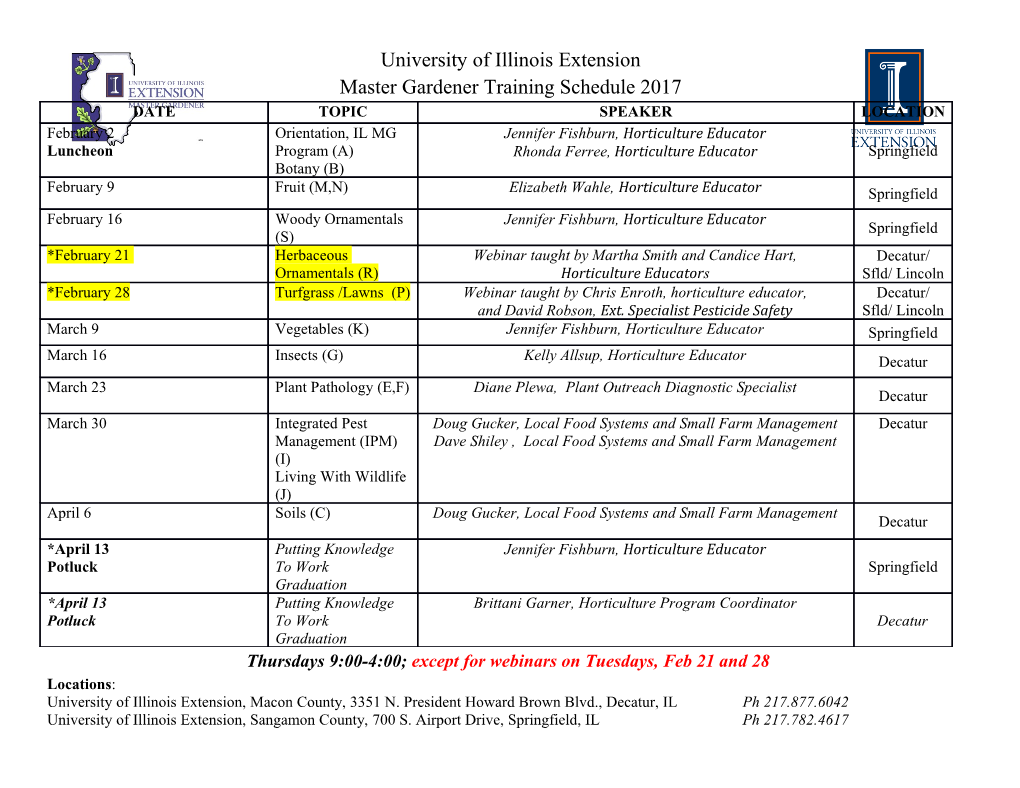
An End-to-End Automatic Cloud Database Tuning System Using Deep Reinforcement Learning Ji Zhangx, Yu Liux, Ke Zhoux , Guoliang Liz, Zhili Xiaoy, Bin Chengy, Jiashu Xingy, Yangtao Wangx, Tianheng Chengx, Li Liux, Minwei Ranx, and Zekang Lix xWuhan National Laboratory for Optoelectronics, Huazhong University of Science and Technology, China zTsinghua University, China, yTencent Inc., China {jizhang, liu_yu, k.zhou, ytwbruce, vic, lillian_hust, mwran, zekangli}@hust.edu.cn [email protected]; {tomxiao, bencheng, flacroxing}@tencent.com ABSTRACT our model and improves efficiency of online tuning. We con- Configuration tuning is vital to optimize the performance ducted extensive experiments under 6 different workloads of database management system (DBMS). It becomes more on real cloud databases to demonstrate the superiority of tedious and urgent for cloud databases (CDB) due to the di- CDBTune. Experimental results showed that CDBTune had a verse database instances and query workloads, which make good adaptability and significantly outperformed the state- the database administrator (DBA) incompetent. Although of-the-art tuning tools and DBA experts. there are some studies on automatic DBMS configuration tuning, they have several limitations. Firstly, they adopt a 1 INTRODUCTION pipelined learning model but cannot optimize the overall The performance of database management systems (DBMSs) performance in an end-to-end manner. Secondly, they rely relies on hundreds of tunable configuration knobs. Supe- on large-scale high-quality training samples which are hard rior knob settings can improve the performance for DBMSs to obtain. Thirdly, there are a large number of knobs that (e.g., higher throughput and lower latency). However, only a are in continuous space and have unseen dependencies, and few experienced database administrators (DBAs) master the they cannot recommend reasonable configurations in such skills of setting appropriate knob configurations. In cloud high-dimensional continuous space. Lastly, in cloud environ- databases (CDB), however, even the most experienced DBAs ment, they can hardly cope with the changes of hardware cannot solve most of the tuning problems. Consequently, configurations and workloads, and have poor adaptability. cloud database service providers are facing a challenge that To address these challenges, we design an end-to-end au- they have to tune cloud database systems for a large num- tomatic CDB tuning system, CDBTune, using deep reinforce- ber of users with limited and expensive DBA experts. As ment learning (RL). CDBTune utilizes the deep deterministic a result, developing effective systems to accomplish auto- policy gradient method to find the optimal configurations matic parameters configuration and optimization becomes in high-dimensional continuous space. CDBTune adopts a an indispensable way to overcome this challenge. try-and-error strategy to learn knob settings with a limited There are two classes of representative studies in DBMS number of samples to accomplish the initial training, which configuration tuning: search-based methods [55] and learning- alleviates the difficulty of collecting massive high-quality based methods [4, 14, 35]. The search-based methods, e.g., samples. CDBTune adopts the reward-feedback mechanism BestConfig [55], search the optimal parameters based on in RL instead of traditional