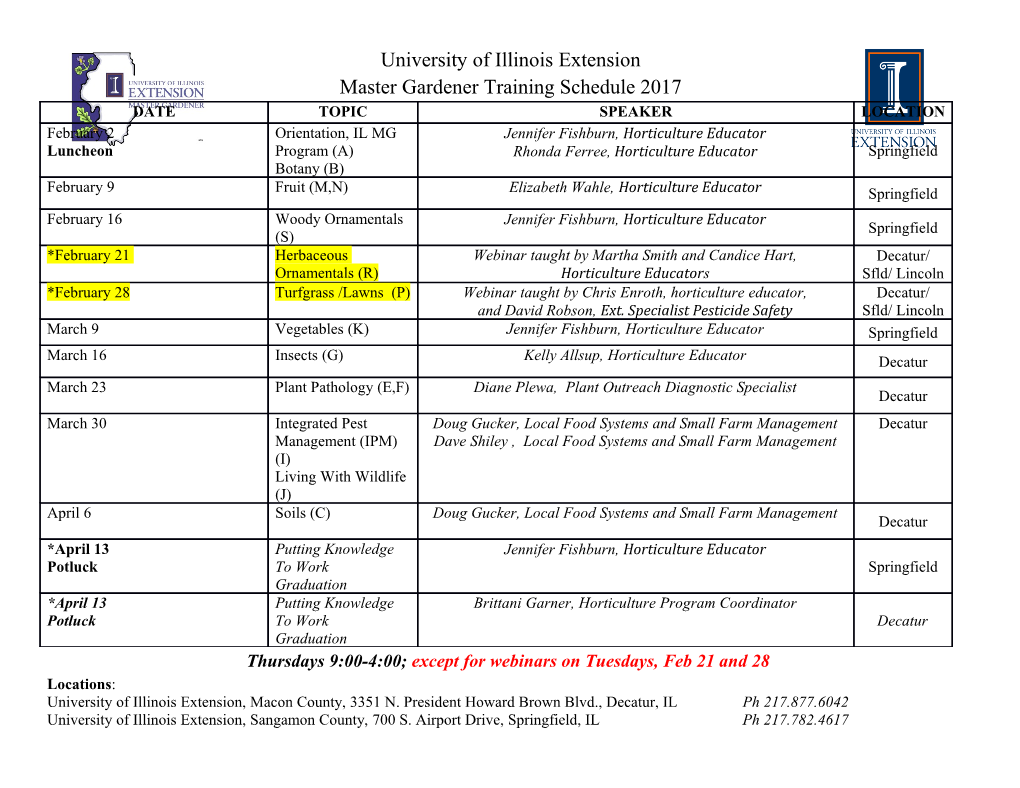
Genetics: Early Online, published on May 8, 2018 as 10.1534/genetics.117.300630 1 Comparison of Genotypic and Phenotypic Correlations: 2 Cheverud’s Conjecture in Humans 3 4 Sebastian M. Sodini*, Kathryn E. Kemper*, Naomi R. Wray*†, Maciej Trzaskowski* 5 6 * Institute for Molecular Bioscience, The University of Queensland, St Lucia, Brisbane, 4072, 7 Australia 8 † Queensland Brain Institute, The University of Queensland, St Lucia, Brisbane, 4072, Australia 9 Copyright 2018. 1 10 Running Title: Cheverud’s Conjecture in Humans 11 12 Keywords: genetic correlation, genetic proxy, LD score regression, morphological non-morphological 13 traits, UK Biobank 14 15 Corresponding Author: Dr. Maciej Trzaskowski, Institute for Molecular Bioscience, 306 Carmody 16 Road, The University of Queensland, St Lucia, 4072, Brisbane, Australia. Phone number: 17 +61733466430; e-mail address: [email protected] 18 19 20 21 Abstract 22 Accurate estimation of genetic correlation requires large sample sizes and access to genetically 23 informative data, which are not always available. Accordingly, phenotypic correlations are often 24 assumed to reflect genotypic correlations in evolutionary biology. Cheverud’s conjecture asserts that 25 the use of phenotypic correlations as proxies for genetic correlations is appropriate. Empirical evidence 26 of the conjecture has been found across plant and animal species, with results suggesting that there is 27 indeed a robust relationship between the two. Here, we investigate the conjecture in human 28 populations, an analysis made possible by recent developments in availability of human genomic data 29 and computing resources. A sample of 108,035 British European individuals from the UK Biobank 30 was split equally into discovery and replication datasets. 17 traits were selected based on sample size, 31 distribution and heritability. Genetic correlations were calculated using linkage disequilibrium score 32 regression applied to the genome-wide association summary statistics of pairs of traits, and compared 33 within and across datasets. Strong and significant correlations were found for the between-dataset 34 comparison, suggesting that the genetic correlations from one independent sample were able to predict 35 the phenotypic correlations from another independent sample within the same population. Designating 36 the selected traits as morphological or non-morphological indicated little difference in correlation. The 37 results of this study support the existence of a relationship between genetic and phenotypic correlations 38 in humans. This finding is of specific interest in anthropological studies, which use measured 39 phenotypic correlations to make inferences about the genetics of ancient human populations. 40 41 Introduction 42 Genetic correlations are a measure of genetic factors shared between two traits. When two traits 43 are highly genetically correlated, the genes that contribute to the traits are usually co-inherited (Lynch 44 & Walsh, 1998). While traditionally used in animal breeding (Lynch & Walsh, 1998), in a broader 2 45 research context, genetic correlations contribute to understanding the development and pathways of 46 traits, population level gene flow and the co-occurrences of traits (Via & Hawthorne, 2005). For this 47 reason, genetic correlations play an important role in evolutionary biology, and estimates of genetic 48 correlations are also used in theoretical modelling of human populations. 49 Genetic correlations (푟) are calculated from the additive genetic variance and covariance 50 between traits, as shown for traits X and Y, 푐표푣푔(푋,푌) 푐표푣푔(푋,푌) 51 푟 = , or for standardized traits where the phenotypic variances are one, 푟 = , where √푉푔푋푉푔푌 2 2 √ℎ푋ℎ푌 2 2 52 ℎ푋and where ℎ푌are the heritability estimates of the two traits and 푉푋and 푉푌are the variances of the 53 traits. 54 Traditionally genetic correlations are calculated from pedigree data using statistical methods to 55 partition phenotypic (co)variance into genetic variance and genetic covariance (Henderson 1986). 56 More recent methods make use of genome-wide single nucleotide polymorphism (SNP) data and the 57 very small coefficients of relationship between very large numbers of unrelated individuals in order to 58 calculate these parameters (Lee et al., 2012). Since only common variants are included in the 59 calculations, this approach assumes that the genetic correlation is the same across the allelic frequency 60 spectrum. Accepting this caveat as reasonable, the approach has an advantage over the traditional 61 methods, as unrelated individuals are less likely to have had exposure to similar environmental effects, 62 reducing confounding from shared environment. Additionally, as genotyping becomes cheaper, 63 genome-wide SNP data is becoming more readily and widely available than pedigree data. Moreover, 64 unbiased estimates of genetic correlations are achievable with minimal computing resources from 65 analysis of summary statistics from genome-wide association studies via the LD-score regression 66 method (Bulik-Sullivan et al., 2015a; Ni et al., 2017). 