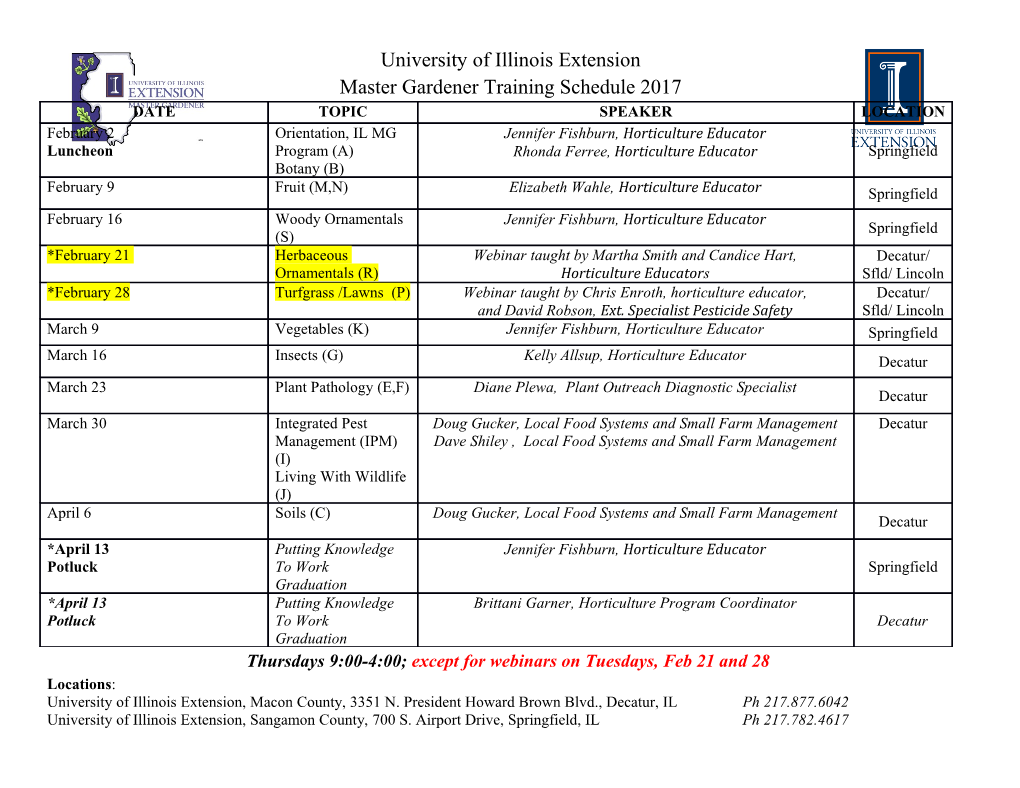
AP Statistics Chapter 7 Linear Regression Objectives: • Linear model • Predicted value • Residuals • Least squares • Regression to the mean • Regression line • Line of best fit • Slope intercept • se 2 • R 2 Fat Versus Protein: An Example • The following is a scatterplot of total fat versus protein for 30 items on the Burger King menu: The Linear Model • The correlation in this example is 0.83. It says “There seems to be a linear association between these two variables,” but it doesn’t tell what that association is. • We can say more about the linear relationship between two quantitative variables with a model. • A model simplifies reality to help us understand underlying patterns and relationships. The Linear Model • The linear model is just an equation of a straight line through the data. – The points in the scatterplot don’t all line up, but a straight line can summarize the general pattern with only a couple of parameters. – The linear model can help us understand how the values are associated. The Linear Model • Unlike correlation, the linear model requires that there be an explanatory variable and a response variable. • Linear Model 1. A line that describes how a response variable y changes as an explanatory variable x changes. 2. Used to predict the value of y for a given value of x. 3. Linear model of the form: 6 The Linear Model • The model won’t be perfect, regardless of the line we draw. • Some points will be above the line and some will be below. • The estimate made from a model is the predicted value (denoted as yˆ ). The Linear Model • The predicted value: y • Putting a hat on y is standard statistics notation to indicate that something has been predicted by a model. Whenever you see a hat over a variable name or symbol, you can assume it is the predicted version of that variable or symbol. 8 Example: Linear Model Observed Values Predicted Values 9 The Linear Model • The linear model will not pass exactly through all the points, but should be as close as possible. • A good linear model makes the vertical distances between the observed points and the predicted points (the error) as small as possible. • This “error” doesn’t mean it’s a mistake. Statisticians often refer to variability not explained by the model as error. 10 The Linear Model Predicted value ŷ (y-hat) Observed value y Error = observed – predicted (y – ŷ) Residuals • This “error”, the difference between the observed value and its associated predicted value is called the residual. • To find the residuals, we always subtract the predicted value from the observed one: residual observed predicted y yˆ Residuals • Symbol for residual is: e • Why e for residual? • Because r is already taken. No, the e stands for “error.” • So, e y y Residuals • A negative residual means the predicted value’s too big (an overestimate). • A positive residual means the predicted value’s too small (an underestimate). • In the figure, the estimated fat of the BK Broiler chicken sandwich is 36 g, while the true value of fat is 25 g, so the residual is –11 g of fat. “Best Fit” Means Least Squares • Some residuals are positive, others are negative, and, on average, they cancel each other out. • So, we can’t assess how well the line fits by adding up all the residuals. • Similar to what we did with deviations, we square the residuals and add the squares. • The smaller the sum, the better the fit. • The line of best fit is the line for which the sum of the squared residuals is smallest, the least squares line. Least – Squares Regression Line (LSRL) • The LSRL is the line that minimizes the sum of the squared residuals between the observed and predicted y values (y – ŷ). 16 Correlation and the Line • What we know about correlation from chapter 7 can lead us to the equation of the linear model. • Start with a scatterplot of standardized values. Original scatterplot - fat Standardized scatterplot – versus protein for 30 items on zy (standardized fat) vs zx the Burger King menu. (standardized protein). Correlation and the Line • What line would you choose to model the relationship of standardized values? • Start at the center of the line. If an item has average protein x , should it have average fat y ? • Yes, so the line should pass through the point xy , . This is the first property of the line we are looking for, it must always pass through the point . • In the plot of z-scores, the point is the origin and then the line passes through the origin (0, 0). Correlation and the Line • The equation for a line that passes through the origin is y = mx. • So the equation on our standardized