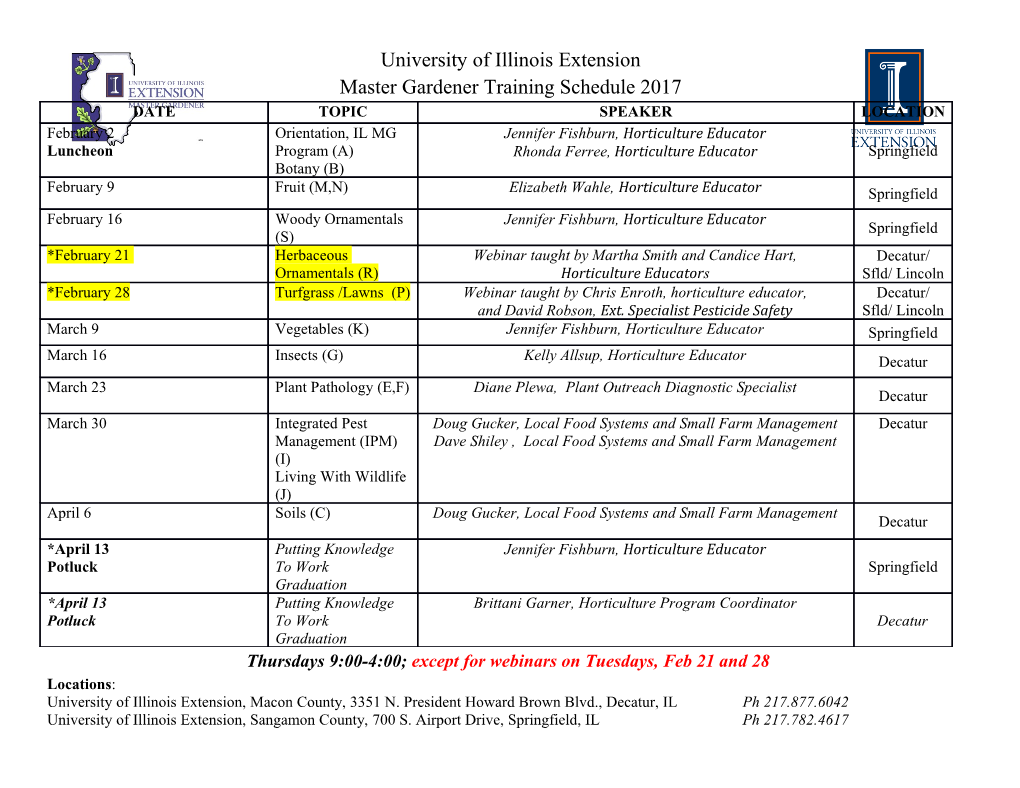
Computing Science (CMPUT) 455 Search, Knowledge, and Simulations James Wright Department of Computing Science University of Alberta [email protected] Winter 2021 1 455 Today - Lecture 22 • AlphaGo - overview and early versions • Coursework • Work on Assignment 4 • Reading: AlphaGo Zero paper 2 AlphaGo Introduction • High-level overview • History of DeepMind and AlphaGo • AlphaGo components and versions • Performance measurements • Games against humans • Impact, limitations, other applications, future 3 About DeepMind • Founded 2010 as a startup company • Bought by Google in 2014 • Based in London, UK, Edmonton (from 2017), Montreal, Paris • Expertise in Reinforcement Learning, deep learning and search 4 DeepMind and AlphaGo • A DeepMind team developed AlphaGo 2014-17 • Result: Massive advance in playing strength of Go programs • Before AlphaGo: programs about 3 levels below best humans • AlphaGo/Alpha Zero: far surpassed human skill in Go • Now: AlphaGo is retired • Now: Many other super-strong programs, including open source Image source: • https://www.nature.com All are based on AlphaGo, Alpha Zero ideas 5 DeepMind and UAlberta • UAlberta has deep connections • Faculty who work part-time or on leave at DeepMind • Rich Sutton, Michael Bowling, Patrick Pilarski, Csaba Szepesvari (all part time) • Many of our former students and postdocs work at DeepMind • David Silver - UofA PhD, designer of AlphaGo, lead of the DeepMind RL and AlphaGo teams • Aja Huang - UofA postdoc, main AlphaGo programmer • Many from the computer Poker group • Some are starting to come back to Canada (DeepMind Edmonton and Montreal) 6 State of Computer Go before AlphaGo Summary of previous work, and of our course so far • Search - MCTS, quite strong • Simulations - OK, hard to improve • Knowledge • Good for move selection • Considered hopeless for position evaluation • Strength: about 3 handicap levels below best humans • Quick progress considered unlikely - see next slide 7 Online Betting Market - Computer Go Program Beats Human Champion • Online “play-money” betting market • Claim: A machine will be the best Go player in the world, sometime before the end of 2020 • Before AlphaGo, chances were Image source: considered low, and falling http://www.ideosphere.com/ • First Nature paper changed it fx-bin/Claim?claim=GoCh around completely 8 AlphaGo Versions and Publications • Worked on Go program for about 2 years, mostly kept secret • DCNN paper published in 2015 (see previous lecture) • Fall 2015 Match AlphaGo Fan vs European champion Fan Hui (2 dan professional) • AlphaGo won 5:0 in official games, lost some other games • Match kept secret until January 2016 • January 2016 Article in Nature describes this version of AlphaGo 9 AlphaGo Versions and Publications (continued) • March 2016 Match AlphaGo Lee vs top-level human player Lee Sedol, wins 4 - 1 • January 2017 AlphaGo Master plays 60 games on internet vs top humans, wins 60 - 0 • May 2017 Match vs world #1 Ke Jie, wins 3 - 0 AlphaGo retires from match play • October 2017 AlphaGo Zero article in Nature Learns without human game knowledge • December 2017/December 2018 Alpha Zero article preprint/final - chess and shogi 10 AlphaGo Project • AlphaGo project was “Big Science” • Dozens of developers • World experts in RL, game tree search and MCTS, neural nets, deep learning • Many millions of dollars in hardware and computing costs Image source: http://sports.sina. • Huge engineering effort com.cn/go/2016-12-19/ 11 AlphaGo vs Fan Hui • Fall 2015 - early AlphaGo version AlphaGo Fan Hui • Fan Hui: trained in China, 2 dan professional • Lives in Europe since 2000, French citizen • European champion 2013 - 2015 • AlphaGo beat Fan Hui by 5:0 in Image source: official games https://www.geekwire.com/2016/ • Fan Hui won some faster, informal games 12 AlphaGo Article in Nature • Nature and Science are the top two scientific journals • Papers on computing science are rare • Papers on games are very rare • Games articles from our dept: • Checkers Is Solved, Science 2007 • Heads-up limit hold’em poker is solved, Science 2015 • January 2016 AlphaGo on title page of Nature Image source: • Describes version that beat https://www.nature.com Fan Hui 13 AlphaGo Fan Design As described in January 2016 article in Nature • Search: MCTS (pretty standard, parallel MCTS) • Modified UCT combines simulation result and value network evaluation • Simulation (rollout) policy: relatively normal • Uses small, fast network for policy • Supervised Learning (SL) policy network • DCNN, similar to their 2015 paper • Learns move prediction from master games • Improved in details, more data 14 AlphaGo Fan Design (continued) • New: strong RL policy network • Learns by Reinforcement Learning (RL) from self-play • New: value network • Learns from labeled game data created by strong RL policy • Main contributions • Reinforcement learning for training DCNN policy net • Value network for state evaluation • Large, extremely well-engineered learning and playing system 15 Rollout Policy Network • Used for simulations in MCTS • Designed to be simple and fast • “Linear softmax of small pattern features” • Probabilistic move selection as in Coulom’s paper, Go4 • Weights trained by RL instead of MM • Slightly larger patterns/extended features • 24.2% move prediction rate • 2µs per move selection (500,000 simulated moves/second) 16 AlphaGo Fan Deep Network Architecture • Three “big” networks • SL policy network, RL policy network, RL value network • Same overall design for all three • 13-layer deep convolutional NN • 192 filters in convolutional layers • 4.8ms evaluation on GPU • About 200 Evaluations/second/GPU • More than 2000x slower than simulation policy evaluation • Can learn and represent much more complex information 17 Input Features for NN Image source: AlphaGo paper • Pretty similar input as previous nets 18 Value Network • Learn a state evaluation function • Given a Go position • Computes probability of winning • No search, no simulation! • Learns mapping • From state s • To expected outcome E[z] of the game • Network architecture mostly same as policy nets • Difference: output layer • Value net outputs single number: evaluation of s • Compare policy nets: output = probability distribution 19 AlphaGo Fan Learning Pipeline and Play • Training Pipeline • AlphaGo program • Performance measurements • Games against humans 20 AlphaGo Fan Training Pipeline Supervised Learning (SL) • Rollout policy • SL policy network Reinforcement Learning • RL policy network • Value network 21 Supervised Learning (SL) Policy Network • Trained from 30 million positions from the KGS Go Server • Maximize likelihood of human move • 57% move prediction when using all simple input features • 55.7% when using only raw board input • “Small improvements in accuracy led to large improvements in playing strength” • MCTS with this network is already much better than all previous Go programs 22 RL Policy Network • Learns from self play • Learning: adjust weights of net to learn from winner of each game • Exactly same network architecture as SL net • Weights initialized from SL net • Then plays many millions of games • Keeps a pool of previous networks as opponents to avoid overfitting 23 Rewards and RL Training Procedure • Goal: learn improved weights ρ for current network • Play full game • Reward z only at end • +1 for winning, -1 for losing • Update weights ρ by stochastic gradient ascent • Ascent: go in the direction that maximizes expected outcome of game • Formula from REINFORCE learning method (Williams 1992) 24 REINFORCE Update Formula REINFORCE update formula @ log p (a j s) ∆ρ = α ρ z @ρ • ∆ρ .. change in weight ρ • α step size for gradient ascent • pρ(a j s) .. probability of playing the move a which was played in the game from state s • z .. game result (+1 or -1) 25 RL Policy Network vs Other Go Programs • Tested RL Policy Network as a standalone player • No search, only one call to network • Plays move by sampling from pρ • Won 80% against SL policy net • Won 85% vs MCTS Go program pachi which used 100,000 simulations/move (!) 26 Training the Value Net • Value net computes a function vθ(s) • Function arguments: • input state s • Value net weights θ • Output: vθ(s) is a single real number • Training: minimize squared error between • Value net prediction vθ(s) • True game outcome zi X 2 Squared error = (vθ(si ) − zi ) i • Measure mean squared error (MSE): • Squared error / number of training items 27 Training Data for Value Net • Played 30 million self-play games using the RL policy player • Randomly sample one single state s from each game • Why only one state? Because of overfitting problems • Label s with the outcome z of the game • Result: 30 million training points (si ; zi ) • Learn the value net by trying to predict zi from si • This was called “reinforcement learning of value networks” in the paper • It is really supervised learning from game data • Game data was produced by the RL policy 28 MCTS in AlphaGo Fan Player • Player uses MCTS - search and simulations • Neural nets used as (very strong) in-tree knowledge • Policy net guides tree search • Value net helps evaluate leaf nodes • 3 values stored on each edge (s,a) of game tree • Winrate Q, visit count N, prior probability P from policy net 29 In-tree Move Selection • Choose move which maximizes Q + u • Winrate Q, exploitation term • u exploration term, with multiplicative knowledge • P Decay by u = C 1+N • Exploration constant C 30 Computing V (sL) • Value estimate V (sL) of leaf node sL • V (sL) is
Details
-
File Typepdf
-
Upload Time-
-
Content LanguagesEnglish
-
Upload UserAnonymous/Not logged-in
-
File Pages44 Page
-
File Size-