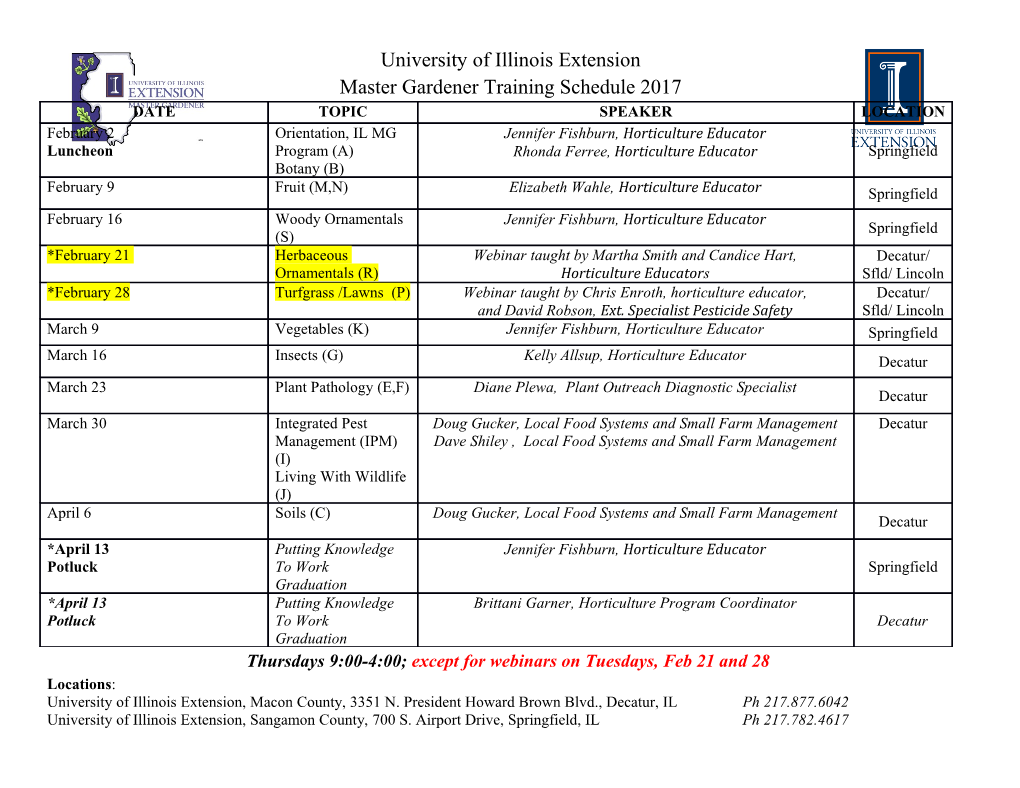
Editorial Manager(tm) for PLoS Genetics Manuscript Draft Manuscript Number: PGENETICS-D-11-00413 Title: Integrating genetic and gene expression evidence into genome-wide association analysis of gene sets Short Title: Integrative association analysis of gene sets Article Type: Research Article Section/Category: Natural Variation Keywords: gene set analysis; eQTL; integrative genomics Corresponding Author: Sayan Mukherjee Corresponding Author's Institution: Duke University First Author: Qing Xiong Order of Authors: Qing Xiong;Nicola Ancona;Elizabeth Hauser;Sayan Mukherjee;Terrence Furey Abstract: Background: Single variant or single gene analyses generally account for only a small proportion of the phenotypic variation in complex traits. Alternatively, gene set or pathway association analyses are playing an increasingly important role in uncovering genetic architectures of complex traits through the identification of systematic genetic interactions. Two dominant paradigms for gene set analyses are association analyses based on SNP genotypes and those based on gene expression profiles. However, gene-disease association can manifest in many ways such as alterations of gene expression, genotype and copy number, thus an integrative approach combining multiple forms of evidence can more accurately and comprehensively capture pathway associations. Methodology: We have developed a single statistical framework, Gene Set Association Analysis (GSAA), that simultaneously measures genome-wide patterns of genetic variation and gene expression variation to identify sets of genes enriched for differential expression and/or trait-associated genetic markers. Simulation studies illustrate that joint analyses of genomic data increase the power to detect real associations when compared to gene set methods that use only one genomic data type. Significance/Findings: The analyses of two human disease, glioblastoma and Crohn's disease, detected abnormalities in previously identified disease-associated pathways, such as pathways related to the PI3K signaling, DNA damage response, and activation of NF-κB. In addition, GSAA revealed novel pathway associations, for example differential genetic and expression characteristics in genes from the ABC transporter family in glioblastoma and from the HLA system in Crohn's disease. These demonstrate that GSAA can help uncover biological pathways underlying human diseases and complex traits. Software is freely available at http://gsaa.genome.duke.edu. Suggested Reviewers: Eleazar Eskin UCLA [email protected] Eric Stone North Carolina State Unviersity [email protected] Emmanouil Dermitzakis Wellcome Trust Sanger Institute [email protected] Vamsi Mootha Harvard University [email protected] Opposed Reviewers: Cover Letter DUKE UNIVERSITY DEPARTMENT OF STATISTICAL SCIENCE D URHAM NC 27708-0251 - USA SAYAN MUKHERJEE ASSISTANT PROFESSOR OF STATISTICAL SCIENCE COMPUTER SCIENCE AND MATHEMATICS INVESTIGATOR INSTITUTE FOR GENOME Wednesday, February 23, 2011 SCIENCES & POLICY tel/fax: +1 919 684 4608/8594 [email protected] Dear Editor: We are submitting our manuscript entitled "Integrating genetic and gene expression evidence into genome-wide association analysis of gene sets" for your review. Increasing amounts of diverse genome-wide data for complex traits and diseases are available and continue being rapidly generated, but our understanding of these data is not keeping pace. The primary goal of this study is to develop a more effective method to simultaneously leverage information from multiple genomic data, particularly genotype and expression data, to identify biological pathways underlying complex traits and diseases. We believe that this work represents a substantial advance in the joint analysis of genome-wide association data and genome-wide expression data in the context of complex traits and diseases and will be of broad interest to your readership. Two major approaches to uncover the genetic architecture of traits have been genome-wide association studies (GWAS), to examine correlations between individual genetic variants and trait variation, and gene expression-based studies, to examine correlations between gene expression and trait variation. Despite the enormous success of GWA studies, the identified single nucleotide polymorphisms (SNPs) explain only a small proportion of the phenotypic variation, and the predictive power of these SNPs remains low for many complex diseases. Gene set or pathway- based approaches have shown some success as an alternative to single locus analyses in an attempt to address the limited explanatory power of identified single variants. However, existing approaches have focused on pathway analyses of a single data type, especially genotype data or expression data, and didn’t offer a integrative solution at the