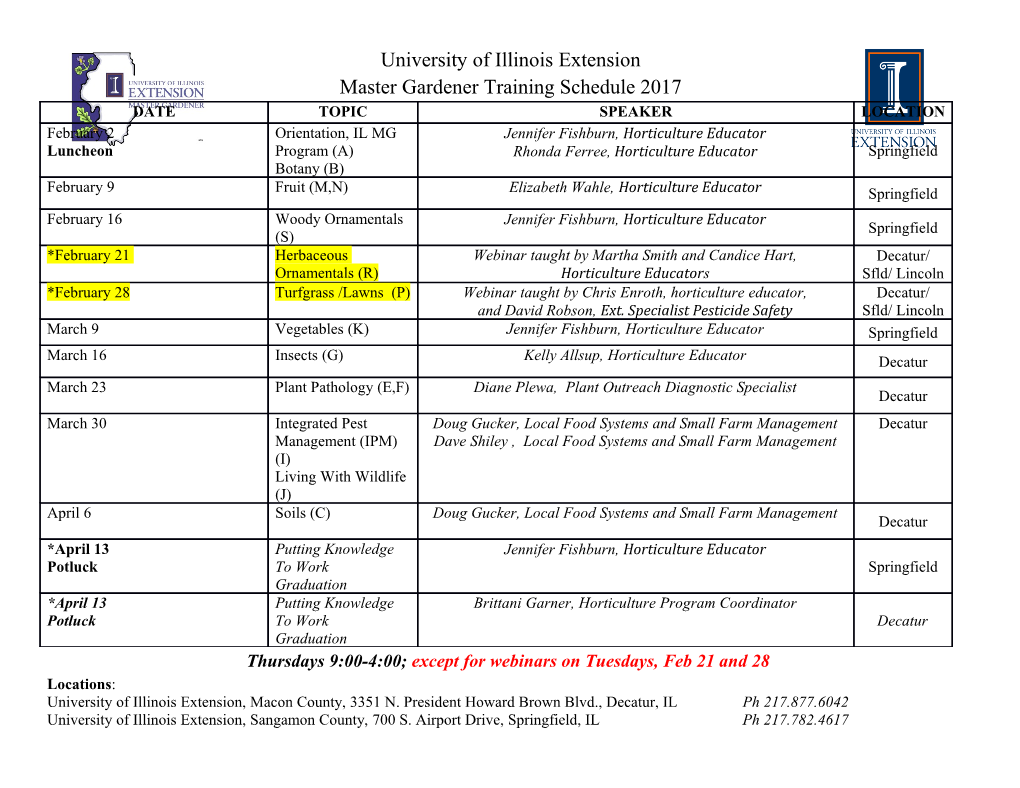
Forward Looking Expected Credit Losses, Model Risk And Uncertainty – A Foundational Approach for CECL, IFRS9 and Stress Testing Jorge R. Sobehart Credit and Obligor Risk Analytics Citi Franchise Risk Architecture CECL 2017, New York October 11-12, 2017 The analysis and conclusions set forth are those of the author only. Citigroup is not responsible for any statement or conclusion herein, and no opinions or theories presented herein necessarily reflect the position of the institution. 1 Credit Stress Tests, Model Risk And Uncertainty Key points • Creating a foundational model development framework for multiple business applications - Functional and technical soundness • Understanding model benefits and limitations, and changes in business environment 6.1.1• Analyzing[T] Diagnostic Tests Applicable t Regression the impact of credit-based Models cycles, financial crises and uncertainty on portfolio losses • Integrating expert judgment and contextual information into stress testing for loss estimation • Creating a model uncertainty framework for adjusting stress test model estimates 2 Foundational Risk Models for Loss Estimation • Conditional Stress Expected Testing Losses • CCAR/DFAST • Loans, Securities, PPNR, RWA Foundational • Conditional PD & Rating Unexpected Risk Migration, LGD, Capital Losses EAD & Utilization • Concentration Models Effects • Expected Credit Losses Reserves • Reserves • IFRS9/CECL Are the models conceptually sound for multiple applications under benign and stress conditions? 3 Portfolio Diversification Effects (In Theory….) DiversifiCation benefit: Adding independent obligors with similar exposures and credit quality reduces Increasing Diversification Increasing the uncertainty of credit losses (as a percentage of outstanding) ConCentration risk: Adding correlated obligors and/or large exposures increases the uncertainty of credit losses Ideally, diversification would reduce the portfolio loss sensitivity to specific details about PDs, EADs, LGDs correlations, spreads, etc. 4 Sensitivity To Parameter Variability (In Practice…) Idealized portfolio 10000 Idealized Portfolio Similar size exposures in well diversified sectors 1000 Thin tails Less sensitive to specific 100 Probability details on PDs, EADs, LGDs Frequency Frequency 10 Portfolio losses 1 0.1 1 10 100 1000 Exposure ($mm) Highly diversified portfolio exposures Realistic Portfolio Risk concentration to particular names or Fat tails industries Probability • Require detailed analysis Frequency for different sectors and regions Portfolio losses More sensitive to uncertainty Exposure Amount ($MM) and specific details on PDs, EADs and LGDs 5 Through-The-Cycle Risk Capital And Pro-Cyclicality Portfolio Loss Distribution Point-in-Time (or Short-Term) Risk Capital RC Typical VaR measures conditional on short history can be pro- cyclical and lead to volatile estimates today today future future Short Term History Through-The-CyCle Risk Capital Forecasts can also be added RC today future 1990 2000 2010 today future Lower volatility over Long-Term History and Stress Scenarios time (model reduces cyclical variability) Scenario uncertainty based on different economic conditions 6 Risk Capital/Stress Testing – Capturing