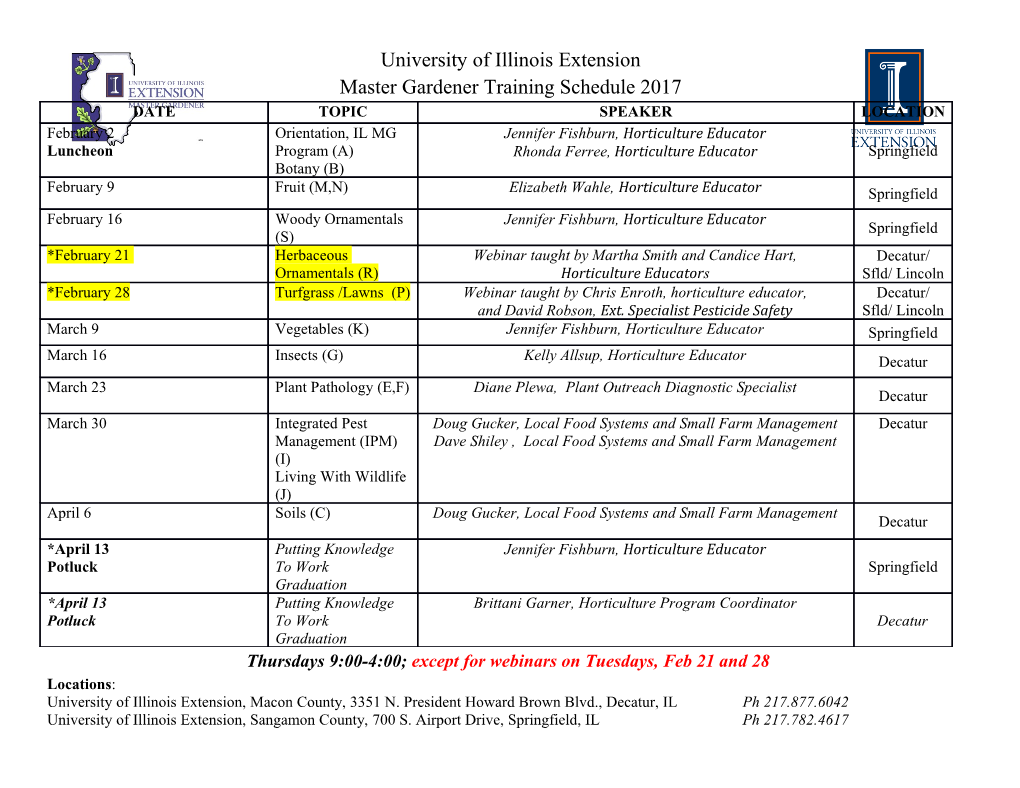
Bayesian Methods: Naïve Bayes Nicholas Ruozzi University of Texas at Dallas based on the slides of Vibhav Gogate Last Time • Parameter learning – Learning the parameter of a simple coin flipping model • Prior distributions • Posterior distributions • Today: more parameter learning and naïve Bayes 2 Maximum Likelihood Estimation (MLE) • Data: Observed set of 훼퐻 heads and 훼푇 tails • Hypothesis: Coin flips follow a binomial distribution • Learning: Find the “best” 휃 • MLE: Choose to maximize the likelihood (probability of D given 휃) 휃푀퐿퐸 = arg max 푝(퐷|휃) 휃 3 MAP Estimation • Choosing 휃 to maximize the posterior distribution is called maximum a posteriori (MAP) estimation 휃푀퐴푃 = arg max 푝(휃|퐷) 휃 • The only difference between 휃푀퐿퐸 and 휃푀퐴푃 is that one assumes a uniform prior (MLE) and the other allows an arbitrary prior 4 Sample Complexity • How many coin flips do we need in order to guarantee that our learned parameter does not differ too much from the true parameter (with high probability)? • Can use Chernoff bound (again!) – Suppose 푌1, … , 푌푁 are i.i.d. random variables taking values in {0, 1} such that 퐸푝 푌 = 푦. For 휖 > 0, 1 2 푝 푦 − 푌 ≥ 휖 ≤ 2푒−2푁휖 푁 5 Sample Complexity • How many coin flips do we need in order to guarantee that our learned parameter does not differ too much from the true parameter (with high probability)? • Can use Chernoff bound (again!) – For the coin flipping problem with 푋1, … , 푋푛 iid coin flips and 휖 > 0, 1 2 푝 휃 − 푋 ≥ 휖 ≤ 2푒−2푁휖 푡푟푢푒 푁 6 Sample Complexity • How many coin flips do we need in order to guarantee that our learned parameter does not differ too much from the true parameter (with high probability)? • Can use Chernoff bound (again!) – For the coin flipping problem with 푋1, … , 푋푛 iid coin flips and 휖 > 0, −2푁휖2 푝 휃푡푟푢푒 − 휃푀퐿퐸 ≥ 휖 ≤ 2푒 7 Sample Complexity • How many coin flips do we need in order to guarantee that our learned parameter does not differ too much from the true parameter (with high probability)? • Can use Chernoff bound (again!) – For the coin flipping problem with 푋1, … , 푋푛 iid coin flips and 휖 > 0, −2푁휖2 푝 휃푡푟푢푒 − 휃푀퐿퐸 ≥ 휖 ≤ 2푒 2 1 2 훿 ≥ 2푒−2푁휖 ⇒ 푁 ≥ ln 2휖2 훿 8 MLE for Gaussian Distributions • Two parameter distribution characterized by a mean and a variance 9 Some properties of Gaussians • Affine transformation (multiplying by scalar and adding a constant) are Gaussian – 푋 ~ 푁(휇, 휎2) – 푌 = 푎푋 + 푏 ⇒ 푌 ~ 푁(푎휇 + 푏, 푎2휎2) • Sum of Gaussians is Gaussian – 푋 ~ 푁(휇 , 2), 푌 ~ 푁(휇 , 2) 푋 휎푋 푌 휎푌 – 푍 = 푋 + 푌 ⇒ 푍 ~ 푁(휇 + 휇 , 2 + 2) 푋 푌 휎푋 휎푌 • Easy to differentiate, as we will see soon! 10 Learning a Gaussian • Collect data Exam Score – Hopefully, i.i.d. samples 0 85 – e.g., exam scores 1 95 2 100 • Learn parameters 3 12 … … – Mean: μ 99 89 – Variance: σ 11 MLE for Gaussian: • Probability of 푁 i.i.d. samples 퐷 = 푥(1), … , 푥(푁) 푁 2 푁 푥 푖 −휇 1 − 푝 퐷 휇, 휎 = ෑ 푒 2휎2 2 2휋휎 =1 • Log-likelihood of the data 푁 2 푁 푥 − 휇 ln 푝(퐷|휇, 휎) = − ln 2휋휎2 − 2 2휎2 =1 12 MLE for the Mean of a Gaussian 푁 2 휕 휕 푁 푥 − 휇 ln 푝(퐷|휇, 휎) = − ln 2휋휎2 − 휕휇 휕휇 2 2휎2 =1 푁 2 휕 푥 − 휇 = − 휕휇 2휎2 =1 푁 푥 − 휇 = − 휎2 =1 푁휇 − σ푁 푥 = =1 = 0 휎2 푁 1 휇 = 푥 푀퐿퐸 푁 =1 13 MLE for Variance 푁 2 휕 휕 푁 푥 − 휇 ln 푝(퐷|휇, 휎) = − ln 2휋휎2 − 휕휎 휕휎 2 2휎2 =1 푁 2 푁 휕 푥 − 휇 = − + − 휎 휕휎 2휎2 =1 푁 2 푁 푥 − 휇 = − + = 0 휎 휎3 =1 푁 1 2 휎2 = 푥 − 휇 푀퐿퐸 푁 푀퐿퐸 =1 14 Learning Gaussian parameters 푁 1 휇 = 푥 푀퐿퐸 푁 =1 푁 1 2 휎2 = 푥 − 휇 푀퐿퐸 푁 푀퐿퐸 =1 • MLE for the variance of a Gaussian is biased – Expected result of estimation is not true parameter! – Unbiased variance estimator 푁 1 2 휎2 = 푥 − 휇 푢푛푏푎푠푒푑 푁 − 1 푀퐿퐸 =1 15 Bayesian Categorization/Classification • Given features 푥 = (푥1, … , 푥푚) predict a label 푦 • If we had a joint distribution over 푥 and 푦, given 푥 we could find the label using MAP inference arg max 푝(푦|푥1, … , 푥푚) 푦 • Can compute this in exactly the same way that we did before using Bayes rule: 푝 푥1, … , 푥푚 푦 푝 푦 푝 푦 푥1, … , 푥푚 = 푝 푥1, … , 푥푚 16 Article Classification • Given a collection of news articles labeled by topic, goal is, given a new news article to predict its topic – One possible feature vector: • One feature for each word in the document, in order 푡ℎ – 푥 corresponds to the word – 푥 can take a different value for each word in the dictionary 17 Text Classification 18 Text Classification • 푥1, 푥2, … is sequence of words in document • The set of all possible features (and hence 푝(푦|푥)) is huge – Article at least 1000 words, 푥 = (푥1, … , 푥1000) 푡ℎ – 푥 represents word in document • Can be any word in the dictionary – at least 10,000 words – 10,0001000 = 104000 possible values – Atoms in Universe: ~1080 19 Bag of Words Model • Typically assume position in document doesn’t matter 푝 푋 = 푥 푌 = 푦 = 푝(푋푘 = 푥|푌 = 푦) – All positions have the same distribution – Ignores the order of words – Sounds like a bad assumption, but often works well! • Features – Set of all possible words and their corresponding frequencies (number of times it occurs in the document) 20 Bag of Words aardvark 0 about2 all 2 Africa 1 apple0 anxious 0 ... gas 1 ... oil 1 … Zaire 0 21 Need to Simplify Somehow • Even with the bag of words assumption, there are too many possible outcomes – Too many probabilities 푝(푥1, … , 푥푚|푦) • Can we assume some are the same? 