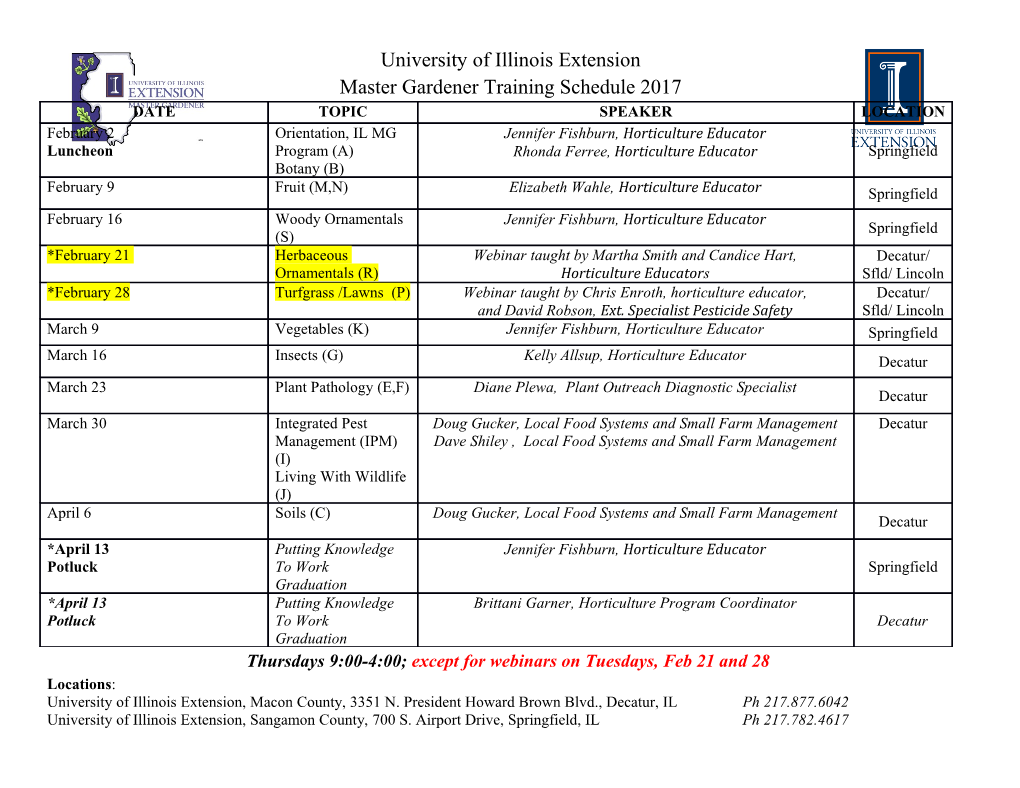
Personalized Recommendation in Social Network Sites A DISSERTATION SUBMITTED TO THE FACULTY OF THE GRADUATE SCHOOL OF THE UNIVERSITY OF MINNESOTA BY Jilin Chen IN PARTIAL FULFILLMENT OF THE REQUIREMENTS FOR THE DEGREE OF DOCTOR OF PHILOSOPHY Joseph Konstan and John Riedl, advisors September, 2011 © Jilin Chen 2011 Acknowledgements I would like to thank: • Joseph Konstan and John Riedl, for their sagacious guidance and advice • GroupLens, for providing me a friendly and intellectually rich environment • Ed Chi, for his supportive mentorship and rich intellectual contribution • Researchers and fellow interns at IBM Research and Palo Alto Research Center during my internships, for their insightful input and generous friendship • My family, for their care, support, and encouragement i Abstract Social network sites have experienced an explosion in both the number of users and the amount of user-contributed content in recent years. Today, millions of people visit Facebook, Twitter and the like to keep up with friends, to engage in random chatter, and to share and consume photos, news, useful tips and fun stories. Many active users of social network sites are, however, constantly troubled by information overload – there are too many other people to interact with and too much content to read. As a result, the difficulty in finding the right people and content to focus on has been identified as a key challenge for social network sites. In this thesis we seek to meet the challenge by designing three personalized recommender systems. The first system is a people recommender, which helps users find potential friends to connect with on social network sites; the second system is an information recommender, which helps users find interesting pieces of information from their online social network; the third system is a conversation recommender, which helps users find interesting conversations happening around their online social network. In designing the recommender systems, we adapt algorithms from related domains and create new algorithms. We refine these algorithms through offline data analysis. We then design and improve user interfaces of the recommender systems through participatory design. With the full recommender systems designed and implemented, we deploy these systems to real users of social network sites, and evaluate a variety of recommender algorithms online through user studies. Through these user studies we not only provide qualitative and quantitative comparisons of recommender algorithms, but also provide broader insights for designing recommender systems on social network sites. ii Table of Contents List of Tables...................................................................................................................v List of Figures ................................................................................................................vi Chapter 1: Introduction....................................................................................................1 1.1 Research Methodology...........................................................................................4 1.2 Thesis Overview ....................................................................................................6 Chapter 2: Related Recommendation Techniques ............................................................8 2.1 Recommendation Techniques: An Overview..........................................................8 2.2 Recommenders and Intelligent Interfaces on Social Network Sites.......................10 2.3 Recommendation Techniques in this Work ..........................................................11 Chapter 3: Recommending People .................................................................................14 3.1 Background and Research Goals ..........................................................................14 3.2 The IBM Beehive Platform ..................................................................................17 3.3 Recommender Algorithms ...................................................................................19 3.3.1 Algorithm 1: Content Matching.....................................................................19 3.3.2 Algorithm 2: Content-plus-Link (CplusL) .....................................................21 3.3.3 Algorithm 3: Friend-of-Friend (FoF) .............................................................22 3.3.4 Algorithm 4: SONAR....................................................................................23 3.4 Study I: Personalized Survey................................................................................24 3.4.1 Methodology.................................................................................................24 3.4.2 Results ..........................................................................................................27 