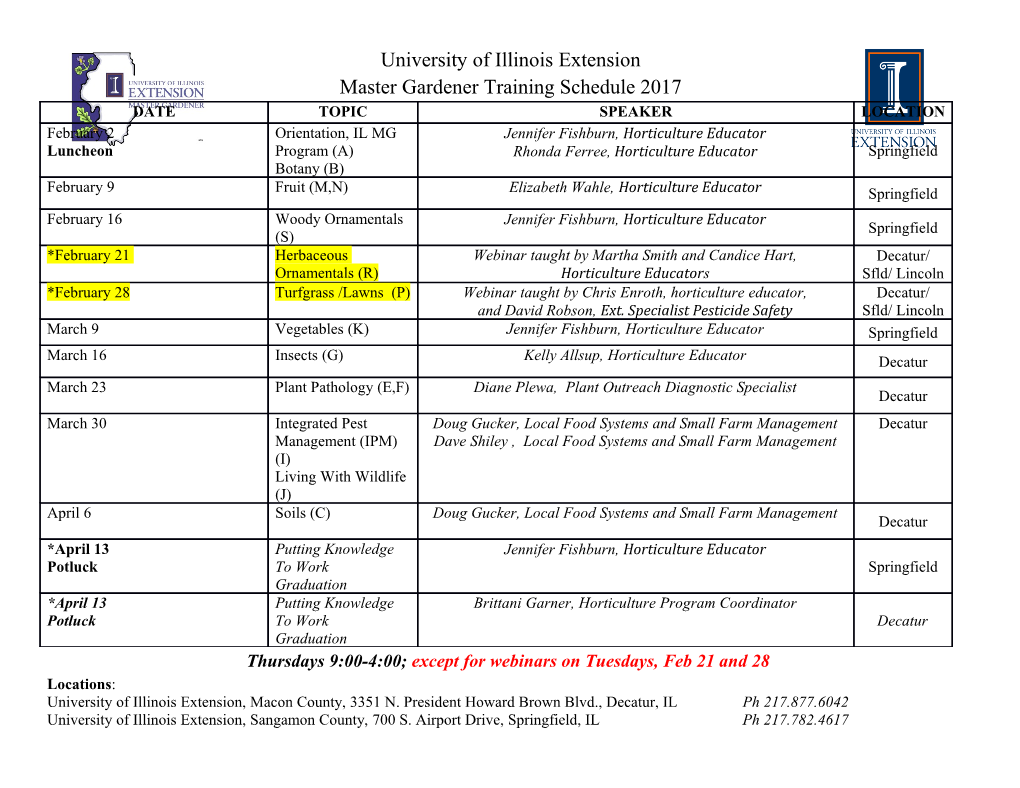
Density and Distribution Estimation Nathaniel E. Helwig Assistant Professor of Psychology and Statistics University of Minnesota (Twin Cities) Updated 04-Jan-2017 Nathaniel E. Helwig (U of Minnesota) Density and Distribution Estimation Updated 04-Jan-2017 : Slide 1 Copyright Copyright c 2017 by Nathaniel E. Helwig Nathaniel E. Helwig (U of Minnesota) Density and Distribution Estimation Updated 04-Jan-2017 : Slide 2 Outline of Notes 1) PDFs and CDFs 3) Histogram Estimates Overview Overview Estimation problem Bins & breaks 2) Empirical CDFs 4) Kernel Density Estimation Overview KDE basics Examples Bandwidth selection Nathaniel E. Helwig (U of Minnesota) Density and Distribution Estimation Updated 04-Jan-2017 : Slide 3 PDFs and CDFs PDFs and CDFs Nathaniel E. Helwig (U of Minnesota) Density and Distribution Estimation Updated 04-Jan-2017 : Slide 4 PDFs and CDFs Overview Density Functions Suppose we have some variable X ∼ f (x) where f (x) is the probability density function (pdf) of X. Note that we have two requirements on f (x): f (x) ≥ 0 for all x 2 X , where X is the domain of X R X f (x)dx = 1 Example: normal distribution pdf has the form 2 1 − (x−µ) f (x) = p e 2σ2 σ 2π + which is well-defined for all x; µ 2 R and σ 2 R . Nathaniel E. Helwig (U of Minnesota) Density and Distribution Estimation Updated 04-Jan-2017 : Slide 5 PDFs and CDFs Overview Standard Normal Distribution If X ∼ N(0; 1), then X follows a standard normal distribution: 1 2 f (x) = p e−x =2 (1) 2π 0.4 ) x ( 0.2 f 0.0 −4 −2 0 2 4 x Nathaniel E. Helwig (U of Minnesota) Density and Distribution Estimation Updated 04-Jan-2017 : Slide 6 PDFs and CDFs Overview Probabilities and Distribution Functions (revisited) Probabilities relate to the area under the pdf: Z b P(a ≤ X ≤ b) = f (x)dx a (2) = F(b) − F(a) where Z x F(x) = f (u)du (3) −∞ is the cumulative distribution function (cdf). Note: F(x) = P(X ≤ x) =) 0 ≤ F(x) ≤ 1 Nathaniel E. Helwig (U of Minnesota) Density and Distribution Estimation Updated 04-Jan-2017 : Slide 7 PDFs and CDFs Estimation Problem Problem of Interest n We want to estimate f (x) or F(x) from a sample of data fxi gi=1. We will discuss three different approaches: Empirical cumulative distribution functions (ecdf) Histogram estimates Kernel density estimates Nathaniel E. Helwig (U of Minnesota) Density and Distribution Estimation Updated 04-Jan-2017 : Slide 8 Empirical Cumulative Distribution Function Empirical Cumulative Distribution Function Nathaniel E. Helwig (U of Minnesota) Density and Distribution Estimation Updated 04-Jan-2017 : Slide 9 Empirical Cumulative Distribution Function Overview Empirical Cumulative Distribution Function 0 iid Suppose x = (x1;:::; xn) with xi ∼ F(x) for i 2 f1;:::; ng, and we want to estimate the cdf F. ^ The empirical cumulative distribution function (ecdf) Fn is defined as 1 F^ (x) = P^(X ≤ x) = Pn I n n i=1 fxi ≤xg where 1 if x ≤ x I = i