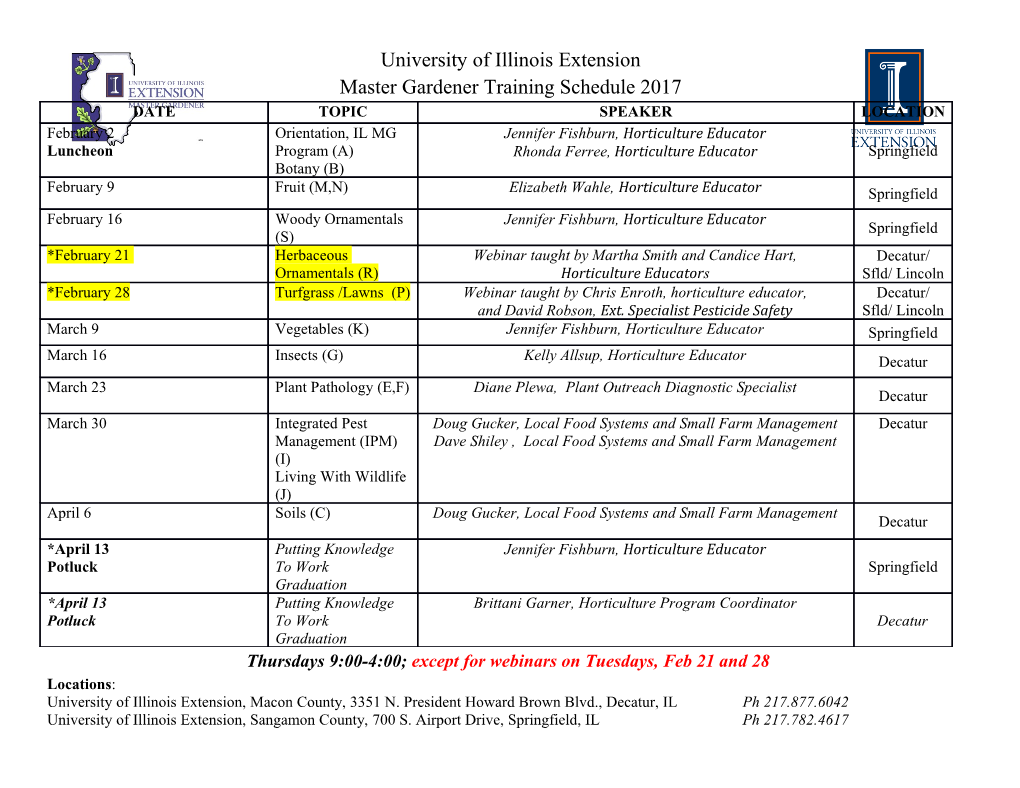
COLOR FEATURE INTEGRATION WITH DIRECTIONAL RINGLET INTENSITY FEATURE TRANSFORM FOR ENHANCED OBJECT TRACKING Thesis Submitted to The School of Engineering of the UNIVERSITY OF DAYTON In Partial Fulfillment of the Requirements for The Degree of Master of Science in Electrical Engineering By Kevin Thomas Geary Dayton, Ohio December, 2016 COLOR FEATURE INTEGRATION WITH DIRECTIONAL RINGLET INTENSITY FEATURE TRANSFORM FOR ENHANCED OBJECT TRACKING Name: Geary, Kevin Thomas APPROVED BY: _______________________________ _______________________________ Vijayan K. Asari, Ph.D. Eric J. Balster, Ph.D. Advisory Committee Chairman Committee Member Professor Associate Professor Electrical and Computer Engineering Electrical and Computer Engineering _______________________________ Theus H. Aspiras, Ph.D. Committee Member Research Engineer and Adjunct Faculty Electrical and Computer Engineering _______________________________ _______________________________ Robert J. Wilkens, Ph.D., P.E. Eddy M. Rojas, Ph.D., M.A., P.E. Associate Dean for Research and Innovation Dean, School of Engineering Professor School of Engineering ii ABSTRACT COLOR FEATURE INTEGRATION WITH DIRECTIONAL RINGLET INTENSITY FEATURE TRANSFORM FOR ENHANCED OBJECT TRACKING Name: Geary, Kevin Thomas University of Dayton Advisor: Dr. Vijayan K. Asari Object tracking, both in wide area motion imagery (WAMI) and in general use cases, is often subject to many different challenges, such as illumination changes, background variation, rotation, scaling, and object occlusions. As WAMI datasets become more common, so too do color WAMI datasets. When color data is present, it can offer very strong potential features to enhance the capabilities of an object tracker. A novel color histogram-based feature descriptor is proposed in this thesis research to improve the accuracy of object tracking in challenging sequences where color data is available. The use of a three dimensional color histogram is explored, and various color spaces are tested. It is found to be effective but overly costly in terms of calculation time when comparing reference features to the test features. A reduced, two dimensional histogram is proposed, created from three channel color spaces by removing the intensity/luminosity channel before calculating the histogram. The two dimensional histogram is also evaluated as a feature for object tracking, and it is found that the HSV two dimensional histogram iii performs significantly better than other color space histograms, and that the two dimensional histogram performs at a level very near that of the three dimensional histogram, but an order of magnitude less complex in the feature distance calculation. The proposed color feature descriptor is then integrated with the Directional Ringlet Intensity Feature Transform (DRIFT) object tracker. The two dimensional HSV color histogram is enhanced further by making use of the DRIFT Gaussian ringlets as a mask for the histogram, resulting in a set of weighted histograms as the color feature descriptor. This is calculated alongside the existing DRIFT features of intensity and Kirsch mask edge detection. The distance scores for the color feature and DRIFT features are