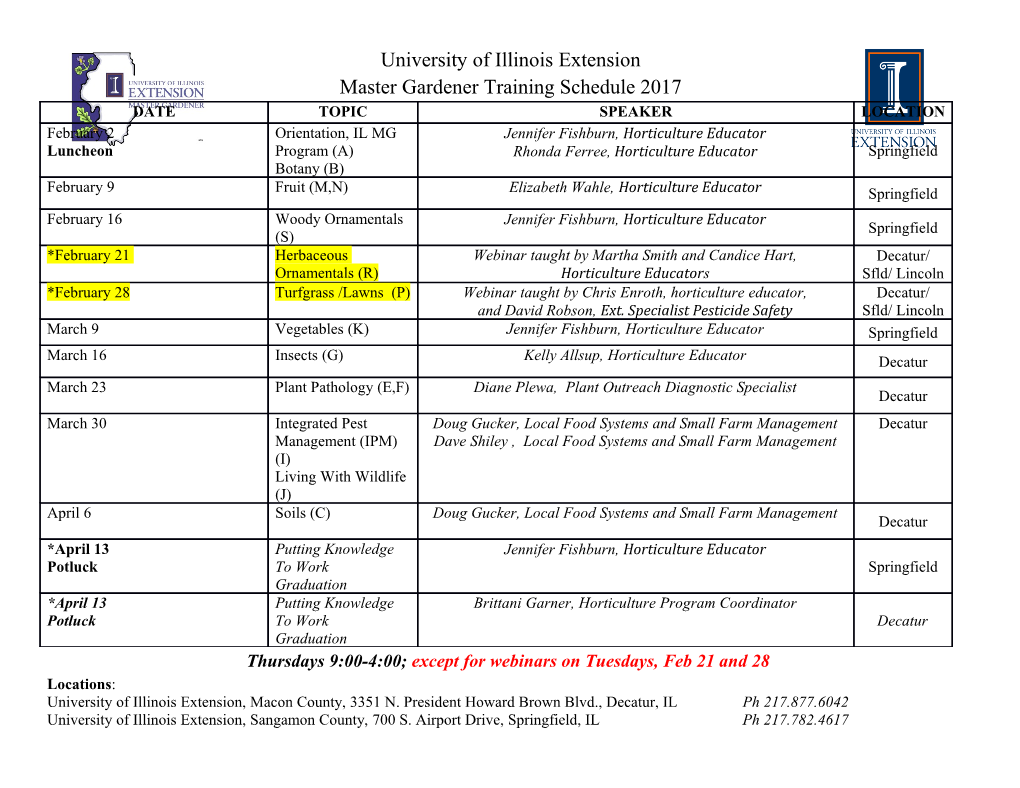
UC Irvine UC Irvine Electronic Theses and Dissertations Title Improving Efficacy of Support Groups in Online Environments Permalink https://escholarship.org/uc/item/12q608h5 Author Esmaeeli, Ali Publication Date 2020 License https://creativecommons.org/licenses/by/4.0/ 4.0 Peer reviewed|Thesis/dissertation eScholarship.org Powered by the California Digital Library University of California UNIVERSITY OF CALIFORNIA, IRVINE Improving Efficacy of Support Groups in Online Environments DISSERTATION submitted in partial satisfaction of the requirements for the degree of DOCTOR OF PHILOSOPHY in Management by Ali Esmaeeli Dissertation Committee: Professor L. Robin Keller, Co-chair Professor Cornelia (Connie) Pechmann, Co-chair Associate Professor John Turner 2020 © 2020 Ali Esmaeeli DEDICATION To My supportive family who value diligence and wisdom ii TABLE OF CONTENTS Page LIST OF FIGURES v LIST OF TABLES vi ACKNOWLEDGMENTS vii VITA viii ABSTRACT OF THE DISSERTATION ix INTRODUCTION 1 CHAPTER 1: The Effects of Buddy Systems in Online Support Groups on Members’ Goal Attainment and Interactions 4 Theoretical Framework and Hypotheses 7 Buddy Systems and Hypotheses Development 7 Measuring Tie Strength 13 Research Methods 16 Setting 16 Dependent and Independent Variables 18 Analyses Approach 21 Empirical Results 23 Descriptive Statistics 23 Test of H1 25 Test of H2 26 Test of H3 29 Test of H4 30 Test of H5 (Mediation Test) 34 Discussion 36 Summary and Conclusion 36 Limitations 37 CHAPTER 2: Adding a Chatbot to Online Support Groups: The Natural Language Understanding Component 39 Context 43 Setting 43 Roles of the chatbot 44 Design 45 Chatbot Design 45 Intents 47 Natural Language Understanding (NLU) Component 51 iii Result 53 Descriptive statistics 53 Intent classification 54 General Discussion 58 Findings 58 Limitations and next steps 60 REFERENCES 62 APPENDIX: Source Code of the Bot Intent Classifier 72 iv LIST OF FIGURES Page Figure 1.1 The effect of buddy activity on interactions and goal attainment 13 Figure 1.2 Member’s goal attainment 25 Figure 1.3 Tie from member’s buddy to the member 28 Figure 1.4 Tie to the buddy versus tie to a non-buddy (mean) 30 Figure 1.5 Tie from a member to other people in the group 33 Figure 2.1 Chatbot components 46 Figure 2.2 Confusion matrix for the intent classifier (N=4034) 56 v LIST OF TABLES Page Table 1.1 Studies of offline buddy systems 9 Table 1.2 Statistics for tie strength measures 24 Table 1.3 Summary of findings for hypothesis tests 35 Table 2.1 Comparison between different categories of software bots 42 Table 2.2 Intents about using NRT correctly 48 Table 2.3 Intents about efficacy of NRT 49 Table 2.4 Intents about negative emotions 49 Table 2.5 Intents about positive actions 50 Table 2.6 Non-triggering intents 51 Table 2.7 Intent frequency of records (N=16136) 54 Table 2.8 Classification report for the test dataset (N=4034) 57 vi ACKNOWLEDGMENTS I would like to express my deepest appreciation to my advisors, Professor L. Robin Keller and Professor Cornelia (Connie) Pechmann, for their unwavering support and guidance throughout this entire process. Thanks also to Professor John Turner for his valuable insights and suggestions that helped me in my research. I am extremely grateful to my wife, Marzieh, for being a great companion throughout this journey. My success would not have been possible without her support. This research was supported