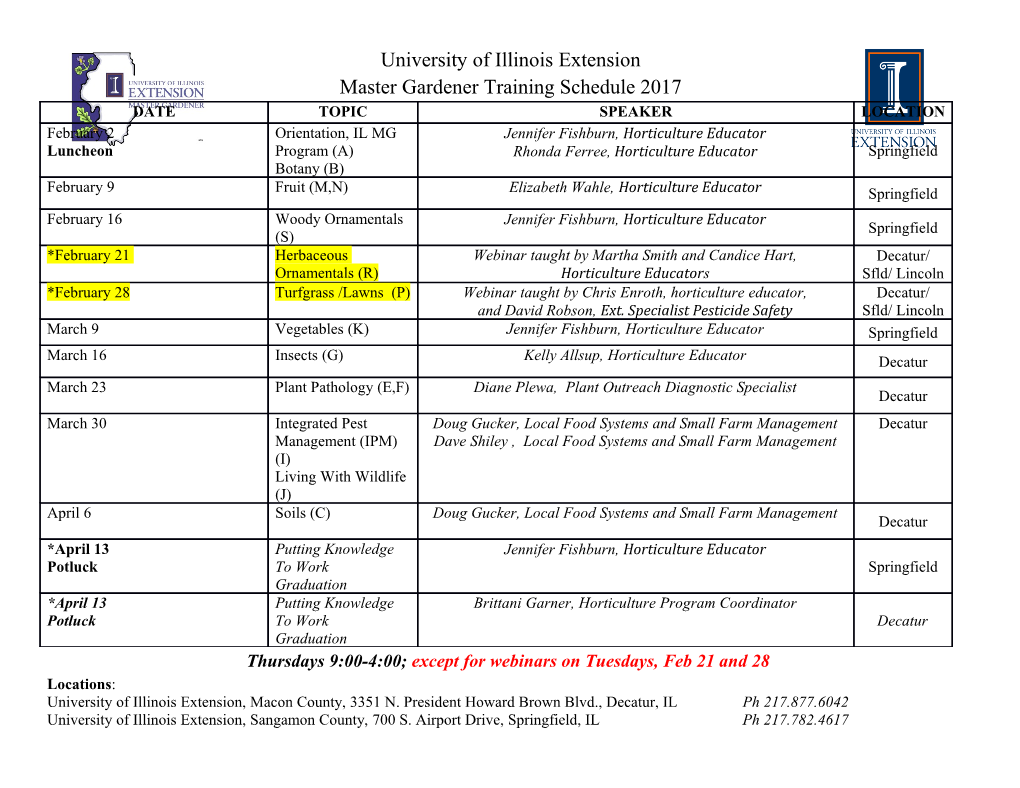
Statistical Genomics and Bioinformatics Workshop 8/16/2013 Statistical Genomics and Bioinformatics Workshop: Genetic Association and RNA-Seq Studies Population Genetics and Genome‐wide Genetic Association Studies (GWAS) Brooke L. Fridley, PhD University of Kansas Medical Center 1 Study of Genetic Association Cases Controls Genetic association studies look at the frequency of genetic changes in a group of cases and controls to try to determine whether specific changes are associated with disease. 2 1 Statistical Genomics and Bioinformatics Workshop 8/16/2013 Genetic Analysis Strategies Linkage effect GWAS Association size Rare Variant Analysis allele frequency Ardlie, Kruglyak & Seielstad (2002) Nature Genetics Reviews Zondervan & Cardon (2004) Nature Genetics Reviews 3 Genetics of Complex Traits • Multiple genes / variants – Common and rare variants – Interactions, Haplotypes, Pathways • Environment – Gene‐Environment interaction 4 2 Statistical Genomics and Bioinformatics Workshop 8/16/2013 In reality, much more complex! 5 NIHGRI GWAS Catalog (8/11/2013) http://www.genome.gov/gwastudies/ 6 3 Statistical Genomics and Bioinformatics Workshop 8/16/2013 Population Genetics 7 Recombination A1 B1 D1 Before meioses A2 B2 D2 A1 B1 D2 Crossovers occur during meioses A2 B2 D1 D2 A1 B1 After recombination A2 B2 D1 8 4 Statistical Genomics and Bioinformatics Workshop 8/16/2013 Linkage Disequilibrium (LD) • Particular alleles at neighboring loci tend to be co-inherited. • For tightly linked loci, this co-inheritance might lead to associations between alleles in the population. • LD describes the situation where particular alleles at nearby loci occur together on the same chromosome more often than expected by chance D2 A1 B1 A2 B2 D1 9 Linkage of a Marker with a Disease Locus A a a a A a A a a a a a 10 5 Statistical Genomics and Bioinformatics Workshop 8/16/2013 Use of LD for Genetic Association Studies • SNPs are markers, many have no function • Indirect association LD between SNP marker and causal variant 11 LD measures • LD Varies by race – Recent populations have higher LD (less recombination) – African populations are more genetically diverse than European populations • pairwise measure • D = observed - expected haplotype frequency •D′ (-1 < D′ <1) – standardized = D / max possible value of D – Related to recombination history, D’=1 means no recombination •r2 (0 < r2 <1) – correlation coefficient – less sensitive to low MAFs 12 6 Statistical Genomics and Bioinformatics Workshop 8/16/2013 LD Measures: r2 •r2 = 1 ‘perfect’ LD – Occurs if only two (of four possible) haplotypes are present – Two markers provide identical information – Stronger condition than ‘complete’ LD •r2 = 0 two markers are in perfect equilibrium • Sample size needed to detect association using a surrogate marker is equal to N/r2 13 LD by Racial Populations RRM1 in African American Population RRM1 in White, Non-Hispanic Population 14 7 Statistical Genomics and Bioinformatics Workshop 8/16/2013 Genetic data support hypotheses that humans migrated out of Africa • National Geographic Society’s Genographic Project, https://genographic.nationalgeographic.com/genographic/lan/en/atlas.html 15 Typical