calculated separately, given the same weight, and then added together to form the final hybrid feature distance score. The combined proposed object tracker, C-DRIFT, is evaluated on both challenging WAMI data sequences and challenging general case tracking sequences that include head, body, object, and vehicle tracking. The evaluation results show that the proposed C-DRIFT algorithm significantly improves on the average accuracy of the DRIFT algorithm. Future work on the integrated algorithm includes integrated scale change handling created from a hybrid of normalized color histograms and existing DRIFT rescaling methods. iv TABLE OF CONTENTS ABSTRACT ……………………………………………………………………………. iii LIST OF ILLUSTRATIONS …………………………………………………………… vii LIST OF TABLES …………………………………………………………………… viii CHAPTER 1: INTRODUCTION………………………………………………………... 1 CHAPTER 2: LITERATURE SURVEY ………………………………………………... 5 2.1 Image Registration Techniques ………………………………………………… 5 2.2 Tracking Algorithm Feature Extraction …………………………………………. 6 2.3 Color Feature Extraction ………………………………………………………… 8 CHAPTER 3: OVERVIEW OF DRIFT ALGORITHM ……………………………...... 10 3.1 DRIFT Tracking Technique …………………………………………………….. 10 3.2 DRIFT Tracking Results ……………………………………………………….. 19 CHAPTER 4: COLOR HISTOGRAM BASED FEATURES FOR TRACKING ….….. 22 4.1 Three Dimensional Color Histogram Features ………………………………… 22 4.2 Two Dimensional Color Histogram Features ………………………………….. 29 4.3 Color Feature Fusion with DRIFT ……………………………………………... 31 CHAPTER 5: OBJECT TRACKING EVALUATIONS …………………..…………… 36 5.1 Datasets and Testing Setup …………………………………………………….. 36 5.2 Testing Strategies and Results …………………………………………………..39 v 5.3 Discussion …………………………………………………………………... 43 CHAPTER 6: CONCLUSION ………………………….……………………………… 45 REFERENCES …………………………………………………………………………. 48 vi LIST OF ILLUSTRATIONS 1.1 Comparison of grayscale intensities of colorful cars ………………………………... 3 1.2 Color feature extraction process …………………………………………………….. 4 3.1 DRIFT object tracking method …………………………………………………….. 11 3.2 STTF nonlinear enhancement process ….…………………………………………... 12 3.3 Structure of the DRIFT feature descriptor ……………………..…………………… 14 3.4 Gaussian ring kernel, with rings 휌 = 3 ……….………….………………………….. 15 3.5 Kirsch compass kernels …...………………………………………………………… 16 3.6 Object tracking on CLIF and LAIR datasets ………………………………………. 21 4.1 RGB cube histogram ……………………………………………………………….. 23 4.2 Structure of color space testing tracking algorithm ………………………………... 27 4.3 Sample frame from Egtest01 dataset …….…………………………………………. 28 4.4 Heat map of HSV 2D histogram …………………………………………………… 30 4.5 Diagram of Color DRIFT Structure ………………………………………………… 32 4.6 FAST feature comparison between pair of frames ………………………………… 35 5.1 Frames from object tracking sequences corresponding to Table 5.1 ………………. 37 5.2 Frames from object tracking sequences corresponding to Tables 5.2 and 5.3 …….. 38 5.3 Plot of thresholded overlap success for Visual Tracker sets ……………………….. 40 5.4 Plot of thresholded center error success for Visual Tracker sets …………………... 41 vii LIST OF TABLES 3.1 Object Tracking Frame Detection Accuracy for CLIF and LAIR sets …................... 