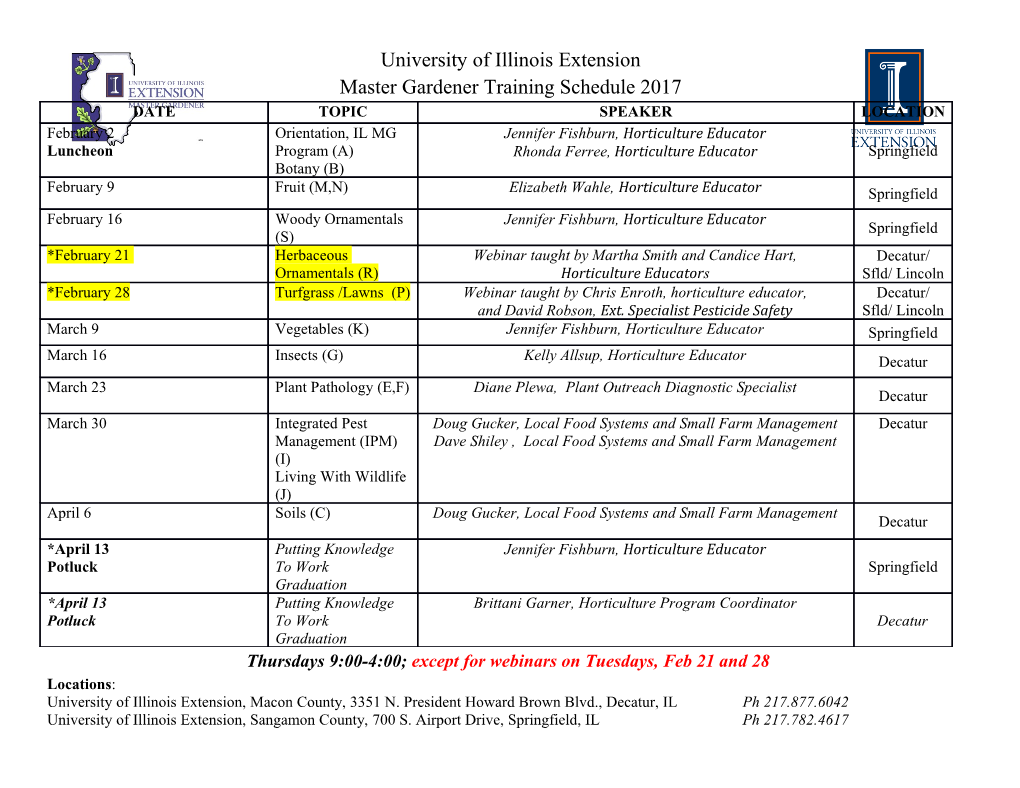
A Comparative Study of Pretrained Language Models for Automated Essay Scoring with Adversarial Inputs 1st Phakawat Wangkriangkri 2nd Chanissara Viboonlarp 3rd Attapol T. Rutherford Department of Computer Engineering Department of Computer Engineering Department of Linguistics Chulalongkorn University Chulalongkorn University Chulalongkorn University Bangkok, Thailand Bangkok, Thailand Bangkok, Thailand [email protected] [email protected] [email protected] 4th Ekapol Chuangsuwanich Department of Computer Engineering Chulalongkorn University Bangkok, Thailand [email protected] Abstract—Automated Essay Scoring (AES) is a task that for scoring essays. We experimented three popular transfer- deals with grading written essays automatically without human learning text encoders: Global Vectors for Word Repre- intervention. This study compares the performance of three sentation (GloVe) [6], Embeddings from Language Models AES models which utilize different text embedding methods, namely Global Vectors for Word Representation (GloVe), Em- (ELMo) [7], and Bidirectional Encoder Representations from beddings from Language Models (ELMo), and Bidirectional Transformers (BERT) [8]. An ideal AES system should both Encoder Representations from Transformers (BERT). We used correlate with manually-graded essay scores and be resistant two evaluation metrics: Quadratic Weighted Kappa (QWK) and to adversarial hacks; thus, we evaluated the models against a novel “robustness”, which quantifies the models’ ability to both criteria. detect adversarial essays created by modifying normal essays to cause them to be less coherent. We found that: (1) the BERT- Our contributions can be summarized as follows: based model achieved the greatest robustness, followed by the • We developed three AES-specific models utilizing dif- GloVe-based and ELMo-based models, respectively, and (2) fine- ferent transfer-learning text encoders, and compared tuning the embeddings improves QWK but lowers robustness. These findings could be informative on how to choose, and their performances using Quadratic Weighted Kappa whether to fine-tune, an appropriate model based on how (QWK) [9], which measures how well the models’ much the AES program places emphasis on proper grading predictions agree with human ratings. of adversarial essays. • We also introduced a new evaluation metric based on Keywords—Automated Essay Scoring, Embedding, Language robustness, which measures the models’ resistance to Modeling, Adversarial Input adversarial essays. A model’s QWK and robustness, considered together, are indicative of the model’s suit- I. INTRODUCTION ability for the AES task. We found that the BERT-based The ability to write coherently and fluently is imperative model is the most suitable, followed by the GloVe-based for students. Automated Essay Scoring (AES) has gained and ELMo-based models, respectively. Nevertheless, we increasing attention as it allows language learner’s writing noticed that these models did not display sufficient skills to be assessed at scale. This type of assessment presents robustness according to the evaluation metric used. a challenge in natural language processing (NLP) because • We discovered that fine-tuning the models’ pre-trained the grading criteria involves all levels of linguistic analyses. embedding weights on the dataset used in this work im- Systems can detect word-level and sentence-level errors, such proves QWK but decreases the three models’ robustness as typos and grammatical errors, at a reasonable accuracy, as a result of the models being more accustomed to poor but discourse-level errors, such as lack of coherence, focus, writing. or structure, require a more sophisticated model, and remain the crux of AES. Although recent neural-network approaches II. RELATED WORKS are proven to be effective for grading naturally written essays [1]–[3], they are prone to exploitation by adversarial AES system has been improved with new techniques input which tricks the systems to yield good scores without over the decades. The Intelligent Essay Assessor system, respecting the actual grading criteria. Several past studies developed in 1999 [10], employed Latent Semantic Analysis, explored AES systems that detect coherency [4], [5] and while the e-rater program used by Educational Testing Ser- prompt-relevancy [5] in order to rectify this problem. vice [11] utilized linear regression with feature engineering In this study, we employed models that encode para- to grade essays. In a program developed in 1998 [12], a graphs of text and capture discourse-level context required Naive Bayes model classified texts based on their content Fig. 1. Each of the three models (GloVe-based, ELMo-based, and BERT-based) are hierarchical in nature, moving up from word-level to essay-level. and writing styles. AES has also been posed as a ranking liest works to compare different text embedding methods on problem [13]. their suitability for detecting adversarial inputs, by creating More recent AES systems often incorporate neural net- and testing models that are structurally similar but based on works. Various