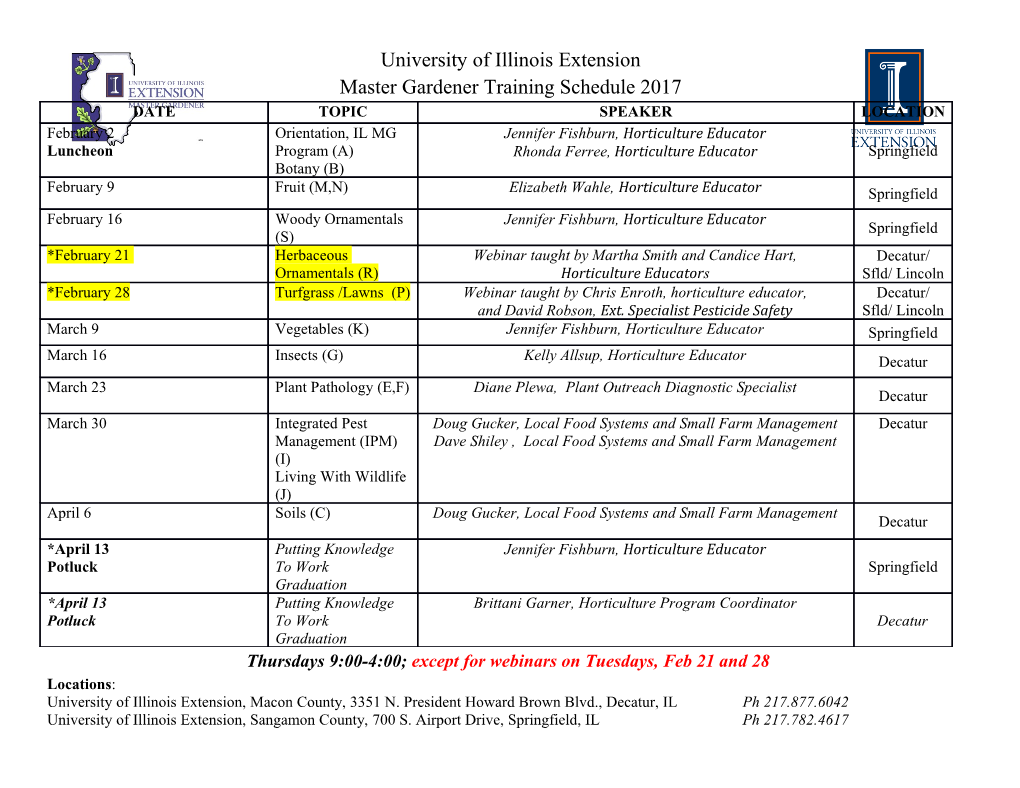
Sum of the masses of the Milky Way and M31: A likelihood-free inference approach Pablo Lemos, Niall Jeffrey, Lorne Whiteway, Ofer Lahav, Noam I. Libeskind, Yehuda Hoffman To cite this version: Pablo Lemos, Niall Jeffrey, Lorne Whiteway, Ofer Lahav, Noam I. Libeskind, et al.. Sum ofthe masses of the Milky Way and M31: A likelihood-free inference approach. Phys.Rev.D, 2021, 103 (2), pp.023009. 10.1103/PhysRevD.103.023009. hal-02999468 HAL Id: hal-02999468 https://hal.archives-ouvertes.fr/hal-02999468 Submitted on 26 Apr 2021 HAL is a multi-disciplinary open access L’archive ouverte pluridisciplinaire HAL, est archive for the deposit and dissemination of sci- destinée au dépôt et à la diffusion de documents entific research documents, whether they are pub- scientifiques de niveau recherche, publiés ou non, lished or not. The documents may come from émanant des établissements d’enseignement et de teaching and research institutions in France or recherche français ou étrangers, des laboratoires abroad, or from public or private research centers. publics ou privés. PHYSICAL REVIEW D 103, 023009 (2021) Sum of the masses of the Milky Way and M31: A likelihood-free inference approach Pablo Lemos ,1,* Niall Jeffrey ,2,1 Lorne Whiteway ,1 Ofer Lahav,1 Noam Libeskind I,3,4 and Yehuda Hoffman5 1Department of Physics and Astronomy, University College London, Gower Street, London WC1E 6BT, United Kingdom 2Laboratoire de Physique de l’Ecole Normale Sup´erieure, ENS, Universit´e PSL, CNRS, Sorbonne Universit´e, Universit´e de Paris, Paris, France 3Leibniz-Institut fr Astrophysik Potsdam (AIP), An der Sternwarte 16, 14482 Potsdam, Germany 4University of Lyon, UCB Lyon-1/CNRS/IN2P3, IPN Lyon, France 5Racah Institute of Physics, Hebrew University, Jerusalem, 91904 Israel (Received 29 October 2020; accepted 18 December 2020; published 11 January 2021) We use density estimation likelihood-free inference, Λ cold dark matter simulations of ∼2M galaxy pairs, and data from Gaia and the Hubble Space Telescope to infer the sum of the masses of the Milky Way and Andromeda (M31) galaxies, the two main components of the local group. This method overcomes most of the approximations of the traditional timing argument, makes the writing of a theoretical likelihood unnecessary, and allows the nonlinear modeling of observational errors that take into account correla- M M tions in the data and non-Gaussian distributions. We obtain an 200 mass estimate MWþM31 ¼ 4 6þ2.3 1012 M . −1.8 × ⊙ (68% C.L.), in agreement with previous estimates both for the sum of the two masses and for the individual masses. This result is not only one of the most reliable estimates of the sum of the two masses to date, but is also an illustration of likelihood-free inference in a problem with only one parameter and only three data points. DOI: 10.1103/PhysRevD.103.023009 I. INTRODUCTION applications for which LFI could improve the robustness of parameter inference using cosmological data. In this work Likelihood-free inference (LFI) has emerged as a very we perform a LFI-based parameter estimation