter- dently (and much later) developed and refined by Smith and mediate distribMishima’ ution for which the observed color has maxi- Blinn [11]: take an image of the same object in front of mul- mum probability; i.e., the optimal α is chosen independently tiple known backgrounds. This approach leads to an overcon- of F and B. As a post-process, the F and B are computed strained system without building any neighborhood distribu- as weighted sums of the foreground and background cluster tions and can be solved in a least-squares framework. While approach means using the individual pairwise distribution probabilities this approach requires even more controlled studio conditions as weights. The F and B colors are then perturbed to force than the single solid background used in blue screen matting them to be endpointsBayesian of a line segment passing through the and is not immediately suitable for live-action capture, it does observed color and satisfying the compositing equation. provide a means of estimating highly accurate foreground and alpha values for real objects. We use this method to provide Both the Knockout and the Ruzon-Tomasi techniques can truth ground-truth mattes when making comparisons. be extended to video by hand-segmenting each frame, but more automatic techniques are desirable for video. Mit- sunaga et al. [6Ground ] developed the AutoKey system for extract- 3. Our Bayesian framework ing foreground and alpha mattes from video, in which a Alpha Matte Composite For the developmentInset that follows, we will assume that our user seeds a frame with foreground and background con- input image has already been segmented into three regions: tours,Figurewhich3 Blue-screenthen evmattingolve oofverliontime.(takenThisfrom leftmostapproach,columnhoweof Figurever, 2). Mishima’“background,s results in” the“foretopground,row suffer” andfrom “b“unkno l u e spill.wn,” ” with the back- makTheesmiddlestrongandsmoothnessbottom rows shoassumptionsw the Bayesianaboutresult andthegroundforegroundtruth, respectivelyground. and foreground regions having been delineated con- and background (in fact, the extracted foreground layer is as- servatively. The goal of our algorithm, then, is to solve for sumed to be constant near the silhouette) and is designed for the foreground color F , background color B, and opacity α use with fairly hard edges in the transition from foreground given the observed color C for each pixel within the unknown to background; i.e., it is not well-suited for transparency and region of the image. Since F , B, and C have three color chan- hair-like silhouettes. nels each, we have a problem with three equations and seven In each of the cases above, a single observation of a pixel unknowns. yields an underconstrained system that is solved by building Like Ruzon and Tomasi [10], we will solve the problem spatial distributions or maintaining temporal coherence. Wal- in part by building foreground and background probability refresher Estimating the matte joint distribution: p(a, b) marginal distribution (projection): p(a)= b p(a, b) conditional distribution (slice): p(a b)=p(a, b)/p(b) Bayes: p(a b)p(b)=p(a)p(b a)p(a) | R • Applying the pattern of | | MAP estimation: p(F, B, ↵ C) = p(C F, B, ↵)p(F, B, ↵) | | what we want what we have need some to maximize a model for assumptions (likelihood) (probability) here (prior) A Bayesian Approach to Digital Matting Yung-Yu Chuang1 Brian Curless1 David H. Salesin1,2 Richard Szeliski2 1Department of Computer Science and Engineering, University of Washington, Seattle, WA 98195 2Microsoft Research, Redmond, WA 98052 • Bayesian matting: E-mail: {cyy, curless, salesin}@cs.washington.edu [email protected] http://grail.cs.washington.edu/projects/digital-matting/ Abstract Other approaches attempt to pull mattes from natural (arbi- trary) backgrounds, using statistics of known regions of fore- This paper proposes a new Bayesian framework for solving ground or background in order to estimate the foreground and gaussian noise model for probability of C the matting problem, i.e. extracting a foreground element from background colors along the boundary. Once these
Details
-
File Typepdf
-
Upload Time-
-
Content LanguagesEnglish
-
Upload UserAnonymous/Not logged-in
-
File Pages28 Page
-
File Size-