3.5 Study II: Controlled Field Study...........................................................................34 3.5.1 Methodology.................................................................................................35 3.5.2 Results ..........................................................................................................36 3.6 Discussion ...........................................................................................................39 3.6.1 Understanding Algorithm Effectiveness ........................................................39 3.6.2 People Recommendation beyond Beehive .....................................................40 Chapter 4: Recommending Information .........................................................................42 4.1 Background and Research Goals ..........................................................................42 4.2 Information Production and Gathering on Twitter ................................................45 4.3 Recommender Design..........................................................................................46 4.3.1 Dimension 1: Selecting the Candidate Set .....................................................47 4.3.2 Dimension 2: Ranking URLs Using Topic Relevance....................................49 4.3.3 Dimension 3: Ranking URLs Using Social Voting ........................................52 4.3.4 Recommender Algorithms.............................................................................53 4.4 Pilot Interviews....................................................................................................55 4.4.1 Methodology.................................................................................................55 4.4.2 Results ..........................................................................................................57 4.5 Controlled Field Study.........................................................................................59 4.5.1 Methodology.................................................................................................60 4.5.2 Results ..........................................................................................................61 4.6 Discussion ...........................................................................................................66 4.6.1 Understanding the Effectiveness of Our Approach ........................................66 iii 4.6.2 Generalizability of Our Approach..................................................................67 4.6.3 Distinguishing Algorithms: Relevance vs. Serendipity ..................................68 Chapter 5: Recommending Conversation.......................................................................72 5.1 Background and Research Goals ..........................................................................72 5.2 Conversations on Twitter .....................................................................................74 5.3 Recommender Design..........................................................................................76 5.3.1 Factor 1: Thread Length ................................................................................77 5.3.2 Factor 2: Topic Relevance.............................................................................78 5.3.3 Factor 3: Tie-Strength ...................................................................................78 5.3.4 Recommender Algorithms.............................................................................80 5.3.5 Preliminary Explorations...............................................................................81 5.4 User Study...........................................................................................................83 5.4.1 Pre-Survey ....................................................................................................83 5.4.2 Main Study....................................................................................................83 5.4.3 Post-Survey...................................................................................................84 5.5 Results.................................................................................................................86 5.5.1 Pre-Survey ....................................................................................................86 5.5.2 Main Study....................................................................................................90 5.5.3 Post-Survey...................................................................................................93 5.5.4 Summary of Results ......................................................................................95
Details
-
File Typepdf
-
Upload Time-
-
Content LanguagesEnglish
-
Upload UserAnonymous/Not logged-in
-
File Pages122 Page
-
File Size-