by National Cancer Institute Grant R01CA204356-04. vii VITA Ali Esmaeeli 2007 B.S. in Information Technology Engineering, Amirkabir University of Technology 2010 M.S. in Information Technology Engineering, Amirkabir University of Technology 2020 Ph.D. in Management, University of California, Irvine PUBLICATIONS A. Esmaeeli, C. Pechmann, and J.J. Prochaska, Examination of a Homophily-based Buddy System for Online Support Groups: Relationships with Tie Strength and Goal Attainment, Revision requested at Journal of Interactive Marketing M. Razzazi and A. Esmaeeli, Balance Allocation Mechanism: An Optimal Mechanism for Multiple Keywords Sponsored Search Auctions, Information Sciences, vol. 262, March 2014 http://dx.doi.org/10.1016/j.ins.2013.08.033, pp. 190-214 A. Esmaeeli and H.R. Shahriari, Privacy Protection of Grid Service Requesters through Distributed Attribute Based Access Control Model, Proc. of 5th Int. Conf. on Advances in Grid and Pervasive Computing, Lecture Notes in Computer Science, Springer-Verlag, Taiwan, May 2010, pp. 573-582 A. Esmaeeli and M. Shajari, MVPayword: Secure and Efficient Payword-Based Micropayment Scheme, Proc. of 2nd Int. Conf. on the Applications of Digital Information and Web Technologies, London, Aug. 2009, pp. 609-614 viii ABSTRACT OF THE DISSERTATION Improving Efficacy of Support Groups in Online Environments by Ali Esmaeeli Doctor of Philosophy in Management University of California, Irvine, 2020 Professor L. Robin Keller and Professor Cornelia (Connie) Pechmann, Co-chairs In this research I evaluated two strategies for improving performance of online support groups. The first strategy was to use a buddy system in online support groups. This system involves pairing demographically similar members of a group to serve as each other’s buddies. Analyses of real online support groups indicated that members whose only difference with other members is in having a buddy who is more active rather than less active in the group are more engaged in the group and have a higher chance of attaining their goal for which they joined the group. Analysis also showed that members help their buddies more than they help other group members. Results are robust across four measures of tie strength, including contact frequency, reciprocity, and two measures of contact length. Overall, results suggest that managers can use the buddy system in online support groups as an effective method to drive group engagement and increase the support provided and goal attainment. The second strategy was to add a chatbot to the online support groups to provide members with additional informational and motivational support. The design of the chatbot which can respond to members’ messages based on their content is presented here. ix The chatbot has a natural language understanding component which can identify intent of messages out of 26 possible intents related to smoking cessation which is the main context of the online support groups studied here. The chatbot is supposed to respond to messages with 25 intents called triggering intents and ignore 1 intent which is non-triggering intent. Triggering intents account for less than half of the total messages. The bot is intentionally designed to have higher precision than recall for triggering intents. Precision for triggering intents is the probability of identifying the intent correctly if the message is identified as a triggering intent. Recall for triggering intents is