20 4.1 3D histogram tracking results by color space ……………………………………… 28 4.2 2D histogram tracking results by color space ……………………………………… 31 4.3 Comparison of tracker time per frame for 3D and 2D histograms ………………… 31 5.1 VIVID object tracking overlap …………………………………………………… 39 5.2 Visual Tracker Benchmark object tracking frame detection accuracy …………… 42 5.3 Visual Tracker Benchmark object tracking average center error ………………… 43 viii CHAPTER 1 INTRODUCTION In recent years, wide area motion imagery (WAMI) data has become increasingly common, with an abundance of use cases. These uses can range from surveillance related tasks, to search and rescue operations, to traffic pattern analysis. In many use cases, tracking of objects, especially vehicles, is necessary. Because of the large area covered by such imagery, computer vision techniques for automated tracking become increasingly important. However, object tracking in WAMI data is a challenging task due to many factors. These factors can include camera motion, variances in object illumination, object occlusion, changes in object scale, object rotation, and variations in background. Additionally, the resolution of individual objects in such imagery tends to be very low. This is because while the WAMI images themselves are very high resolution, the images are captured from high in the air. Even when multiple sensors are deployed in an array to capture the highest possible resolution image of the area, the resolution of objects such as vehicles will remain relatively low. WAMI data can also contain sequences where, due to complex lighting conditions, the contrast of an object can be very similar to that of the background. When any number of these complicating factors is present in the imagery, it becomes much more difficult to accurately track an object, compromising the purpose of the WAMI data. 1 Many of these challenging conditions are overcome when using the Directional Ringlet Intensity Feature Transform (DRIFT) tracking algorithm [1-2]. The DRIFT algorithm consists of several stages. The tracker is initialized with the location of the target object and the size of its bounding box. Before reference features are calculated, the image goes through a preprocessing step in which intensity illumination and spatial enhancement is applied. The reference features of the object are then created from the grayscale image using intensity features, and edge features are created using a Kirsch mask. These features are then filtered using Gaussian ringlet filters to create the completed model [3]. Tracking then begins by applying the same image enhancement techniques to the incoming frame. An enhanced sliding window search approach is used in the search area, with the same intensity and edge features being calculated for each candidate region. Once all candidates have been accumulated, the earth mover’s distance (EMD) is calculated to determine the distance score between each region and the reference. If the lowest distance is below a threshold, the location of that candidate region is recorded as the object location. If the distance score is below a second threshold, the reference model is updated. Lastly, a Kalman filter is applied
Details
-
File Typepdf
-
Upload Time-
-
Content LanguagesEnglish
-
Upload UserAnonymous/Not logged-in
-
File Pages62 Page
-
File Size-