푝(푥1, 푥2|푦 ) = 푝(푥1|푦) 푝(푥2|푦) – This is a conditional independence assumption 22 Conditional Independence • X is conditionally independent of Y given Z, if the probability distribution for X is independent of the value of Y, given the value of Z 푝 푋 푌, 푍 = 푃(푋|푍) • Equivalent to 푝 푋, 푌 푍 = 푝 푋 푍 푃(푌|푍) 23 Naïve Bayes • Naïve Bayes assumption – Features are independent given class label 푝(푥1, 푥2|푦 ) = 푝(푥1|푦) 푝(푥2|푦) – More generally 푚 푝 푥1, … , 푥푚|푦 = ෑ 푝(푥|푦) =1 • How many parameters now? – Suppose 푥 is composed of 푚 binary features 24 The Naïve Bayes Classifier • Given 푌 – Prior 푝(푦) – 푚 conditionally independent features 푋 given the class 푌 푋1 푋2 푋푛 – For each 푋, we have likelihood 푃(푋|푌) • Classify via ∗ 푦 = ℎ푁퐵 푥 = arg max 푝 푦 푝(푥1, … , 푥푚|푦) 푦 푚 = arg max 푝(푦) ෑ 푝(푥|푦) 푦 25 MLE for the Parameters of NB • Given dataset, count occurrences for all pairs – 퐶표푢푛푡(푋 = 푥, 푌 = 푦) is the number of samples in which 푋 = 푥 and 푌 = 푦 • MLE for discrete NB 퐶표푢푛푡 푌 = 푦 푝 푌 = 푦 = ′ σ푦′ 퐶표푢푛푡(푌 = 푦 ) 퐶표푢푛푡 푋 = 푥, 푌 = 푦 푝 푋 = 푥 푌 = 푦 = ′ σ ′ 퐶표푢푛푡 (푋 = 푥 , 푌 = 푦) 푥푖 26 Naïve Bayes Calculations 27 Subtleties of NB Classifier: #1 • Usually, features are not conditionally independent: 푚 푝 푥1, … , 푥푚 푦 ≠ ෑ 푝(푥|푦) =1 • The naïve Bayes assumption is often violated, yet it performs surprisingly well in many cases • Plausible reason: Only need the probability of the correct class to be the largest! – Example: binary classification; just need to figure out the correct side of 0.5 and not the actual probability (0.51 is the same as 0.99). 28 Subtleties of NB Classifier: #2 • What if you never see a training instance (푋1 = 푎, 푌 = 푏) – Example: you did not see the word Enlargement in spam! – Then 푝 푋1 = 푎 푌 = 푏 = 0 – Thus no matter what values 푋2, ⋯ , 푋푚take 푃 푋1 = a, 푋2 = 푥2, ⋯ , 푋푚 = 푥푚 푌 = 푏 = 0 – Why? 29 Subtleties of NB Classifier: #2 • To fix this, use a prior! – Already saw how to do this in the coin-flipping example using the Beta distribution – For NB over discrete spaces, can use the Dirichlet prior – The Dirichlet distribution is a distribution over 푧1, … , 푧푘 ∈ (0,1) such that 푧1 + ⋯ + 푧푘 = 1 characterized by 푘 parameters 훼1, … , 훼푘 푘 훼푖−1 푓 푧1, … , 푧푘; 훼1, … , 훼푘 ∝ ෑ 푧 =1 • Called smoothing, what are the MLE estimates under these kinds of priors? 30.
Details
-
File Typepdf
-
Upload Time-
-
Content LanguagesEnglish
-
Upload UserAnonymous/Not logged-in
-
File Pages30 Page
-
File Size-