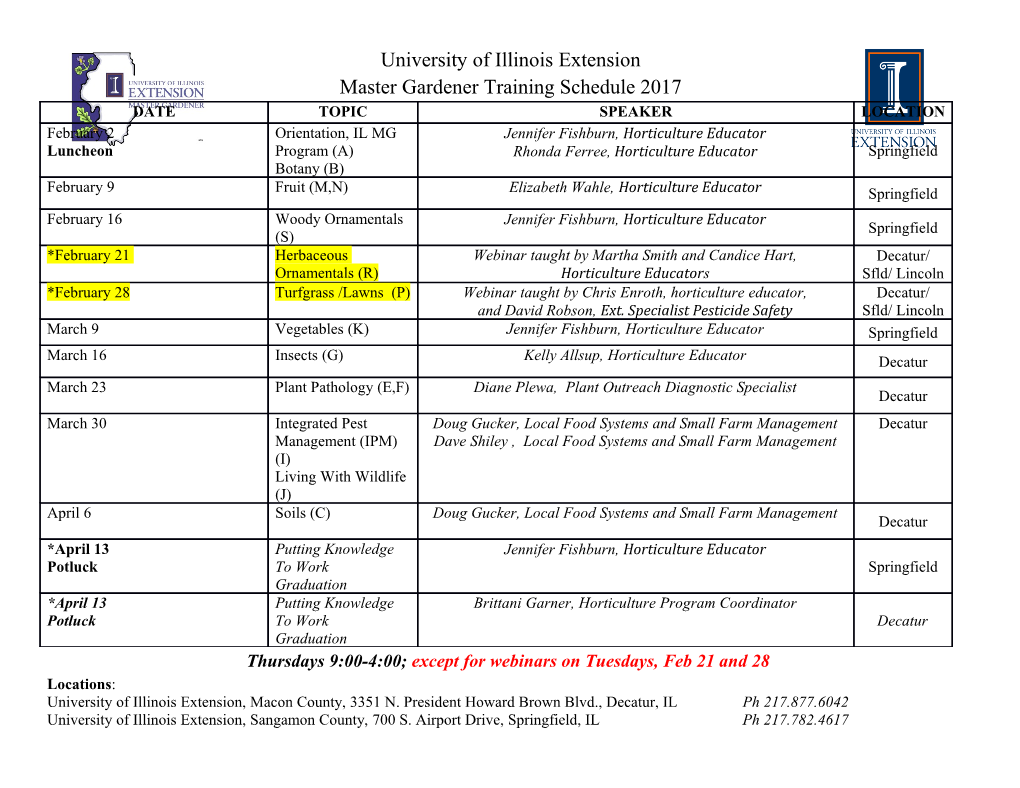
Self-disclosure topic model for Twitter conversations JinYeong Bak Chin-Yew Lin Alice Oh Department of Computer Science Microsoft Research Asia Department of Computer Science KAIST Beijing 100080, P.R. China KAIST Daejeon, South Korea [email protected] Daejeon, South Korea [email protected] [email protected] Abstract is social support from others (Wills, 1985; Der- lega et al., 1993), shown also in online social net- Self-disclosure, the act of revealing one- works (OSN) such as Twitter (Kim et al., 2012). self to others, is an important social be- Receiving social support would then lead the user havior that contributes positively to inti- to be more active on OSN (Steinfield et al., 2008; macy and social support from others. It Trepte and Reinecke, 2013). In this paper, we seek is a natural behavior, and social scien- to understand this important social behavior using tists have carried out numerous quantita- a large-scale Twitter conversation data, automati- tive analyses of it through manual tagging cally classifying the level of self-disclosure using and survey questionnaires. Recently, the machine learning and correlating the patterns with flood of data from online social networks subsequent OSN usage. (OSN) offers a practical way to observe Twitter conversation data, explained in more de- and analyze self-disclosure behavior at an tail in section 4.1, enable a significantly larger unprecedented scale. The challenge with scale study of naturally-occurring self-disclosure such analysis is that OSN data come with behavior, compared to traditional social science no annotations, and it would be impos- studies. One challenge of such large scale study, sible to manually annotate the data for a though, remains in the lack of labeled ground- quantitative analysis of self-disclosure. As truth data of self-disclosure level. That is, a solution, we propose a semi-supervised naturally-occurring Twitter conversations do not machine learning approach, using a vari- come tagged with the level of self-disclosure in ant of latent Dirichlet allocation for au- each conversation. To overcome that challenge, tomatically classifying self-disclosure in a we propose a semi-supervised machine learning massive dataset of Twitter conversations. approach using probabilistic topic modeling. Our For measuring the accuracy of our model, self-disclosure topic model (SDTM) assumes that we manually annotate a small subset of self-disclosure behavior can be modeled using a our dataset, and we show that our model combination of simple linguistic features (e.g., shows significantly higher accuracy and pronouns) with automatically discovered seman- F-measure than various other methods. tic themes (i.e., topics). For instance, an utterance With the results our model, we uncover “I am finally through with this disastrous relation- a positive and significant relationship be- ship” uses a first-person pronoun and contains a tween self-disclosure and online conversa- topic about personal relationships. tion frequency over time. In comparison with various other models, SDTM shows the highest accuracy, and the result- 1 Introduction ing self-disclosure patterns of the users are cor- related significantly with their future OSN usage. Self-disclosure is an important and pervasive