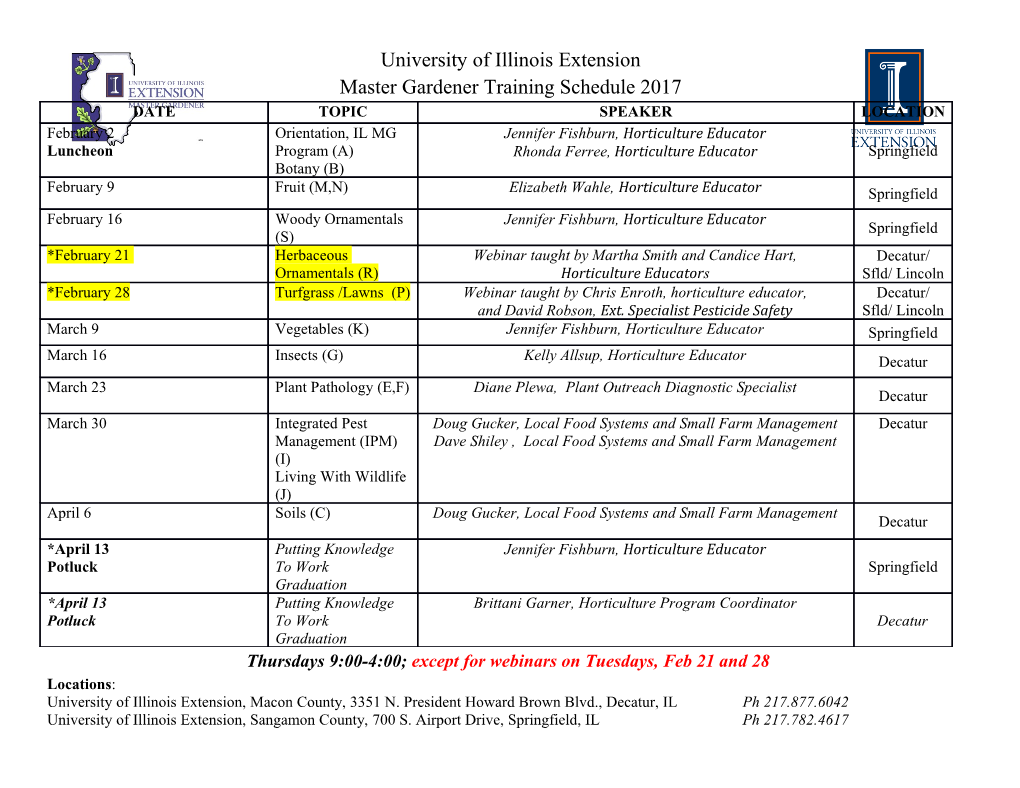
Advanced Methodology for European Laeken Indicators Deliverable 4.2 Robust Methodology for Laeken Indicators Version: 2011 Beat Hulliger, Andreas Alfons, Peter Filzmoser, Angelika Meraner, Tobias Schoch and Matthias Templ The project FP7{SSH{2007{217322 AMELI is supported by European Commission funding from the Seventh Framework Programme for Research. http://ameli.surveystatistics.net/ II Contributors to deliverable 4.2 Chapter 1: Beat Hulliger and Tobias Schoch, University of Applied Sciences Northwest- ern Switzerland. Chapter 2: Beat Hulliger and Tobias Schoch, University of Applied Sciences Northwest- ern Switzerland. Chapter 3: Andreas Alfons, Matthias Templ, Peter Filzmoser, and Josef Holzer, Vienna University of Technology. Chapter 4: Beat Hulliger and Tobias Schoch, University of Applied Sciences Northwest- ern Switzerland. Chapter 5: Beat Hulliger and Tobias Schoch, University of Applied Sciences Northwest- ern Switzerland. Chapter 6: Beat Hulliger and Tobias Schoch, University of Applied Sciences Northwest- ern Switzerland. Chapter 7: Beat Hulliger and Tobias Schoch, University of Applied Sciences Northwest- ern Switzerland. Chapter 8: Matthias Templ, Alexander Kowarik, Peter Filzmoser, Vienna University of Technology. Chapter 9: Beat Hulliger and Tobias Schoch, University of Applied Sciences Northwest- ern Switzerland. Chapter 10: Beat Hulliger and Tobias Schoch, University of Applied Sciences North- western Switzerland. Chapter 11: Matthias Templ, Karel Hron, Peter Filzmoser, Vienna University of Tech- nology. Chapter 12: Angelika Meraner, Peter Filzmoser, and Matthias Templ, Vienna Univer- sity of Technology. Main responsibility Beat Hulliger, University of Applied Sciences Northwestern Switzerland. Evaluators Internal experts: Ralf Munnich,¨ Christian Bruch, Tobias Enderle, Jan-Philipp Kolb, and Stefan Zins AMELI-WP4-D4.2 III Aim and Objectives of Deliverable 4.2 Indicators and in particular the Laeken indicators are vulnerable to outliers. Outliers may not only bias the indicators but also introduce a high additional variability. Therefore, outliers and other deviations from theoretical distributions may undermine the quality of indicators thoroughly. Robust procedures remain stable in those situations but are more complex to handle. The workpackage on robustness of the AMELI project developed robust imputation procedures, in particular for multivariate data, including detection of outlying and influential observations and small area estimation procedures. The pro- cedures were implemented in the statistical programming language R and corresponding packages were developed. The evaluation of the robustness of classical indicators, in particular the Laeken indicators, and of the new robust procedures will be described in Deliverable D7.1. Quality measures of the robustness of indicators and of the impact of robust procedures were developed in Workpackage 4 and implemented in the Workpack- ages 6 and 7. As