Steps in a GWAS • Study Enrollment (case-control; cohort) • Extract DNA from blood sample • Genotype (Illumina, Affymetrix or NGS) • QC genotypes / data • Genotype – phenotype association analysis – Population Stratification – Genetic Models – Multiple Testing Correction – Environment, interactions • Visualization of results • Replication / Validation 16 8 Statistical Genomics and Bioinformatics Workshop 8/16/2013 Study Designs Intervention studies (experiments) 1. Clinic trials 2. Pharmacogenomic studies Observational studies 1. The simplest is the Cross- Sectional (Prevalence) design which is conducted completely at present. 2. The Cohort (Prospective) design measures exposure in the present and the phenotype in the future. 3. The Case-Control (Retrospective) design measures the phenotype in the present and looks backwards for exposure history. 17 Study Designs in Genetic Epidemiology 18 9 Statistical Genomics and Bioinformatics Workshop 8/16/2013 Case‐Control Studies Case-control studies are used to identify factors that may contribute to a medical condition by comparing subjects who have that condition (the ‘cases’) with patients who do not have the condition but are otherwise similar (the ‘controls’) Case-control studies are retrospective and non-randomized 19 Case‐Control selection Cases and controls should be sampled from the same homogeneous population – Confounders - age, sex, ethnic background, ... Population-based cases: include all subjects or a random sample of all subjects with the disease at a single point or during a given period of time in the defined population. Hospital-based cases: all patients in a hospital department at a given time 20 10 Statistical Genomics and Bioinformatics Workshop 8/16/2013 Selection of Controls Study base: Controls can be used to characterise the distribution of exposure Comparable-accuracy: Equal reliability in the information obtained from cases and controls (to avoid systematic misclassification) Overcome confounding: Elimination of confounding through control selection (matching or stratified sampling) 21 Comparison of Study Designs Cohort study Case-control study • Rare exposure • Quick, inexpensive • Examine multiple • Well-suited to the effects of a single evaluation of exposure diseases with long • Minimizes bias in the latency period in exposure • Rare diseases determination • Direct measurements • Examine multiple of incidence of the etiologic factors for a disease single disease 22 11 Statistical Genomics and Bioinformatics Workshop 8/16/2013 Comparison of Study Designs Cohort study Case-control study • Not rare diseases • Not rare exposure • Prospective: • Incidence rates Expensive and time consuming cannot be estimated • Retrospective: in unless the study is adequate records population based • Validity can be • retrospective, non- affected by losses to randomized nature follow-up limits the conclusions that can be drawn from them. 23 Data Structure individual affection gender SNP 1 SNP 2 … SNP n 11F21…2 21M22…1 30F12…2 41F11…2 50M0-9 …1 sample id case/control genotypes 24 12 Statistical Genomics and Bioinformatics Workshop 8/16/2013 Allele and Genotype frequencies Allele distribution Genotype distribution T A Total T|T T|A A|A Total Cases 