architectures have been used; for example, three different text embeddings: BERT, ELMo and GloVe. an LSTM model [14] with embedding layer and average III. MODEL pooling [1], and a CNN model [15] with sentence embedding [2]. Reference [3] introduced attention mechanism [16] to In this section we describe our AES models. We developed the AES task and found that CNNs are better-suited for three AES models based on different embedding techniques, detecting sentence structures, while LSTMs are preferable namely GloVe-based, ELMo-based, and BERT-based models. for evaluating document-level coherence. Our models’ illustration is shown in Fig. 1. Our models all Many AES systems utilize word or context embedding. have a hierarchical nature: each computes word-level repre- Word embeddings encode knowledge of the relationships sentations, then sentence-level, then essay-level successively. between words. The technique dates back to the early The following paragraphs describe each sub-level in details. 2000’s [17] and gained popularity in 2013 [18]. One of the Note that for BERT-based model, the whole transformer prominent word embeddings is GloVe [6], which encodes the architecture is used as an end-to-end AES model as suggested ratio of co-occurrence probabilities between pairs of words. by [8]. We pass a sequence of word tokens to the pre- Word embeddings’ limitation is that a word’s representation trained BERT model [23]. We use the model named BERT- is the same in all contexts. Context embedding addresses Base, which, before connected to an output layer, has 12 this limitation by encoding text on a case-by-case basis and layers (transformer blocks) of hidden size of 768 and has 12 self-attention heads. The implementation of our models giving a unique representation to each text. Examples of 1 context embeddings are ELMo [7], which encodes texts using is available on Github . two unidirectional LSTM models, and BERT [8], which A. Word-level encodes texts using a bidirectional transformer model [19]. In each model, embeddings are initialized with pre-trained For the GloVe-based model, word tokens weights, then are either fixed while training the main system [w1; w2; w3; :::; wn] of each sentence s are passed into or updated during training. a GloVe pre-trained lookup layer to acquire word vectors [w ; w ; w ; :::; w ]. These word vectors are fed into a Due to the large number of parameters involved in AES 1 2 3 n convolutional layer followed by attention-pooling to acquire systems, the exact criteria for grading essays is often obscure. the sentence representation s. System fragilities have been exposed by tricking them with For the ELMo-based model, as described by [7], word incoherent essays that serve as adversarial inputs. Adversarial tokens w are passed into convolutional layers followed examples include well-written paragraphs repeated many i by highway layers to acquire the intermediate word rep- times [20] or a set of well-written sentences randomly per- resentations x . These representations are passed into the muted [4]. Systems have been developed to detect adversarial i main two-layer language model in both directions known as essays, and each system usually employs a single text embed- Bidirectional Language Model (BiLM) to generate a hidden ding. Reference [4] used Senna word embedding [21], [22] forw state h for forward direction and hback for backward di- in their model of local coherence, while [5] preferred BERT i i rection. The x are then weight-summed with the two hidden context embedding for their system of two-stage learning i states to acquire the context-sensitive word embedding w . framework. i To the best of our knowledge, our work is one of the ear- 1https://github.com/sunnypwang/aes Fixed mean-pooling across all words determines the sentence TABLE I representation s. We used the pre-trained BiLM module from ASAP DATASET DESCRIPTION Tensorflow Hub, which is trained on the 1 Billion Word prompt #essays avg sentences score mode Benchmark 2. 1 1783 23 2-12 8 B. Sentence-level 2 1800 20 1-6 4 3 1726 6 0-3 2 4 1772 5 0-3 1 Sequences of sentence vectors [s1,s2,s3,...,sn] are passed 5 1805 6 0-4 2 into a single-layer forward LSTM with attention-pooling to 6 1800 7 0-4 3 acquire the final representation. Each sentence vector si is 7 1569 11 0-30 16 passed through an LSTM unit to generate a hidden state 8 723 34 0-60 40 hi. The hidden states from every time step are then passed through the self-attention layer in the method described by [3]. The attention mechanism helps to indicate how each A. Dataset sentence contributes to the final score. A sentence with high We used the Automated Student Assessment Prize (ASAP) attention weight has more responsibility in determining the dataset from the Hewlett Foundation, available on Kaggle3. final score than a sentence with low attention weight. This dataset consists of essays written by students in grades The attention weights are calculated as follows: Let H be seven to ten in 8 different prompts, as described in Table I.
Details
-
File Typepdf
-
Upload Time-
-
Content LanguagesEnglish
-
Upload UserAnonymous/Not logged-in
-
File Pages6 Page
-
File Size-