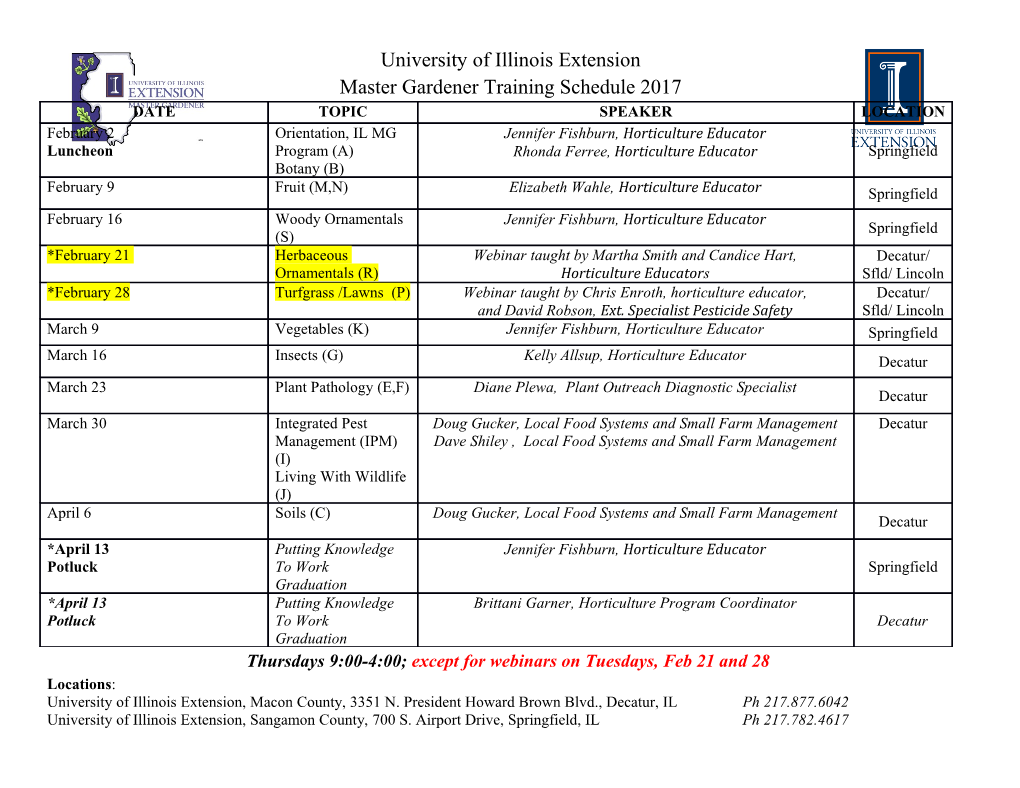
land Article Adding Space to Disease Models: A Case Study with COVID-19 in Oregon, USA Nathan H. Schumaker 1,* and Sydney M. Watkins 2 1 Department of Fisheries and Wildlife, Oregon State University Corvallis, Corvallis, OR 97331, USA 2 Computational Ecology Group, Canmore, AB T1W 3L4, Canada; [email protected] * Correspondence: [email protected] Abstract: We selected the COVID-19 outbreak in the state of Oregon, USA as a system for developing a general geographically nuanced epidemiological forecasting model that balances simplicity, realism, and accessibility. Using the life history simulator HexSim, we inserted a mathematical SIRD disease model into a spatially explicit framework, creating a distributed array of linked compartment models. Our spatial model introduced few additional parameters, but casting the SIRD equations into a geographic setting significantly altered the system’s emergent dynamics. Relative to the non-spatial model, our simple spatial model better replicated the record of observed infection rates in Oregon. We also observed that estimates of vaccination efficacy drawn from the non-spatial model tended to be higher than those obtained from models that incorporate geographic variation. Our spatially explicit SIRD simulations of COVID-19 in Oregon suggest that modest additions of spatial complexity can bring considerable realism to a traditional disease model. Keywords: HexSim; spatially explicit model; simulation model; SIRD model; COVID-19; epidemiol- ogy; compartment model Citation: Schumaker, N.H.; Watkins, S.M. Adding Space to Disease Models: 1. Introduction A Case Study with COVID-19 in Oregon, USA. Land 2021, 10, 438. Disease spread is an inherently spatial process [1–6], yet many epidemiological models https://doi.org/10.3390/land10040438 are non-spatial or pseudo-spatial [7]. This discrepancy reflects the fact that representing explicit spatial structures and nuanced spatiotemporal feedback mechanisms within math- Academic Editor: Audrey Mayer ematical and computer models is difficult. Yet, landscape structure clearly influences disease dynamics. Exposure involves the exchange of pathogens between individuals, or Received: 24 March 2021 an individual and its environment, thus tying rates of infection to population distribution Accepted: 17 April 2021 and movement behavior [8–11]. Furthermore, population-wide emergent properties af- Published: 20 April 2021 fecting these processes, such as occupancy rates and source-sink patterns, exhibit complex spatial structures of their own [12–16]. Likewise, the consequences of infection will vary Publisher’s Note: MDPI stays neutral depending on where transmission occurs [17,18]; introducing a novel pathogen into a with regard to jurisdictional claims in densely populated area would be more likely to result in an outbreak than if the infection published maps and institutional affil- arose in a location with few occupants [19,20]. These concerns, and widespread interest in iations. the role geography plays in disease dynamics, have fueled the development of specialized and highly sophisticated mathematical and computer models [21–25]. Advanced mathematical models can be difficult to understand, and have limited ability to represent dynamic spatial processes operating within complex landscapes [26–29]. Copyright: © 2021 by the authors. Similarly, while a large and impressive body of work has introduced geographic realism Licensee MDPI, Basel, Switzerland. to epidemiological simulators [5,22,30,31], only a fraction of readers encountering these This article is an open access article studies have sufficient familiarity with the software employed to fully appreciate key distributed under the terms and mechanics and assumptions. Here, we illustrate an epidemiological application of HexSim, conditions of the Creative Commons a simulation model development framework from landscape ecology. HexSim emphasizes Attribution (CC BY) license (https:// generality, flexibility, and accessibility; models constructed within the program may range creativecommons.org/licenses/by/ from non-spatial to spatial, mathematical to individual-based, and from probabilistic to 4.0/). Land 2021, 10, 438. https://doi.org/10.3390/land10040438 https://www.mdpi.com/journal/land Land 2021, 10, x FOR PEER REVIEW 2 of 13 Land 2021, 10, 438 2 of 13 probabilistic to mechanistic [16]. We believe HexSim offers epidemiologists a new devel- mechanisticopment platform [16]. We that believe can help HexSim balance offers simplicity epidemiologists and transparency a new development with nuance platform and re- thatalism. can help balance simplicity and transparency with nuance and realism. ManyMany mathematicalmathematical andand simulationsimulation modelsmodels ofof COVID-19COVID-19 havehave recentlyrecently beenbeen devel-devel- opedoped [[3232––3737],], butbut few are spatially spatially explicit explicit [29,38 [29,38].]