Uncertainty Include scenario Use credit and uncertainty for economic indicators to meaningful risk estimates generate possible stress at high confidence levels scenarios beyond historical range Simulated vs. Historical Default Rates for CCC 80% Moody's 70% mean-deviation mean 60% mean+deviation S&P 50% Region 1 40% 30% Default rate Region 2 Frequency 20% 10% 0% 1980 1985 1990 1995 2000 2005 Year Correlation Spreads, PD and EAD and usage LGD Correlations credit migration Credit Losses for 1 Std. Dev. Scenarios 8 1997 7 1998 1999 6 2000 Consistent correlated 2001 5 2002 2003 credit migration scenarios 4 Frequency 3 with uncertainty 2 1 0 0 20 40 60 80 100 CreditPortfolio Losses ($MM) Loss 7 Expected Credit Loss – IFRS9 and CECL 8 Expected Cash Flows (IFRS9/CECL) ► Under IFRS9 accounting guidelines the estimation of an asset’s impairment -as determined by the Expected Credit Losses (ECL) – is a critical concept for assets under Amortized Cost and Fair Value through Other Comprehensive Income (OCI). ► More precisely, expected credit losses are probability weighted estimates of the present value of all cash shortfalls (in each period “t”) over the expected life of the financial instrument. ► Discounting is based on the EffeCtive Interest Rate (EIR). ► A cash shortfall is the difference between the cash flows that are due to an entity in accordance with the contract (contractual cash flows) and the cash flows that the entity expects to receive in practice (expected cash flows). ► Because expected credit losses consider the amount and timing of payments, a credit loss arises even if the entity expects to be paid in full but later than when contractually due. Asset life Possible losses due to credit events at different times ?@AB/@CB ��� = & ��������/ (���ℎ ����6/ − ���ℎ ����8/)×�����������/ /DE Present value discount Contractual Expected cash Probability weight for each based on EIR at “t” cash flow at “t” flow at “t” period in the scenario at “t” 9 12 Month vs. Lifetime Expected Credit Loss ► At initial recognition, an allowance (or provision in the case of commitments and guarantees) is required for ECL resulting from default events that are possible within the next 12 months (’12- month ECL’). Asset life Possible losses for credit events in the next 12 months ?@AB/@CB ���FGC/HI = & ��������/ (���ℎ ����6/ − ���ℎ ����8/)×�����������/ /DE Present value Contractual Expected cash Probability weight for each discount cash flow flow period in the scenario ► In the event of a significant deCline in credit quality (increase in credit risk), an allowance (or provision) is required for ECL resulting from all possible default events over the expected life of the financial instrument (‘lifetime ECL’). Asset life Possible losses for credit events through the life of the asset ?@AB/@CB ���?