fxi ≤xg 0 otherwise denotes an indicator function. Nathaniel E. Helwig (U of Minnesota) Density and Distribution Estimation Updated 04-Jan-2017 : Slide 10 Empirical Cumulative Distribution Function Overview Some Properties of ECDFs The ecdf assigns probability 1=n to each value xi , which implies that 1 P^ (A) = Pn I n n i=1 fxi 2Ag for any set A in the sample space of X. For any fixed value x, we have that ^ E[Fn(x)] = F(x) ^ 1 V [Fn(x)] = n F(x)[1 − F(x)] As n ! 1 we have that ^ as sup jFn(x) − F(x)j ! 0 x2R which is the Glivenko-Cantelli theorem. Nathaniel E. Helwig (U of Minnesota) Density and Distribution Estimation Updated 04-Jan-2017 : Slide 11 Empirical Cumulative Distribution Function Examples ECDF: Example 1 (Uniform Distribution) > set.seed(1) ecdf(x) > x = runif(20) ● 1.0 ● > xseq = seq(0,1,length=100) ● ● ● > Fhat = ecdf(x) 0.8 ● ● > plot(Fhat) ● ● 0.6 ● > lines(xseq,punif(xseq), ● Fn(x) ● ● 0.4 + col="blue",lwd=2) ● ● ● ● 0.2 ● ● ● 0.0 0.0 0.2 0.4 0.6 0.8 1.0 x ^ Note that Fn is a step-function estimate of F (with steps at xi values). Nathaniel E. Helwig (U of Minnesota) Density and Distribution Estimation Updated 04-Jan-2017 : Slide 12 Empirical Cumulative Distribution Function Examples ECDF: Example 2 (Normal Distribution) > set.seed(1) ecdf(x) > x = rnorm(20) ● 1.0 ● > xseq = seq(-3,3,length=100) ● ● ● > Fhat = ecdf(x) 0.8 ● ● > plot(Fhat) ● ● 0.6 ● > lines(xseq,pnorm(xseq), ● Fn(x) ● ● 0.4 + col="blue",lwd=2) ● ● ● ● 0.2 ● ● ● 0.0 −2 −1 0 1 2 x ^ Note that Fn is a step-function estimate of F (with steps at xi values). Nathaniel E. Helwig (U of Minnesota) Density and Distribution Estimation Updated 04-Jan-2017 : Slide 13 Empirical Cumulative Distribution Function Examples ECDF: Example 3 (Bivariate Distribution) Table 3.1 An Introduction to the Bootstrap (Efron & Tibshirani, 1993). School LSAT (y) GPA (z) School LSAT (y) GPA (z) 1 576 3.39 9 651 3.36 2 635 3.30 10 605 3.13 3 558 2.81 11 653 3.12 4 578 3.03 12 575 2.74 5 666 3.44 13 545 2.76 6 580 3.07 14 572 2.88 7 555 3.00 15 594 2.96 8 661 3.43 Defining A = f(y; z): 0 < y < 600; 0 < z < 3:00g, we have ^ P15 P15(A) = (1=15) i=1 If(yi ;zi )2Ag = 5=15 Nathaniel E. Helwig (U of Minnesota) Density and Distribution Estimation Updated 04-Jan-2017 : Slide 14 Histogram Estimates Histogram Estimates Nathaniel E. Helwig (U of Minnesota) Density and Distribution Estimation Updated 04-Jan-2017 : Slide 15 Histogram Estimates Overview Histogram Definition If f (x) is smooth, we have that P(x − h=2 < X < x + h=2) = F(x + h=2) − F(x − h=2) Z x+h=2 = f (z)dz ≈ hf (x) x−h=2 where h > 0 is a small (positive) scalar called the bin width. If F(x) were known, we could estimate f (x) using F(x + h=2) − F(x − h=2) ^f (x) = h but this isn’t practical (b/c if we know F we don’t need to estimate f ). Nathaniel E. Helwig (U of Minnesota) Density and Distribution Estimation Updated 04-Jan-2017 : Slide 16 Histogram Estimates Overview Histogram Definition (continued) If F(x) is unknown we could estimate f (x) using Pn F^ (x + h=2) − F^ (x − h=2) If 2( − = ; + = ]g ^f (x) = n n = i=1 xi x h 2 x h 2 n h nh which uses previous formula with the ECDF in place of the CDF. More generally, we could estimate f (x) using Pn Ifx 2I g n ^f (x) = i=1 i j = j n nh nh m for all x 2 Ij = (cj − h=2; cj + h=2] where fcj gj=1 are chosen constants. Nathaniel E. Helwig (U of Minnesota) Density and Distribution Estimation Updated 04-Jan-2017 : Slide 17 Histogram Estimates Bins and Breaks Histogram Bins/Breaks Different choices for m and h will produce different estimates of f (x). Freedman and Diaconis (1981) method: Set h = 2(IQR)n−1=3 where IQR = interquartile range Then divide range of data by h to determine m Sturges (1929) method (default in R’s hist function): Set m = dlog2(n) + 1e where dxe denotes ceiling function May oversmooth for non-normal data (i.e., use too few bins) Nathaniel E. Helwig (U of Minnesota) Density and Distribution Estimation Updated 04-Jan-2017 : Slide 18 Histogram Estimates Bins and Breaks Histogram: Example 1 > par(mfrow=c(1,3)) > set.seed(1) > x = runif(20) > hist(x,main="Sturges") > hist(x,breaks="FD",main="FD") > hist(x,breaks="scott",main="scott") Sturges FD scott 6 10 10 5 8 8 4 6 6 3 Frequency Frequency Frequency 4 4 2 2 2 1 0 0 0 0.0 0.2 0.4 0.6 0.8 1.0 0.0 0.2 0.4 0.6 0.8 1.0 0.0 0.2 0.4 0.6 0.8 1.0 x x x Nathaniel E. Helwig (U of Minnesota) Density and Distribution Estimation Updated 04-Jan-2017 : Slide 19 Histogram Estimates Bins and Breaks Histogram: Example 2 > par(mfrow=c(1,3)) > set.seed(1) > x = rnorm(20) > hist(x,main="Sturges") > hist(x,breaks="FD",main="FD") > hist(x,breaks="scott",main="scott") Sturges FD scott 5 8 8 4 6 6 3 4 4 Frequency Frequency Frequency 2 2 2 1 0 0 0 −2 −1 0 1 2 −3 −2 −1 0 1 2 −3 −2 −1 0 1 2 x x x Nathaniel E. Helwig (U of Minnesota) Density and Distribution Estimation Updated 04-Jan-2017 : Slide 20 Kernel Density Estimation Kernel Density Estimation Nathaniel E. Helwig (U of Minnesota) Density and Distribution Estimation Updated 04-Jan-2017 : Slide 21 Kernel Density Estimation KDE Basics Kernel Function: Definition A kernel function K is a function such that. K (x) ≥ 0 for all −∞ < x < 1 K (−x) = K (x) R 1 −∞ K (x)dx = 1 In other words, K is a non-negative function that is symmetric around 0 and integrates to 1. Nathaniel E. Helwig (U of Minnesota) Density and Distribution Estimation Updated 04-Jan-2017 : Slide 22 Kernel Density Estimation KDE Basics Kernel Function: Examples A simple example is the uniform (or box) kernel: 1 if − 1=2 ≤ x < 1=2 K (x) = 0 otherwise Another popular kernel function is the Normal kernel (pdf) with µ = 0 and σ fixed at some constant: 2 1 − x K (x) = p e 2σ2 σ 2π We could also use a triangular kernel function: K (x) = 1 − jxj Nathaniel E.
Details
-
File Typepdf
-
Upload Time-
-
Content LanguagesEnglish
-
Upload UserAnonymous/Not logged-in
-
File Pages43 Page
-
File Size-