of the sum of promising technique for inferring parameters from data, masses of the Milky Way and M31. The likelihood function particularly in cosmology. It provides parameter posterior for this problem requires significant simplifications, but probability estimation without requiring the calculation of forward simulations can be obtained easily. an analytic likelihood (i.e., the probability of the data being The Milky Way and Andromeda are the main compo- observed given the parameters). LFI uses forward simu- nents of the Local Group, which includes tens of smaller lations in place of an analytic likelihood function. Writing a galaxies. We define M 31 as the sum of the MW and likelihood for cosmological observables can be extremely MWþM M31 masses. Estimating M 31 remains an elusive and complex, often requiring the solution of Boltzmann equa- MWþM complex problem in astrophysics. As the mass of each of tions, as well as approximations for highly nonlinear the Milky Way and M31 is known only to within a factor of 2, processes such as structure formation and baryonic feed- back. While simulations have their own limitations and are it is important to constrain the sum of their masses. The traditional approach is to use the so-called timing argument computationally expensive, the quality and efficiency of M cosmological simulations are constantly increasing, and (TA) [9]. The timing argument estimates MWþM31 using they are likely to soon far surpass the accuracy or robust- Newtonian dynamics integrated from the big bang. This ness of any likelihood function. integration is an extremely simplified version of a very This is a rapidly growing topic in cosmology, due to the complex problem. Therefore, alternative methods that do not emergence of novel methods for likelihood-free inference rely on the same approximations become extremely useful. [see e.g., [1,2]], with applications to data sets such as the In this work, we use the multidark Planck (MDPL) – joint light curve (JLA) and Pantheon supernova datasets simulation [10 12], combined with data from the Hubble [3,4], and the Dark Energy Survey science verification Space Telescope [HST, [13] and Gaia [14], to estimate M data [5], among others [6–8]. There are, therefore, many MWþM31. A similar data set was previously used in [15] to M obtain a point estimate of MWþM31 using Artificial Neural Networks (ANN) in conjunction with the TA. In contrast, *[email protected] our work uses density estimation likelihood-free inference 2470-0010=2021=103(2)=023009(13) 023009-1 © 2021 American Physical Society PABLO LEMOS et al. PHYS. REV. D 103, 023009 (2021) M r v v −1 TABLE I. Estimates of MWþM31 from previous work. The third column shows the data used, with in Mpc and r, t in km s . The M 1012 M fourth column shows MWþM31 in units of ⊙. Note that Gaussian approximations have been used to convert the reported confidence levels to 68% confidence levels in some cases. r v v M Reference Method Assumed ( , r, t) MWþM31 5 27þ2.48 Li & White (2008) [27] TA calibrated on Sims (0.784, 130, 0) . −0.91 4 2þ2.1 Gonzalez & Kravtsov (2014) [28] Sims (0.783, 109.3, 0) . −1.2 et al. Λ 0 77 0 04; 109 4 4 4; 17 17 4 7þ0.7þ2.9 McLeod (2017) [15] TA þ ð . Æ . Æ . Æ Þ . −0.6−1.8 0 77 0 04; 109 4 4 4; 17 17 4 9þ0.8þ1.7 McLeod et al. (2017) [15] Sims þ ANN þ Shear ð . Æ . Æ . Æ Þ . −0.8−1.3 Phelps et al. (2013) [26] Least Action (0.79, 119, 0) 6.0 Æ 0.5 [DELFI [2,16–18]], using the PYDELFI package [19], A second approach is to consider the dynamics of all the combined with more recent data. While the result is galaxies in the local group using the least action principle important on its own, this paper also illustrates the [25], as, for example, was implemented in [26]. In this fundamental methodology of DELFI in a problem that is approach all members of the local group appear in the statistically simple but physically complex. model, but as a result the derived masses are correlated, and The structure of the paper is as follows: Sec. II reviews the error bars should be interpreted accordingly. A third M and describes previous estimates of MWþM31. Section III approach is to use N-body simulations of the local universe, describes the basics of LFI, and the particular techniques assuming a cosmological model such as ΛCDM [15,27,28]. used in this work. Section IV and Sec. V describe the In this paper we apply this third method, but using the simulations and data, respectively, used in this work. DELFI method (this provides significant improvements Section VI shows our results, and conclusions are presented over other methods, as discussed in the following section). M in Sec. VII. Representative results for MWþM31 from previous works are given in Table I. Throughout the paper we quote II. PREVIOUS ESTIMATES 68% credible intervals. M A first approach to estimating MWþM31 from dynamics, III. LIKELIHOOD-FREE INFERENCE known as TA, is based on the simple idea that MWand M31 are point masses approaching each other on a radial orbit In Bayesian statistics we often face the following D that obeys problem: given observed data obs, and a theoretical model I with a set of parameters θ, calculate the probability of the GM 1 r̈ − MWþM31 Λr; parameters given the data. In other words, we want to ¼ 2 þ ð1Þ P ≡ p θ D ;I r 3 calculate the posterior distribution ð j obs Þ; here p is a probability (for a model with discrete parameters) or a M where MWþM31 is the sum of the masses of the two probability density (for continuous parameters). We do so galaxies [9,20], and where the Λ term, which represents a using Bayes’ theorem: form of dark energy, was added in later studies [21,22].It p D θ;I p θ I L Π was also extended for modified gravity models [23]. Since p θ D ;I ð obsj Þ ð j Þ ⇔ P × r ð j obs Þ¼ p D I ¼ Z ð2Þ we know the present-day distance between MW and M31 ð obsj Þ and their relative radial velocity vr, and if we assume the Λ M age of the universe and , we can infer the mass MWþM31. where L is called the likelihood, Π the prior, and Z the The analysis can be extended to cover the case of nonzero Bayesian evidence.
Details
-
File Typepdf
-
Upload Time-
-
Content LanguagesEnglish
-
Upload UserAnonymous/Not logged-in
-
File Pages14 Page
-
File Size-