the percentage of triggering messages which are identified with correct intent. The chatbot has the precision of 81% and the recall of 44% for triggering intents. Separate randomized control trial experiments are required to evaluate the overall performance of the chatbot in online support groups. x INTRODUCTION Online social networks are very popular, accounting for about one third of the time that people spend online (Buckle 2016). Users spend more than two hours per day on average on online social networks (Mander 2017). This availability and popularity has created a framework for new and innovative applications of online social networks. Examples of these applications are online support groups for health behavior change (Barrera et al. 2002; Cavallo et al. 2012; Maher et al. 2014; Pechmann et al. 2017; Setoyama, Yamazaki and Namayama 2011; Turner-McGrievy and Tate 2013) and collaborative learning (Cho et al. 2007; Wasko and Faraj 2005). Online support groups seek to provide members with social support so that they achieve their goals. These groups attract people with specific goals, interests, or needs. Online support groups are also important for firms. Firms may benefit from online support groups by gaining access to market research information (Bickart and Schindler 2001; Kozinets 2002; Divakaran et al. 2017; Gruner, Homburg and Lukas 2014), charging for the online services offered (Armstrong and Hagel III 1996), direct selling (Naylor, Lamberton and West 2012), advertising to the members (Armstrong and Hagel III 1996), and word-of-mouth promotions (Brown, Broderick and Lee 2007). The main application of online support groups that I focused my research on is in the field of health behavior change. Online support groups seek to provide peer social support for health behavior change by ensuring positive and lasting interactions between the online group members (Moorhead et al. 2013; Portnoy et al. 2008). Examples of these applications include smoking cessation (Cobb, Graham and Abrams 2010; Graham et al. 2017; Pechmann et al. 2015; Pechmann et al. 2017), diabetes control (Barrera et al. 2002), 1 breast cancer support (Setoyama, Yamazaki and Namayama 2011), weight loss (Bradford, Grier and Henderson 2017; Brindal et al. 2012; Hwang et al. 2010; Napolitano et al. 2013; Turner-McGrievy and Tate 2013; Parkinson et al. 2017), physical activity promotion (Cavallo et al. 2012; Foster et al. 2010; Valle et al. 2013), and health information provision (Kuwata et al. 2010; Freyne et al. 2010). Collaborative learning is another important domain that benefits from online social networks. Learning involves the process of knowledge acquisition from social relations and communications with other people (Brown and Duguid 1991). Collaborative learning communities provide knowledge sharing as well as social support for educational attainment (Cho et al. 2007; Haythornthwaite 2002a; Wasko and Faraj 2005). Engagement takes place through computer-supported collaborative learning (Cho et al. 2007) and online discussion forums (Wasko and Faraj 2005). Social interaction is a key element in these collaborative learning environments (Kreijns, Kirschner and Jochems 2003). The basic requirement for success in online groups is to have active members who provide social support to each other (Arguello et al.
Details
-
File Typepdf
-
Upload Time-
-
Content LanguagesEnglish
-
Upload UserAnonymous/Not logged-in
-
File Pages91 Page
-
File Size-