pathway level that takes advantage of the complementary information available in different genome-wide data such as genotype and expression information. Our novel Gene Set Association Analysis (GSAA) method integrates multiple genomic data to better understand the biology of complex traits. GSAA simultaneously considers multiple data types employing a pathway-based approach that has been repeatedly shown to be more robust than single gene analyses and offers an increased interpretability of results. Importantly, GSAA does not require matched samples, meaning expression and genotype data need not be from the same samples. Thus, combinations of existing GWAS and expression data generated from different studies can be readily employed. We conducted a comprehensive simulation study and show that GSAA has greater power to detect association signals than other methods. We employed GSAA in an analysis ofglioblastoma and Crohn’s disease data to demonstrate its utility in analysis and interpretation. Our results show that GSAA can robustly detect signals found in either data type and better highlights key pathways that show variation at the genotype level as well as the expression level. While we concentrate on gene expression and genotype data, GSAA is easily extensible to accommodate additional data types such as DNA copy number or DNA methylation data. The general framework of GSAA will also allow the incorporation of higher resolution genome sequence data as it becomes available for greater numbers of samples in the future. In short, we believe our work described in this manuscript provides a critical methodological advance in genome analyses of complex traits and clearly illustrates its utility on data from previous studies of human disease. We believe that GSAA will greatly complement current GWAS analyses and offers an important alternative way in which to comprehensively understand the genetic underpinnings underlying complex traits and diseases. We strongly believe this work will be of great interest to a broad audience and that your journal is the proper venue for its publication. PLoS Genetics has a number of excellent associate editors on its board. For this particular work, we believe that Vivian Cheung in particular has the most appropriate knowledge and background to accurately evaluate the merits of our submission. If Dr. Cheung is unable to act as the editor, we feel that Leonid Kruglyak and GoncaloAbecasis would also be well qualified. Please note that we submitted an earlier version of this paper to PLoS Genetics. Greg Gibson was the section editor. We addressed all of the reviewer comments from that submission. This new paper is very different from our previous submission, in part due to all of the changes addressing the reviewer comments. That review and our responses are included in Yours sincerely, Sayan Mukherjee, on behalf of the other authors 2 *Manuscript Click here to download Manuscript: gsaa_manuscript.pdf 1 Integrating genetic and gene expression evidence into genome-wide association 2 analysis of gene sets 3 Qing Xiong1, Nicola Ancona2, Elizabeth R. Hauser3, Sayan Mukherjee4,#,*, Terrence S. Furey1,#,* 4 1Department of Genetics, Department of Biology, Lineberger Comprehensive Cancer Center, and 5 Carolina Center for Genomics and Society, The University of North Carolina at Chapel Hill, Chapel Hill, 6 NC 27599, USA; 7 2Institute of Intelligent Systems for Automation National Research Council, Bari IT 70126, Italy; 8 3Center for Human Genetics and Section of Medical Genetics, Department of Medicine, Duke University, 9 Durham, NC 27710, USA; 10 4Departments of Statistical Science, Computer Science, and Mathematics, Institute for Genome Sciences 11 & Policy, Duke University, Durham, NC 27708, USA; 12 #These authors contributed equally to this work 13 *E-mail: [email protected] (SM), [email protected] (TSF) 14 1 15 Abstract 16 17 Single variant or single gene analyses generally account for only a small proportion of the phenotypic 18 variation in complex traits. Alternatively, gene set or pathway association analyses are playing an 19 increasingly important role in uncovering genetic architectures of complex traits through the identification 20 of systematic genetic interactions. Two dominant paradigms for gene set analyses are association analyses 21 based on SNP genotypes and those based on gene expression profiles. However, gene-disease association 22 can manifest in many ways such as alterations of gene expression, genotype and copy number, thus an 23 integrative approach combining multiple forms of evidence can more accurately and comprehensively 24 capture pathway associations. We have developed a single statistical framework, Gene Set
Details
-
File Typepdf
-
Upload Time-
-
Content LanguagesEnglish
-
Upload UserAnonymous/Not logged-in
-
File Pages134 Page
-
File Size-