so- Our contributions to the research community in- cial behavior. People disclose personal informa- clude the following: tion about themselves to improve and maintain relationships (Jourard, 1971; Joinson and Paine, We present a topic model that explicitly in- • 2007). For example, when two people meet for cludes the level of self-disclosure in a conver- the first time, they disclose their names and in- sation using linguistic features and the latent terests. One positive outcome of self-disclosure semantic topics (Sec. 3). 42 Proceedings of the Joint Workshop on Social Dynamics and Personal Attributes in Social Media, pages 42–49, Baltimore, Maryland USA, 27 June 2014. c 2014 Association for Computational Linguistics We collect a large dataset of Twitter conver- 훾 훼 • sations over three years and annotate a small 휋 휃푙 subset with self-disclosure level (Sec. 4). 3 We compare the classification accuracy of 훽푙 푟 푧 푦 • SDTM with other models and show that it 휙푙 푤 ω 푥 performs the best (Sec. 5). 퐾푙 N 3 T C We correlate the self-disclosure patterns of 휆 • users and their subsequent OSN usage to show that there is a positive and significant Figure 1: Graphical model of SDTM relationship (Sec. 6). 2 Background self-disclosure for high accuracy in classifying the three levels of self-disclosure. In this section, we review literature on the relevant Self-disclosure and online social network: aspects of self-disclosure. According to social psychology, when someone Self-disclosure (SD) level: To quantitatively discloses about himself, he will receive social sup- analyze self-disclosure, researchers categorize port from those around him (Wills, 1985; Derlega self-disclosure language into three levels: G (gen- et al., 1993), and this pattern of self-disclosure eral) for no disclosure, M for medium disclosure, and social support was verified for Twitter con- and H for high disclosure (Vondracek and Von- versation data (Kim et al., 2012). Social support dracek, 1971; Barak and Gluck-Ofri, 2007). Ut- is a major motivation for active usage of social terances that contain general (non-sensitive) infor- networks services (SNS), and there are findings mation about the self or someone close (e.g., a that show self-disclosure on SNS has a positive family member) are categorized as M. Examples longitudinal effect on future SNS use (Trepte and are personal events, past history, or future plans. Reinecke, 2013; Ledbetter et al., 2011). While Utterances about age, occupation and hobbies are these previous studies focused on small, qualita- also included. Utterances that contain sensitive in- tive studies, we conduct a large-scale, machine formation about the self or someone close are cat- learning driven study to approach the question of egorized as H. Sensitive information includes per- self-disclosure behavior and SNS use. sonal characteristics, problematic behaviors, phys- ical appearance and wishful ideas. Generally, 3 Self-Disclosure Topic Model these are thoughts and information that one would generally keep as secrets to himself. All other This section describes our model, the self- utterances, those that do not contain information disclosure topic model (SDTM), for classifying about the self or someone close are categorized self-disclosure level and discovering topics for as G. Examples include gossip about celebrities or each self-disclosure level. factual discourse about current events. 