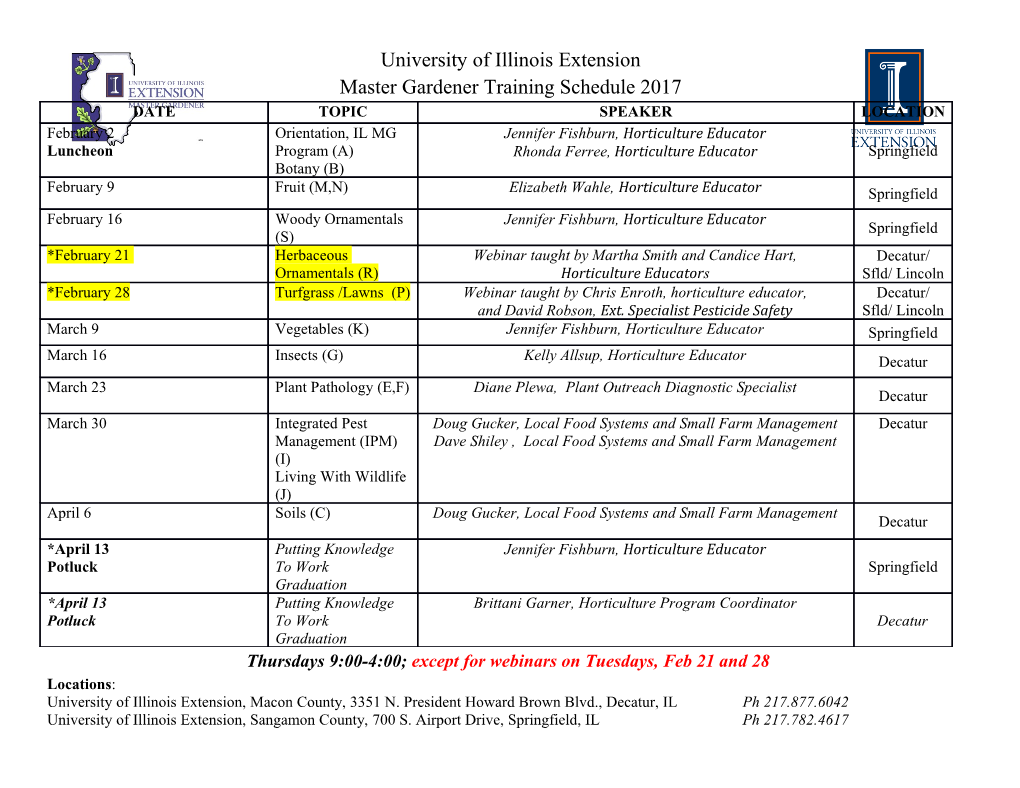
Modified slash Birnbaum-Saunders distribution Jimmy Reyes∗ , Filidor Vilcay, Diego I. Gallardoz and H´ectorW. G´omezx{ Abstract In this paper, we introduce an extension for the Birnbaum-Saunders (BS) distribution based on the modified slash (MS) distribution pro- posed by [12]. This new family of BS type distributions is obtained by replacing the usual normal distribution with the quotient of two independent random variables, one being a normal distribution in the numerator and the power of a exponential of parameter equal to two at the denominator. The resulting distribution is an extension of the BS distribution that has greater kurtosis values than the usual BS distri- bution and the slash Birnbaum-Saunders (SBS) distribution (see [7]). Moments and some properties are derived for the new distribution. Also, we draw inferences by the method of moments and maximum likelihood. A real data application is presented where the model fit- ting is implemented by using maximum likelihood estimation producing better results than the classic BS model and slash BS model. Keywords: Birnbaum-Saunders distribution, Modified slash distribution, Mo- ments; Kurtosis, EM-algorithm. 2000 AMS Classification: 60E05 1. Introduction The Birnbaum Saunders (BS) distribution (see [3] and [4]) was derived to model the material fatigue failure time process, and has been widely applied in reliability and fatigue studies. Extensive work has been done on the BS model with regard to its properties, inferences and applications. Generalizations of the BS distribution have been proposed by many authors; see for example, [6], [15], [7] and [2] among others, which allow obtaining a high degree of flexibility for this distribution. From an analytical point of view, the attention for the BS distribution is due to its attractive properties and its relationship with the normal distribution. ∗Departamento de Matem´aticas,Facultad de Ciencias B´asicas,Universidad de Antofagasta, Antofagasta, Chile, Email: [email protected] yDepartamento de Estat´ıstica, IMECC, Universidade Estadual de Campinas, Campinas, Brasil, Email: [email protected] zDepartamento de Matem´aticas,Facultad de Ciencias B´asicas,Universidad de Antofagasta, Antofagasta, Chile, Email: [email protected] xDepartamento de Matem´aticas,Facultad de Ciencias B´asicas,Universidad de Antofagasta, Antofagasta, Chile, Email: [email protected] {Corresponding Author. 2 A random variable T follows a Birnbaum-Saunders distribution with shape pa- rameter α > 0 and scale parameter β > 0, usually denoted by T ∼ BS(α; β). The random variable T can be represented as ! r( ) 2 α α 2 (1.1) T = β Z + Z + 1 2 2 where Z ∼ N(0; 1). The resulting probability density function (pdf) of T is − 3 t 2 (t + β) (1.2) fT (t; α; β) = ϕ(at(α; β)) 1 ; t > 0; 2αβ 2 p p where ϕ(·) is the pdf of the N(0; 1) distribution and at(α; β) = ( t/β − β=t)/α. The BS distribution proposed by [3] may not be suitable to represent data con- taining