@AB/@CB = & ��������/ (���ℎ ����6/ − ���ℎ ����8/)×�����������/ /DE 10 Illustrative Example – Simple Loan/Bond Ø For a simple fixed interest rate loan/bond with principal payment WT = V0 = EAD0 at expiration T, and interest payments Ct = R V0 0 < � ≤ � with no initial interest payment at t = 0, the ECL reduces to: 1Yr Period STUV ���FNO = & �/���/���E �/ − �/RF /DE Lifetime S ���W@AB = & �/���/���E �/ − �/RF /DE Present value Loss severity Amount outstanding Incremental defaults discount at default at default in each period • That is, for this example, the ExpeCted Credit Loss is simply the cumulative default loss described by the LGDt and EAD0 at time t but discounted (present-valued) to the initial period t = 0 by the factor Dt since defaults occur at different points in time in the future and need to be discounted to the present time. 11 Understanding Functional and Technical Specifications for Models 12 Conceptual Soundness – Functional and Technical • The approach is appropriate for the business 1 purpose for whiCh it is being developed following an assessment of each of the Functional Requirements of the approach, including: – The complexity and nuance of the business Conceptual Soundness Functional for which the approach is being developed Soundness – Whether the selected approach appropriately The approach is captures the relationship between the output and business/risk drivers Conceptually Sound when – The required output and whether it is: 6.1.1 [T] Diagnostic Tests Applicable to Regressionbusiness/risk drivers reflect-based Models the specific business needs Functionally Sound, and • The modeling approach is consistent with industry Technically Sound 2 best practice based on an assessment of: – Modeling approach • Assumptions used to frame the problem Technical Soundness • Assumptions used to make it traCtable – Model data and segmentation – Model estimation – Model performance and outcomes analyses 13 Model Conceptual Soundness, Uncertainty and Model Risk Make the equation correct by adding only one short stroke 5 + 5 + 5 = 550 14 Functional and Technical Soundness Make the equation correct by adding only one short stroke 5 + 5 + 5 = 550 5 + 5 + 5 = 550 Solution fits purpose (Functionally Sound) Solution is technically correct (Technically Sound) Are there other possible solutions? 15 Fundamental and Technical Assumptions Make the equation correct by adding only one short stroke 5 + 5 + 5 = 550 5 + 5 + 5 = 550 Technical difference A Uncertainty 5 + 5 + 5 = 550 Fundamental - difference 5 + 5 + 5 = 550 Technical Model Risk B difference (Need to document all model limitations ) 5 + 5 + 5 = 550 16 Functional and Technical Specifications (1) X Y Non-Investment Grade Default Change in NIG Unempl Unempl Default Rates are Correlated to Changes in Year Rate Rate Rate 1980 7.2 1.2 1.6 Unemployment Rates 1981 8.5 1.3 0.7 1982 10.8 2.3 3.6 1983 8.3 -2.5 4.1 Model Design 1984 7.3 -1.0 3.1 Y 1985 7.0 -0.3 3.8 1986 6.6 -0.4 6.2 Y=AX+B 1987 5.7 -0.9 4.3 1988 5.3 -0.4 3.9 1989 5.4 0.1 5.9 1990 6.3 0.9 10.3 Y 1991 7.3 1.0 9.0 1992 7.4 0.1 4.9 6.1.1 [T] Diagnostic Tests Applicable t Regression- 1993 6.5 -0.9 3.4 1994 5.5 -1.0 2.1 X based ModelsRecession 1995 5.6 0.1 3.1 1996 5.4 -0.2 1.7 Y=AX+B 1997 4.7 -0.7 1.8 1998 4.4 -0.3 3.0 1999 4.0 -0.4 5.3 2000 3.9 -0.1 6.0 2001 5.7 1.8 9.5 2002 6.0 0.3 7.5 OLS 2003 5.7 -0.3 5.1 2 2004 5.4 -0.3 2.4 R 2005 4.9 -0.5 1.7 2006 4.4 -0.5 1.7 2007 5.0 0.6 0.9 Residuals 2008 7.3 2.3 5.4 2009 9.9 2.6 12.1 Lags 2010 9.4 -0.5 3.1 Stationarity X 2011 8.5 -0.9 1.9 2012 7.9 -0.6 2.7 Stability 2013 6.7 -1.2 2.6 2014 5.6 -1.1 1.9 etc.
Details
-
File Typepdf
-
Upload Time-
-
Content LanguagesEnglish
-
Upload UserAnonymous/Not logged-in
-
File Pages61 Page
-
File Size-