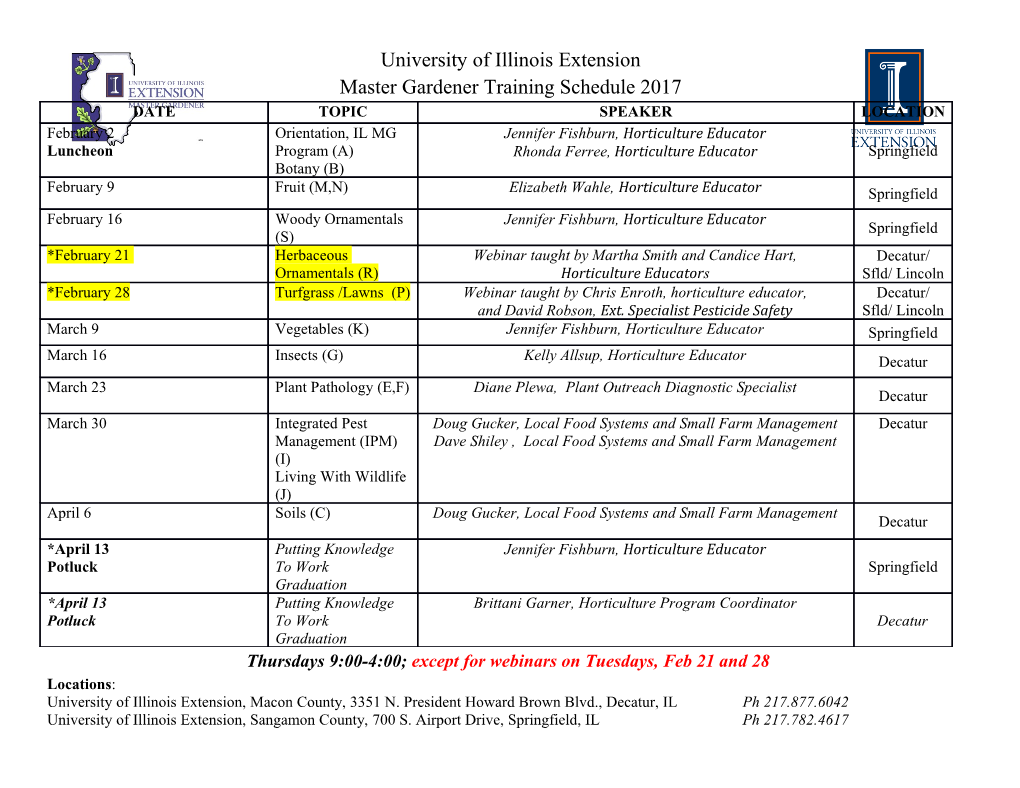
CopyTight 0 1996 by the Genetics Society of America Hierarchical Analysis of Nucleotide Diversity in Geographically Structured Populations Kent E. Holsinger and Roberta J. Mason-Gamer1 Department of Ecology and Evolutionary Biology, University of Connecticut, Storrs, Connecticut 06269-3043 Manuscript received August 1, 1994 Accepted for publication November 4, 1995 ABSTRACT Existing methods for analyzing nucleotide diversity require investigators to identify relevant hierarchi- cal levels before beginning the analysis. We describe a method that partitions diversity into hierarchical components while allowing any structure present in the datato emerge naturally.We present an unbiased version of NEI’Snucleotide diversity statistics and show that our modification has the same properties as WRIGHT’SFw We compare its statistical properties with several other FsT estimators, and we describe how to use these statistics to produce a rooted tree of relationships among the sampled populations in which the mean time to coalescence of haplotypes drawn from populations belonging to the same node is smallerthan the mean time to coalescence of haplotypes drawn from populations belonging to different nodes. We illustrate the method by applying it to data from a recent survey of restriction site variation in the chloroplast genome of Coreopsis grandiflora. OPULATION geneticists have long recognized that EXCOFFIERet al. (1992) recently described a method P the genetic diversity present in a species is hierar- appropriate for the analysis of restriction site and se- chically structured. In addition to differences among quence data that is related to WRIGHT’S F-statistics. It individuals within any one population, there may be uses a distance matrix to describe the relationship differences among populations within a givengeo- among haplotypes in the sample and partitions the vari- graphical region, differences among populations from ation present in the sample into three components: different geographical regions, and differences among variation within populations, variation among popula- entire geographical regions. SEWALL (1951, WRIGHT tions within a particular geographical region, and varia- 1965) introducedFstatistics as a way of assessing genetic tion among regions. It is formally equivalent to anested differentiation at each of these levels, and the interven- ing 40 years have repeatedly demonstrated how useful analysis of variance of the nucleotide diversity in the this approach can be (see, for example, the reviews on sample, and it is a simple generalization to several hier- patterns of electrophoretic diversity by NEVOet al. 1984, archical levels ofthe analysis ofvariance approach WEIR NEI 1987, or HAMRICK and GODT 1990). and BASTEN (1990) originally suggested. NEI (1982) In the past 10 years, population geneticists have proposed a method related to his GSr statistics for allo- turned to increasingly sensitive techniques for detecting zymes (NEI 1973), while TAKAHATAand PALUMBI genetic variation, with a view toward uncovering fine- (1985), LYNCH and CREASE(1990), andNEI and MILLER scale population structure that may be undetectable (1990) proposed similar methods for estimating the in routine allozyme surveys. In particular, population average number of nucleotide substitutions between surveys ofrestriction site and nucleotide sequencevaria- populations. Valuable as these methods are, they all tion are now becoming routine. Unfortunately, Fstatis- suffer from one important limitation. As withany tics, whether those originally proposed by WRIGHTor nested analysis ofvariance, the investigator must specify theirmore recent modifications (COCKERHAM1969, 1973; NEI 1973; WEIR and COCKERHAM1984; LONG the hierarchical structure of the data before beginning 1986), are not appropriatefor analysis of the variation the analysis. In other words, each method requires that revealed by these new techniques. F statistics are calcu- a predetermined hierarchical structure be imposed on lated from allele frequencies at many, independently the data, not inferred from it. Thus, they are not well inherited loci. The variation revealed in restriction site suited to identifying the hierarchical structure that best and sequence studies, however, almost always results reflects the pattern of genetic differentiation among from differences at sites that are not independently populations. inherited. In this paper, we describe a method for describing the hierarchical structure of nucleotide diversity in a Corresponding author: Kent E. Holsinger, Department of Ecology sample that builds on these approaches. To develop and Evolutionary Biology, U-13, University of Connecticut, Storrs, CT NEI’S 06269-3043. E-mail: [email protected] this method we provide a bias correction to (1982) ’ Current address: Haward University Herbaria, 22 Divinity Ave., nucleotide diversity statistics. This statistic provides a Cambridge, MA 02138. direct analog of Fvr that is appropriate for haplotype Genetics 142 629-639 (February, 1996) 630 K. E. andHolsinger R. J. Mason-Gamer frequency data, and we compare its statistical behavior cess involving the actual populations chosen, not the with that of other Fs7. measures based on nucleotide stochastic variation arising from the choice of popula- sequence data. We call this measure gF1, to emphasize tions (CJ: WEIRand COCKERHAM1984). Let v* = Cil its close relationship to NEI'S (1982) gYt.Using the fact 2,i,6tf Then that Fyr is equal to the ratio of the average coalescence times for different pairs of genes (SLATKIN1991), we E(v*) = E(2z,%)6,.. show how & can be used to group populations based '3 on the average time to coalescence for pairs of haplo- Assuming there is no covariance in the sampling of types. The results of this analysis can be displayed as a haplotypes from different populations, E(gtk+) = xjkxf1 tree diagram depicting the pattern of genetic differenti- for I # k (CJ: TAKAHATAand PALUMBI1985). Recalling ation among populations. The mean time to coales- this, we find that cence for two haplotypes drawn from the same node of the tree is less than that for two haplotypes drawn from different nodes. In addition to providing a more de- tailed description of the pattern of nucleotide diversity than methodsthat require pre-specified hierarchical categories, the structure of the tree can be interpreted as reflecting either patternsof gene exchange or phylo- genetic relationship and the statistical significance of the structure that is revealed can be assessed. We illus trate the method by applying it to data derived from a Thus, recent survey of restriction site variation in the chloro- plast genome of Core@sis grundi$oru in the southern United States (MASON-GAMERet al. 1995). is an unbiased estimator for v. Let 7rq be the probability THEMETHOD that haplotypes i and j differ at any nucleotide. Then the probability that two randomly chosen haplotypes Measuring nucleotide sequence diversity: Let nzkbe differ at any nucleotide is 7r = C, xixj7r* (NEI andTAJIMA the number of individuals with haplotype collected i 1981). If the differences in the sample are nucleotide from population k and nk = nib. Then = nik/nk is C, .& sequence differences, then an unbiased estimator for the maximum-likelihood estimate of x,, the frequency 71 is of haplotype i in population k. Similarly, gt = Ck &/n is the maximum-likelihood estimate of its average fre- quency, x, = Xik/n, in the set of populations being considered, where n is the number of populations in- cluded in the sample. Let 6, be the number of differ- where Nis the lengthof the nucleotide sequences. If the ences found between the ith and jth haplotypes in the differences in the sample are restriction site differences, sample. For restriction site surveys, 6, is the number of then an unbiased estimator for the nucleotide diversity restriction site differences found between two haplo- is types i and j. For nucleotide sequence surveys, 6, is the number of nucleotide differences found between two sequences i and j. Let vkbe the average number of differences between where m, is the number of restriction sites detected two haplotypes drawn at random from population k. with the ith restriction enzyme and ri is the number of NEI and TAJIMA(1981) showed that nucleotides in its recognition sequence (NEI and TA- JIMA 1981; NEI 1987). The nucleotide diversity within each population, jik, is estimated in the same way. We now have unbiased estimates for the average nu- is an unbiased estimator for vk. Similarly, we define cleotide diversity within populations, namely the mean v as the average number of differences between two of the %kr and for the nucleotide diversity in a set of haplotypes drawn at randomwithout respect to the pop- populations. Following NEI (1973), letgst be the propor- tion of diversity inthe sample due to differences among ulation from whichthey were drawn: v = xex16tr Because the sample is drawn from several popula- populations. Then tions, a correction is necessary to obtain an unbiased ff-+ &l = -, (5) estimate of v from sample data (CJ:NEI and CHESSER % 1983). In making this correction, we consider only the stochastic variation that arises from the sampling pro- where % = x%h/n is the mean of the %k ($ NEI 1982; N ucleotide Diversity Analysis Diversity Nucleotide 63 1 TAKAHATAand PALTJMBI1985). gSt differs from NEI'S genes drawn at random from the second set of popula- (1982) gt in two ways: it includes a bias correction both tions. Then it follows from (8) that for average
Details
-
File Typepdf
-
Upload Time-
-
Content LanguagesEnglish
-
Upload UserAnonymous/Not logged-in
-
File Pages11 Page
-
File Size-