plot will be zy mzx . • We use z y to indicate that the point on the line is the predicted value , not the actual value zy. Correlation and the Line • Many lines with different slopes pass through the origin. Which one best fits our data? That is, which slope determines the line that minimizes the sum of the squared residuals? • It turns out that the best choice for slope is the correlation coefficient r. • So, the equation of the linear model is zy rzx. Correlation and the Line • • What does this tell us? • Moving one standard deviation away from the mean in x moves us r standard deviations away from the mean in y. • Put generally, moving any number of standard deviations away from the mean in x moves us r times that number of standard deviations away from the mean inz yy. rzx. How Big Can Predicted Values Get? • • r cannot be bigger than 1 (in absolute value), so each predicted y tends to be closer to its mean (in standard deviations) than its corresponding x was. • This property of the linear model is called regression to the mean; the line is called the regression line. zy rzx. The Term Regression • Sir Francis Galton related the heights of sons to the heights of their fathers with a regression line. The slope of the line was less than one. • That is, sons of tall fathers were tall, but not as much above the mean height as their fathers had been above their mean. Sons of short fathers were short, but generally not as far from their mean as their fathers. • Galton interpreted the slope correctly as indicating a “regression” toward the mean height. And regression stuck as a description of the method he used to find the line. The Regression Line in Real Units • Remember from Algebra that a straight line can be written as: y mx b • In Statistics we use a slightly different notation: yˆ b0 b1x • We write to emphasize that the points that satisfy this equation are just our predicted values, not the actual data values. • This model says that our predictions fromyˆ our model follow a straight line. • If the model is a good one, the data values will scatter closely around it. The Regression Line in Real Units • We write b1 and b0 for the slope and intercept of the line. • b1 is the slope, which tells us how rapidly changes with respect to x. • b0 is the y-intercept, which tells where the line crosses (intercepts) the y-axis. yˆ The Regression Line in Real Units • In our model, we have a slope (b1): – The slope is built from the correlation and the standard deviations: sy b1 r sx – Our slope is always in units of y per unit of x. The Regression Line in Real Units • In our model, we also have an intercept (b0). – The intercept is built from the means and the slope: b0 y b1x – Our intercept is always in units of y. Example: Fat Versus Protein • The regression line for the Burger King data fits the data well: – The equation is yx6.8 0.97 The predicted fat content for a BK Broiler chicken sandwich (with 30 g of protein) is 6.8 + 0.97(30) = 35.9 grams of fat. Calculate Regression Line by Hand • First calculate the following for the data; 1. The means 2. The standard deviations 3. The correlation r • Then the LSRL is 1. Slope 2. y- - intercept Calculate Regression Line on TI-83/84 • Enter the data into lists: explanatory variable L1 and response L2 • STAT/CALC/LinReg(a+bx)/L1,L2,VARS/Y- VARS/FUNCTION/Y1 • Your display on the screen shows LinReg(a+bx)L1,L2,Y1. – This creates the LSRL and stores it as function Y1. – The LSRL will now overlay your scatterplot. 30 Graphing the LSRL by Hand • The equation of the LSRL makes prediction easy. Just substitute an x-value into the equation and calculate the corresponding y- value. • Use the equation to calculate two points on the line. One at each end of the line (ie. low x- value and high x-value). • Plot the two points and draw a line. Example: Calculate the LSRL by hand and on the calculator (r = -.64). LSRL by Hand • Calculate the slope – From 2-VAR Stats – • Calculate the y-intercept – From 2-VAR Stats – LSRL by Hand - Continued • Then the LRSL is; – • Or in the context of the problem – By Calculator • STAT/CALC/LinReg(a+bx)/L1,L2,VARS/Y- VARS/FUNCTION/Y1 – y=a+bx – a=109.8738406 – b=-1.126988915 – r2=.4099712614 – r=-.6402899823 • • or 35 Your Turn: • Calculate the linear model by hand using r=.894. • Solution: y .0127 .018 x or BAC .0127 .018 Beers Your Turn: Year Powerboat Reg. Manatees (1000s) Killed • Calculate and graph 1977 447 13 the linear model 1978 460 21 1979 481 24 using the Ti-83/84.
Details
-
File Typepdf
-
Upload Time-
-
Content LanguagesEnglish
-
Upload UserAnonymous/Not logged-in
-
File Pages106 Page
-
File Size-