regression, which enables end- certain given principles. However, they have two limitations. to-end learning and accelerates the convergence speed of Firstly, they spend a great amount of time on searching the optimal configurations. Secondly, they restart the search pro- cessing whenever a new tuning request comes, and thus fail Permission to make digital or hard copies of all or part of this work for to utilize knowledge gained from previous tuning efforts. personal or classroom use is granted without fee provided that copies are not made or distributed for profit or commercial advantage and that copies bear The learning-based methods, e.g., OtterTune [4], utilize this notice and the full citation on the first page. Copyrights for components machine-learning techniques to collect, process and analyze of this work owned by others than ACM must be honored. Abstracting with knobs and recommend possible settings by learning DBA’s credit is permitted. To copy otherwise, or republish, to post on servers or to experiences from historical data. However, they have four redistribute to lists, requires prior specific permission and/or a fee. Request limitations. Firstly, they adopt a pipelined learning model, permissions from [email protected]. which suffers from a severe problem that the optimal solution SIGMOD ’19, June 30-July 5, 2019, Amsterdam, Netherlands © 2019 Association for Computing Machinery. of the previous stage cannot guarantee the optimal solution ACM ISBN 978-1-4503-5643-5/19/06. in the latter stage and different stages of the model may https://doi.org/10.1145/3299869.3300085 not work well with each other. Thus they cannot optimize 1800 2000 550 1600 1750 MySQL Default 500 MySQL Default DBA 1400 DBA 1500 450 OtterTune with deep learning 1200 OtterTune with deep learning 1250 400 OtterTune OtterTune 1000 1000 350 800 750 300 600 500 250 Number of Knobs Number of Throughput (txn/sec) Throughput 400 (txn/sec) Throughput 250 200 2 4 6 8 10 12 2 4 6 8 10 12 14 1.0 2.0 3.0 4.0 5.0 6.0 7.0 Number of Samples (x1000) Number of Samples (x1000) CDB Version (a) CDB (TPC-H) (b) CDB (Sysbench) (c) Knobs Increase (d) Performance surface Figure 1: (a) and (b) show the performance of OtterTune [4] and OtterTune with deep learning over number of samples com- pared with default settings (MySQL v5.6) and configurations generated by experienced DBAs on CDB1(developed by company Tencent). (c) shows the number of tunable knobs provided by CDB in different versions. (d) shows the performance surface of CDB (Read-Write workload of Sysbench, physical memory = 8GB, disk = 100GB). the overall performance in an end-to-end manner. Secondly, alleviates the burden of collecting too many samples in ini- they rely on large-scale high-quality training samples, which tial stage of modeling and is more in line with the DBA’s are hard to obtain. For example, the performance of cloud judgements and tuning action in real scenarios. CDBTune uti- databases is affected by various factors such as memory size, lizes deep deterministic policy gradient method to find the disk capacity, workload, CPU model and database type. It optimal configurations in continuous space, which solves is hard to reproduce all conditions and accumulate high- the problem of quantization loss caused by regression in quality samples. As shown in Figures 1(a) and 1(b), without existing methods. We conducted extensive experiments un- high-quality samples, OtterTune [4] or OtterTune with deep der 6 different workloads on four types of databases. Our learning (we reproduce OtterTune and improve its pipelined experimental results demonstrated that CDBTune can recom- model using deep learning) can hardly gain higher perfor- mend knob settings that greatly improve performance with mance even though provided with an increasing number higher throughput and lower latency compared with existing of samples. Thirdly, in practice there are a large number of tuning tools and DBA experts. Besides, CDBTune has a good knobs as shown in Figure 1(c). They cannot optimize the adaptability so that the performance of CDB deployed on knob settings in high-dimensional continuous space by just configurations recommended by CDBTune will not decline using regression method like the Gaussian Process (GP) re- even though the environment (e.g., memory, disk, workloads) gression OtterTune used, because the DBMS configuration changes. Note that some other ML solutions can be explored tuning problem that aims to find the optimal solution in to improve the database tuning performance further. continuous space is NP-hard [4]. Moreover, the knobs are in In this paper, we make the following contributions: continuous space and have unseen dependencies. As shown (1) To the best of our knowledge, this is the first end-to-end in Figure 1(d), due to nonlinear correlations and dependen- automatic database tuning system that uses deep RL to learn cies between knobs, the performance will not monotonically and recommend configurations for databases. change in any direction. Besides, there exist countless combi- (2) We adopt a try-and-error manner in RL to learn the best nations of knobs because of the continuous tunable parame- knob settings with a limited number of samples. ter space, making it tricky to find the optimal solution. Lastly, (3) We design an effective reward function in RL, which in cloud environment, due to the flexibility of cloud, users enables an end-to-end tuning system, accelerates the conver- often change the hardware configuration, such as adjusting gence speed of our model, and improves tuning efficiency. the memory size and disk capacity. According to statistics (4) CDBTune utilizes the deep deterministic policy gradient from Tencent, 1,800 users have made 6,700 adjustments in method to find the optimal configurations in high-dimensional half a year. In this case, conventional machine learning have continuous space. poor adaptability which needs to retrain the model to adapt (5) Experimental results demonstrate that CDBTune with a to the new environment. good adaptability could recommend knob settings that greatly In this paper, we design an end-to-end automatic cloud improved performance and compared with the state-of-the- database tuning system CDBTune using deep reinforcement art tuning tools and DBA experts. Our system is open-sourced learning (RL). CDBTune uses the reward functions in RL to and publicly available on Github2. provide a feedback for evaluating the performance of cloud database, and propose an end-to-end learning model based 2 SYSTEM OVERVIEW on the feedback mechanism. The end-to-end design improves In this section, we present our end-to-end automatic cloud CDBTune the efficiency and maintainability of the system.
Details
-
File Typepdf
-
Upload Time-
-
Content LanguagesEnglish
-
Upload UserAnonymous/Not logged-in
-
File Pages18 Page
-
File Size-