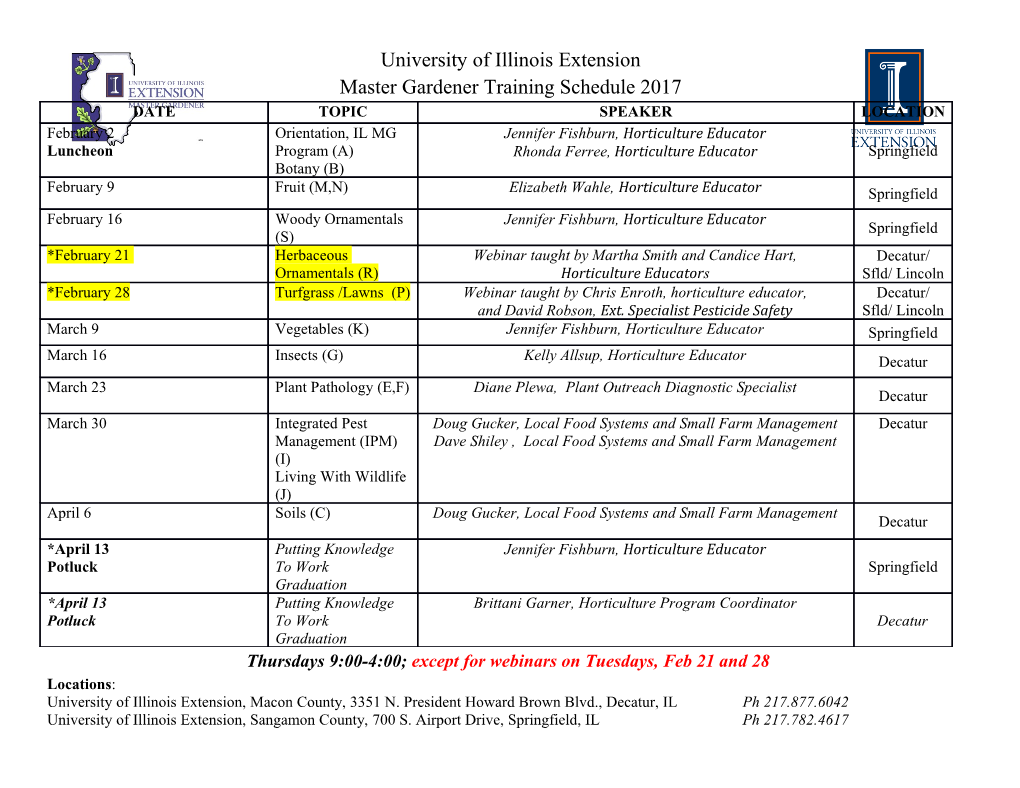
Data Processing with Apache Beam (incubating) and Google Cloud Dataflow Jelena Pjesivac-Grbovic Staff software engineer Cloud Big Data In collaboration with Frances Perry, Tayler Akidau, and Dataflow team XLDB’16 - May 2016 Agenda 1 Infinite, Out-of-Order Data Sets 2 What, Where, When, How 3 Apache Beam (incubating) 4 Google Cloud Dataflow 1 Infinite, Out-of-Order Data Sets Data... ...can be big... ...really, really big... Thursday Wednesday Tuesday … maybe infinitely big... 8:00 9:00 10:00 11:00 12:00 13:00 14:00 … with unknown delays. 8:00 8:00 8:00 8:00 9:00 10:00 11:00 12:00 13:00 14:00 Element-wise transformations Processing 8:00 9:00 10:00 11:00 12:00 13:00 14:00 Time Aggregating via Processing-Time Windows Processing 8:00 9:00 10:00 11:00 12:00 13:00 14:00 Time Aggregating via Event-Time Windows Input Processing Time 10:00 11:00 12:00 13:00 14:00 15:00 Output Event Time 10:00 11:00 12:00 13:00 14:00 15:00 Formalizing Event-Time Skew Skew Reality Ideal Processing Time Event Time Formalizing Event-Time Skew Watermarks describe event time progress. Skew "No timestamp earlier than the ~Watermark watermark will be seen" Ideal Often heuristic-based. Processing Time Too Slow? Results are delayed. Too Fast? Some data is late. Event Time 2 What, Where, When, How What are you computing? Where in event time? When in processing time? How do refinements relate? What are you computing? Element-Wise Aggregating Composite What Where When How What: Computing Integer Sums // Collection of raw log lines PCollection<String> raw = IO.read(...); // Element-wise transformation into team/score pairs PCollection<KV<String, Integer>> input = raw.apply(ParDo.of(new ParseFn()); // Composite transformation containing an aggregation PCollection<KV<String, Integer>> scores = input.apply(Sum.integersPerKey()); What Where When How What: Computing Integer Sums What Where When How What: Computing Integer Sums What Where When How Where in event time? Windowing divides data into event-time-based finite chunks. Fixed Sliding Sessions 1 1 2 3 4 2 3 1 3 4 Key 1 Key 2 Key 3 4 5 2 Time Often required when doing aggregations over unbounded data. What Where When How Where: Fixed 2-minute Windows PCollection<KV<String, Integer>> scores = input .apply(Window.into(FixedWindows.of(Minutes(2))) .apply(Sum.integersPerKey()); What Where When How Where: Fixed 2-minute Windows What Where When How When in processing time? • Triggers control Skew when results are emitted. ~Watermark Ideal • Triggers are often Processing Time relative to the watermark. Event Time What Where When How When: Triggering at the Watermark PCollection<KV<String, Integer>> scores = input .apply(Window.into(FixedWindows.of(Minutes(2)) .triggering(AtWatermark())) .apply(Sum.integersPerKey()); What Where When How When: Triggering at the Watermark What Where When How When: Early and Late Firings PCollection<KV<String, Integer>> scores = input .apply(Window.into(FixedWindows.of(Minutes(2)) .triggering(AtWatermark() .withEarlyFirings(AtPeriod(Minutes(1))) .withLateFirings(AtCount(1)))) .apply(Sum.integersPerKey()); What Where When How When: Early and Late Firings What Where When How How do refinements relate? • How should multiple outputs per window accumulate? • Appropriate choice depends on consumer. Firing Elements Discarding Accumulating Acc. & Retracting Speculative [3] 3 3 3 Watermark [5, 1] 6 9 9, -3 Late [2] 2 11 11, -9 Last Observed 2 11 11 Total Observed 11 23 11 (Accumulating & Retracting not yet implemented.) What Where When How How: Add Newest, Remove Previous What Where When How What / Where / When / How 1.Classic