3.1 Model Classifying self-disclosure level: Prior work on quantitatively analyzing self-disclosure has re- We make two important assumptions based on our lied on user surveys (Trepte and Reinecke, 2013; observations of the data. First, first-person pro- Ledbetter et al., 2011) or human annotation (Barak nouns (I, my, me) are good indicators for medium and Gluck-Ofri, 2007). These methods consume level of self-disclosure. For example, phrases such much time and effort, so they are not suitable for as ‘I live’ or ‘My age is’ occur in utterances that re- large-scale studies. In prior work closest to ours, veal personal information. Second, there are top- Bak et al. (2012) showed that a topic model can ics that occur much more frequently at a particular be used to identify self-disclosure, but that work SD level. For instance, topics such as physical applies a two-step process in which a basic topic appearance and mental health occur frequently at model is first applied to find the topics, and then level H, whereas topics such as birthday and hob- the topics are post-processed for binary classifica- bies occur frequently at level M. tion of self-disclosure. We improve upon this work Figure 1 illustrates the graphical model of by applying a single unified model of topics and SDTM and how these assumptions are embodied 43 Notation Description 1. For each level l G, M, H : For each topic∈ { k }1,...,Kl : G; M; H general; medium; high SD level l ∈ { l } { } Draw φk Dir(β ) C; T ; N Number of conversations; tweets; 2. For each conversation∼ c 1,...,C : words G ∈ { } (a) Draw θc Dir(α) G M H M ∼ K ; K ; K Number of topics for G; M; H (b) Draw θc Dir(α) c; ct Conversation; tweet in{ conversation} c (c) Draw θH ∼ Dir(α) c ∼ yct SD level of tweet ct, G or M/H (d) Draw πc Dir(γ) (e) For each message∼ t 1,...,T : rct SD level of tweet ct, M or H ∈ { } i. Observe first-person pronouns features xct zct Topic of tweet ct th ii. Draw ωct MaxEnt(xct, λ) wctn n word in tweet ct iii. Draw y ∼Bernoulli(ω ) ct ∼ ct λ Learned Maximum entropy parame- iv. If yct = 0 which is G level: G ters A. Draw zct Mult(θ ) ∼ c xct First-person pronouns features B. For each word n 1,...,N : Draw word w ∈ { Mult(φ}G ) !ct Distribution over SD level of tweet ct ctn zct Else which can be M or∼H level: πc SD level proportion of conversation c G M H A. Draw rct Mult(πc) θc ; θc ; θc Topic proportion of G; M; H in con- ∼ rct { } B. Draw zct Mult(θc ) versation c C. For each word∼ n 1,...,N : G M H ∈ { }rct φ ; φ ; φ Word distribution of G; M; H Draw word wctn Mult(φ ) ∼ zct α; γ Dirichlet prior for θ; {π } βG, βM ; βH Dirichlet prior for φG; φM ; φH ncl Number of tweets assigned SD level l Figure 2: Generative process of SDTM. in conversation c l nck Number of tweets assigned SD level l and topic k in conversation c l 3.3 Classifying M vs H levels nkv Number of instances of word v as- signed SD level l and topic k The second part of the classification, the M and the mctkv Number of instances of word v as- H level, is driven by informative priors with seed signed topic k in tweet ct words and seed trigrams.
Details
-
File Typepdf
-
Upload Time-
-
Content LanguagesEnglish
-
Upload UserAnonymous/Not logged-in
-
File Pages8 Page
-
File Size-