3 67 The sampling variance of a genetic correlation estimate, depends on, and is larger than, the 68 sampling variances of the concurrently estimated heritabilities (Robertson, 1959; Visscher et al., 69 2014). Hence, large sample sizes are needed to estimate genetic correlations with accuracy. James 70 Cheverud proposed in 1988 that phenotypic correlations (푟푝) could be used as a proxy for genetic 71 correlations (Cheverud, 1988). 72 Whilst there has been criticism of the conjecture, most notably by Willis et al. (1991), 73 subsequent studies in various organisms have provided much empirical evidence and theory supporting 74 the conclusion. Roff (1996) considered a variety of traits from previously published datasets. This 75 investigation showed that the relationship between the two correlations was most concordant in 76 morphological traits, as opposed to behavioral or life history traits. In addition, while the average 77 absolute disparity (Dabs = |rp-rg| (Willis et al., 1991)) between the correlations was relatively high (0.24- 78 0.46), this difference could be attributed to the sampling error of rg. In 2008, Kruuk et al. repeated the 79 analysis of Roff’s 1996 paper with more recent data with an increased sample size, reaching similar 80 conclusions. 81 The suitability of using phenotypic correlations as a proxy for genetic ones in various traits has 82 been discussed by Hadfield et al. (2007), concluding that while the conjecture may be true in traits 83 with high heritability, particularly those related to growth, there are still exceptions, and the conjecture 84 most likely does not apply to all traits generally. Since phenotypic correlations depend both on the 85 correlation of additive genetic and on the correlation of environmental effects (with the term 86 environmental representing any effects that are not additive genetic), differences between phenotypic 87 and genetic correlations must be explained by the relationship between genetic and environmental 88 effects. Cheverud (1984) suggests that most environmental effects often act in the same direction and 89 through the same pathways as genetic effects, which leads to a similarity between phenotypic and 90 genetic correlations. Hadfield (2007), on the other hand, suggested that certain traits have 4 91 environmental effects that act in the opposite direction to the genetic effects, which could reflect the 92 conclusion of Roff (1996), who found lower correlation for life history and behavioral traits than 93 morphological ones. 94 Despite the possible deficiencies of the conjecture when applied to non-morphological traits, 95 behavioral researchers often assume that correlations between behaviors can give insight into the 96 genetics behind the behavior. In order to test this assumption, Dochtermann (2011) tested the 97 relationship between published behavioral genetic and phenotypic correlations from animal studies. 98 The author found that while the correlation between the phenotypic and genetic correlations was high 99 (r=0.86), the mean absolute difference between traits was also high (0.27), suggesting that phenotypic 100 correlations were not a good predictor of genetic correlations between behavioral traits. Dochtermann 101 found that while not a good predictor, the phenotypic correlation is able to reliably provide information 102 on the direction of the genetic effect for behavioral research, still allowing to make certain genetic 103 conclusions based on phenotypic data. 104 To date, studies investigating the existence of Cheverud’s conjecture in specific populations 105 have looked at morphological traits in insects (Roff, 1995; Reusch & Blanckenhorn, 1998), tamarins 106 (Ackermann & Cheverud, 2002), and plants (Waitt & Levin, 1998) with results corroborating the 107 findings of Cheverud and Roff. While the conjecture has not been investigated in humans, it has been 108 applied in human modelling. As genetic data are not directly accessible in many ancient human 109 populations, phenotypic traits have been used to make conclusions regarding genetic information 110 (Weaver et al., 2007; Relethford & Blangero, 1990). 111 While the proportionality of phenotypic and genetic correlations has been assumed to be true in 112 human populations, there has yet to be a study to investigate the conjecture in the context of humans. 113 This study aims to fill the gap in understanding how the conjecture
Details
-
File Typepdf
-
Upload Time-
-
Content LanguagesEnglish
-
Upload UserAnonymous/Not logged-in
-
File Pages22 Page
-
File Size-