Batch 2. Batch with Fixed 3. Streaming Windows 4. Streaming with 5. Streaming With 6. Sessions Speculative + Late Data Retractions What Where When How 3 Apache Beam (incubating) The Evolution of Beam Colossus BigTable PubSub Dremel Google Cloud Dataflow Spanner Megastore Millwheel Flume MapReduce Apache Beam What is Part of Apache Beam? 1. The Beam Model: What / Where / When / How 2. SDKs for writing Beam pipelines -- starting with Java 3. Runners for Existing Distributed Processing Backends • Apache Flink (thanks to data Artisans) • Apache Spark (thanks to Cloudera) • Google Cloud Dataflow (fully managed service) • Local (in-process) runner for testing Apache Beam Technical Vision 1. End users: who want to write Other Beam Beam Java pipelines or transform libraries in Languages Python a language that’s familiar. 2. SDK writers: who want to make Beam Model: Pipeline Construction Beam concepts available in new languages. Cloud Runner A Runner B 3. Runner writers: who have a Dataflow distributed processing environment and want to support Beam Model: Fn Runners Beam pipelines Execution Execution Execution Growing the Beam Community Collaborate - Beam is becoming a community- driven effort with participation from many organizations and contributors Grow - We want to grow the Beam ecosystem and community with active, open involvement so Beam is a part of the larger OSS ecosystem 4 Google Cloud Dataflow Google Cloud Dataflow • Fully managed service for running Beam pipelines • Dynamically provisioned, on-demand resources • VMs, temporary storage • No tuning required • Autoscaling + Dynamic Work Rebalancing • Built from the experience with Google internal products No Tuning Required: Dynamic Work Rebalancing • Advanced straggler mitigation technique • Ensures all tasks finish at the same time Without DWR With DWR Workers Workers Time Time • For more info google: “No shard left behind: dynamic work rebalancing in Google Cloud Dataflow” No Tuning Required: Autoscaling • Dynamically adjust to the number of workers to match the load • Both for streaming and batch Workers Time Time • For more info google: “Comparing Cloud Dataflow autoscaling to Spark and Hadoop” Integrations • Apache Beam connectors • Google Cloud • Storage, BigQuery, BigTable, Datastore, Pub/Sub, • External / Custom IO • Kafka, HDFS, many in flight • Part of Google Cloud Platform • Monitoring UI • Cloud Logging • Cloud Debugger and Profiler • Stackdriver integration Big Data in Google Cloud Platform • BigQuery • A fast, economical, and fully managed data warehouse solution • Dataflow • Fully managed, real-time, data processing service for batch and streaming • Dataproc • Fast, easy to use managed Spark and Hadoop service • Datalab(beta) • Interactive large scale data analysis, exploration and visualization • Pub/Sub • Reliable, many-to-many, asynchronous messaging service • Genomics • Empowers scientists to organize world’s genomics information Machine Learning in Google Cloud Platform • Machine Learning Platform(alpha) • Fast, large scale, easy to use Machine Learning service • Vision API • Enables insights based on our powerful Vision APIs • Speech API • Speech to text conversion powered by Machine Learning • Translate API • Enables multilingual apps and programmatic translation Learn More! Follow @GCPBigData + @ApacheBeam Apache Beam (incubating) http://beam.incubator.apache.org Google Cloud Dataflow http://cloud.google.com/dataflow Google Cloud Platform http://cloud.google.com Thank you! .
Details
-
File Typepdf
-
Upload Time-
-
Content LanguagesEnglish
-
Upload UserAnonymous/Not logged-in
-
File Pages44 Page
-
File Size-