. The The principal principal focus focus of ofthese these models models has hasbeen been to forecast to forecast rates rates of infection of infection and mortality. and mortality. But these But less these spatially less spatially informed informed models modelshave limited have limitedability to ability capture to capture disease disease dynamics dynamics or intervention or intervention efforts effortsthat vary that signifi- vary significantlycantly from place from placeto place. to place. Here, Here,we use we COVID use COVID-19-19 as a system as a system for exploring for exploring how public how publichealth healthexpectations expectations might mightchange change as geographic as geographic information information is introduced is introduced to forecasting to fore- castingmodels. models. To this Toend, this our end, study our uses study both uses non both-spatial non-spatial and spatial and SIRD spatial models SIRD modelsto contrast to contrastthe forecasted the forecasted benefits benefits of hypothetical of hypothetical vaccine vaccine strategies. strategies. We begin We begin by describing by describing our ourmodeling modeling system, system, then then demonstrate demonstrate that that it itcan can approximate approximate observed patterns ofof viralviral spreadspread inin Oregon,Oregon, andand finallyfinally employemploy thethe tooltool toto explore explore differences differences between between spatiallyspatially nuancednuanced vaccination vaccination deployment deployment strategies. strategies. 2.2. MaterialsMaterials andand MethodsMethods WeWe selected selected thethe statestate of of Oregon, Oregon, USA USA as as a a case case study study location location (Figure (Figure1 ).1) Oregon,. Oregon, locatedlocated on on the the US US west west coast, coast, is 25is 25 million million ha ha in areain area and and has ahas population a population size ofsize 4.2 of mil- 4.2 lion.million We. developedWe developed our spatialour spatial COVID COVID simulation simulation within within the HexSimthe HexSim modeling modeling environ- envi- mentronment [16]. [ HexSim16]. HexSim models models typically typically follow follow every every individual individual in one in orone more or more populations popula- astions they as transition they transition through through a complex a complex sequence sequence of life of history life history events events [39]. [39]. In contrast, In contrast, we designedwe designed our COVIDour COVID simulator simulator as a spatialas a spatial compartment compartment model model in which in which individuals individuals are onlyare on usedly used for tabulatingfor tabulating the the number number of of susceptible, susceptible, infected, infected, recovered, recovered, and and deceased deceased presentpresent at at specific specific locations locations throughout throughout the the landscape landscape [ 40[40,41].,41]. Compartment Compartment models models have have beenbeen frequently frequently used used to to improve improve model model accuracy accuracy while while minimizing minimizing complexity complexity [ 42[42–44–44].]. FigureFigure 1. 1.The The state state of of Oregon, Oregon, illustrating illustrating the the locations locations of of 36 36 counties counties (shades (shades of gray)of gray) and and the relativethe rela- tive size of districts (red) and regions (blue). size of districts (red) and regions (blue). HexSimHexSim (www.hexsim.net(www.hexsim.net accessed accessed onon 24 24 March March 2021) 2021) is is an an established established application application thatthat has has contributed contributed totoa a largelarge numbernumber ofof researchresearch papers,papers, primarilyprimarily inin thethe fieldsfields ofof land-land- scapescape ecologyecology andand conservationconservation biology.biology. BecauseBecause HexSimHexSim doesdoes notnot imposeimpose aa fixed fixed set set of of requiredrequired parameters, parameters, model model designs designs may may reflect reflect data data availability. availability. Though Though it it is is well-suited well-suited forfor exploring exploring theory theory [16[16,45,46],45,46],, HexSim HexSim isis frequentlyfrequently used used inin appliedapplied studiesstudies suchsuch asas pop-pop- ulationulation viabilityviability analysesanalyses [47[47].]. HexSimHexSim simulationssimulations cancan includeinclude oneone or or more more species species and and areare built built around around user-specified user-specified event event sequences. sequences. ItIt isis straightforwardstraightforward toto developdevelop species–species– landscapelandscape and and species–species species–species interactions,
Details
-
File Typepdf
-
Upload Time-
-
Content LanguagesEnglish
-
Upload UserAnonymous/Not logged-in
-
File Pages13 Page
-
File Size-