outlying observations due to its close dependence on the normal distribu- tion. One way to overcome this problem, is to exploit the relationship between the BS and normal distributions to obtain a generalization of BS distributions based on the modified slash (MS) distribution in place of the normal one, which possesses heavier tails than the normal. The MS distribution was proposed recently by [12], which incorporates more kurtosis. This distribution is a type scale mixture of the normal distribution, in which the mixing distribution depends on the exponential distribution. The resulting distribution, called the MS distribution, has heavier tails than the normal and slash-normal distributions. Next, we present a brief review of the MS distribution and some of its properties. A random variable X has a MS distribution (see [12]) if it can be represented by Z (1.3) X = 1 ; V q where Z ∼ N(0; 1) is independent of V ∼ exp(2). The pdf of X can be expressed as Z 1 q −2vq (1.4) fX (x) = 2q v e ϕ(xv) dv; x 2 R: 0 An interesting special case is obtained for q = 1, which is the canonic modified slash distribution, the distribution of the ratio of two independent random variables, one being the standard normal and the other an exp(2) distribution whose pdf can be written in a simple way as follows 8 h − i x 2 <> 2 p1 − 2e Φ(− 2 ) if x =6 0; x2 2π jxj jxj (1.5) fX (x) = > : − 1 (8π) 2 if x = 0; where Φ(·) denotes the cdf of the standard normal distribution. On the other hand, when q tends to 1, the resulting distribution is a normal one. The aim of this paper is to provide a generalized Birnbaum-Saunders distri- bution based on modified slash distribution. This extension is based mainly on the work of [12] and [7]. The resulting distribution, which we call modified slash Birnbaum-Saunders (MSBS) distribution, provides flexible thick-tailed distribu- tions which can be used for robust estimation of parameters. 3 The rest of this paper is organized as follows.In Section 2, we define the BS distribution based on MS distributions and discuss some of its properties. Specif- ically, we derive its probability density function, and moments. In Section 3, we discuss estimation methods based on the modified moment estimators and the maximum likelihood estimation. In Section 4, we provide an illustrative example that displays the usefulness of the generalized BS distributions for fitting a real data set that has been analyzed before in the literature. Finally, some concluding remarks are made in Section 5. 2. Proposed distribution Analogous to the BS distribution of [2] and [7], we can define an extension of the Birnbaum-Saunders distribution based on the MS distribution by considering the following stochastic representation of T : ! r( ) 2 α α 2 (2.1) T = β X + X + 1 2 2 where X ∼ MS(0; 1; q) given in (1.3). So, the random variable T is said to have a modified slash Birnbaum-Saunders (MSBS) distribution with parameters α, β and q, and will be denoted by T ∼ MSBS(α; β; q). The stochastic representation in (2.1) is useful for the simulation of data and also for implementing the EM- algorithm for ML estimation of parameters in MSBS models, as will be discussed later. 