2R Cases R Controls 2S Controls S Total 2N Total N Counts alleles 2*N Counts genotypes N observations observations 25 Hardy-Weinberg Equilibrium (HWE) Predicts constant genotype frequencies within large randomly mating population. If these rules hold, then the locus is in HWE Let p = minor allele frequency (MAF) Minor/Rare allele = a Major/Common allele = A Let 1 – p = major allele frequency Genotype AA aA or Aa aa P(Genotype) (1‐p)*(1‐p) = p*(1‐p)+ (1‐p)*p = p*p = p2 (1‐p)2 2p(1‐p) 26 13 Statistical Genomics and Bioinformatics Workshop 8/16/2013 Hardy-Weinberg Equilibrium (HWE) . Explanations for deviation from HWE . Non-random mating (i.e., population stratification) . Genotype errors . Preferential selection . What if a marker is not in HWE? . Use tests for association that do not depend on HWE 27 Hardy-Weinberg Equilibrium (HWE) Tests for HWE: • Goodness-of-fit statistic (Chi-square test) d.f. = # distinct heterozygotes = k(k-1)/2 small n, p values may not be accurate. • Likelihood exact test based on likelihood of genotypes under HWE, conditioned on observed allele frequencies 28 14 Statistical Genomics and Bioinformatics Workshop 8/16/2013 HWE vs. Linkage Disequilibrium AB d AB D HWE AB d ab D LD ab d AB D AB d AB D 29 Quality Control of SNPs • Exclude SNPs that failure the Hardy- Weinberg test -- Expected proportions of genotypes are not consistent with observed allele frequency -- HWE p-value < 10-6 (for GWAS) • Genotyping success rate < 95% • Differential missingness in cases and controls 30 15 Statistical Genomics and Bioinformatics Workshop 8/16/2013 Quality Control of Samples • Poor quality samples -- Sample genotype success rate < 95 to 97.5% -- Greater proportion of heterozygous genotypes than expected • Related individuals (if independent samples) -- Based on pair-wise comparisons of similarity of genotypes (IBD estimation) • Samples with miss specified gender 31 Genetic Models • T = Minor Allele C = Major Allele Genotype Co-dominant / Additive Dominant Recessive General TT (homozygous) 0 0 -1 (2) 0 0 TC (heterozygous) 1 0 0 (1) 1 0 CC (wildtype) 1 1 1 (0) 1 1 Degrees of freedom 2111 Lettre , Lange, Hirschhorn (2007) Genetic model testing and statistical power in population-based association studies of 32 quantitative traits, Genetic Epidemiology 16 Statistical Genomics and Bioinformatics Workshop 8/16/2013 Statistical Models for GWAS • Depending on the study design, more complicated models can be used – Gene-environment, repeated measures, non- parametric methods • Time to Event Outcome = Cox Proportional Hazards Models • Binary (case/control) = Logistic Regression Models; Chi-Square Tests • Quantitative = Linear Regression Models 33 Single Locus Analysis C/C C/T T/T Not Trait Missing wildtype heterozygous homozygous Missing Control 387 (72%) 136 (25%) 18 (3%) 12 541 477 Case 304 (64%) 152 (32%) 21 (4%) 5 P-values 2 df Chisq-test = 0.029 Dominant 0.006 Fisher’s Exact test = 0.029 Recessive 0.374 Additive 0.011 Armitage trend test = 0.011 Unstructured:1 0.012 Allelic test = 0.009 Unstructured:2 0.231 34 17 Statistical Genomics and Bioinformatics
Details
-
File Typepdf
-
Upload Time-
-
Content LanguagesEnglish
-
Upload UserAnonymous/Not logged-in
-
File Pages47 Page
-
File Size-