2.1. Density function. In this part we derive the pdf for the modified slash Birnbaum-Saunders distribution, which is based on the modified slash distribution 2.1. Proposition. Let T ∼ MSBS(α; β; q). Then, the pdf of T is Z −3=2 1 t (t + β)q q (2.2) f (t) = vqe−2v ϕ(v a (α; β)) dv; t > 0; T 1=2 t αβ 0 where ϕ(·) is the pdf of the N(0; 1) distribution. Proof. Let be X ∼ MS(0; 1; q) with its pdf given in (1.4). The result comes easily from the stochastic representation in (2.1) and using the change of variable theorem 2: The next special case q = 1 is derived easily from Proposition 2.1. In fact, the pdf of T , given in (2.2), reduces to the following expression 8 −3=2 R <> t (t+β) 1 −2v 6 αβ1=2 0 ve ϕ(vat(α; β)) dv; if t = β; (2.3) fT (t) = > : −1=2 1 (8π) αβ ; if t = β: On the other hand, when q tends to 1, we simply obtain the ordinary BS distri- bution, as will be seen in the next proposition. Figure 1 shows the pdf of the MSBS, SBS and BS distributions for different values of α and β, with q = 2. 4 MSBS(0.5,1,2) SBS(0.5,1,2) BS(0.5,1) Density 0.0 0.2 0.4 0.6 0.8 1.0 1.2 0 1 2 3 4 t Figure 1. Plots of densities of the MSBS (black line), SBS (red line) and BS (blue line) distributions, for α = 0:5, β = 1 and q = 2 2.2. Properties of the MSBS distribution. 2.2. Proposition. Let T ∼ MSBS(α; β; q). Then, a) aT ∼ MSBS(α; aβ; q) for all a > 0; b) T −1 ∼ MSBS(α; β−1; q); c) limq!1fT (t; α; β; q) = fT (t; α; β), where fT (:; α; β) is the pdf of a BS(α; β) distribution; d) The conditional distribution( of T), given V = v, is the BS distribution. 1=q That is, T j(V = v) ∼ BS αv;q; β , where αv;q = α=v . Proof. Parts (a) and (b) are directly obtained using the change of variable the- orem. Part (c) is obtained in the same way since the MS distribution tends to the normal distribution. Finally, Part (d) is obtained easily from (1.3) and (2.1). 2 As is required in the study and development of any statistical analysis, the moments are important, especially in applied work. Some of the most important features and characteristics of a distribution can be studied through moments such as skewness and kurtosis parameters, which depend on the gamma function, Γ(·), defined for fx 2 R : x =6 0; −1; −2;:::g, which in turn are likely to be important in a number of applications. The following results are related to them. 5 − 2k q q−2k ∼ q 2.3. Proposition. Let T MSBS(α; β; q) and B2k;q = 2 Γ( q ), q > 2k, k = 1; : : : ; r. If E[X2r] exists and is finite. Then, r ( ) y ( ) ( ) h i X 2r X y α 2(r+s−y) (2.4) E[T r] = βr E X2(r+s−y) and 2y s 2 y=0 s=0 1 (2.5) E[T −r] = E[T r]; β2r E 2(r+s−y) (2(r+s−y))! where [X ] = 2 2(r+s−y)(r+s−y)! B2(r+s−y);q, y = 0; 1; : : : ; r.
Details
-
File Typepdf
-
Upload Time-
-
Content LanguagesEnglish
-
Upload UserAnonymous/Not logged-in
-
File Pages17 Page
-
File Size-