a result of the analysis carried out on the robustness of procedures in Workpackage 4, 6, and 7 recommendations are formulated in Deliverable D7.1 for the use of indicators in what concerns robustness issues. Workpackage 4 was only possible due to an intensive and fruitful collaboration between Technical University of Vienna and Uni- versity of Applied Sciences Northwestern Switzerland. The discussions on contaminations and the subsequent implementation in the simulation environments of the AMELI project have brought research on robustness in survey sampling to a new level. The insight that was possible due to the simulations and the new methods developed would not have been possible without the support of the European Union through the Socio-economic Sciences and Humanities programme of the 7th Framework Programme for Research and Develop- ment. The workpackage contributors are greatful for the support of the European Union and for the additional support granted from their respective institutions. © http://ameli.surveystatistics.net/ - 2011 IV AMELI-WP4-D4.2 Contents 1 Introduction3 I Robust Univariate Methods7 2 Basic Robust Univariate Estimators9 2.1 Weighted Sample Median . 10 2.2 Trimmed and Winsorized Weighted Sample Mean . 10 2.3 Robust Horvitz-Thompson and Ratio M-Estimators . 12 2.4 Choosing the tuning constant . 13 3 Semi-Parametric Robust Estimation 17 3.1 Introduction................................... 17 3.2 Social exclusion indicators . 19 3.2.1 Quintile share ratio (QSR) . 19 3.2.2 Gini coefficient . 19 3.3 The Pareto distribution . 20 3.4 Findingthethreshold.............................. 21 3.4.1 Van Kerm's rule of thumb . 22 3.4.2 Pareto quantile plot . 23 3.4.3 Mean excess plot . 24 3.5 Estimation of the shape parameter . 26 3.5.1 Hill estimator . 26 3.5.2 Weighted maximum likelihood estimator . 27 3.5.3 Integrated squared error estimator . 28 3.5.4 Partial density component estimator . 29 3.6 Estimation of the indicators using Pareto tail modeling . 30 3.7 Conclusions . 32 © http://ameli.surveystatistics.net/ - 2011 VI Contents 4 Robust Non-Parametric Quintile Share Ratio Estimator 35 4.1 Introduction................................... 35 4.2 Preliminaries . 36 4.3 Robustness properties and data contamination . 39 4.3.1 Robust income quantile share ratio estimators . 42 4.4 Estimation with Complex Survey Data . 44 4.4.1 SamplingDesign ............................ 44 4.4.2 Asymptotic framework . 46 4.4.3 Finite population estimates . 48 4.5 Variance estimation . 48 4.6 Adaptive estimation . 53 4.7 Proofs ...................................... 54 5 Robust Basic Unit-Level Small-Area-Estimation Model 59 5.1 Introduction................................... 59 5.2 Small Area Estimation . 60 5.2.1 Unit-Level Models . 60 5.3 Bounded Influence-Equation Approach . 61 5.4 Proposed Method . 63 5.4.1 Preparations . 63 5.4.2 Updating Equations . 66 5.4.3 Algorithm................................ 68 5.4.4 Initialization and choice of starting values . 68 5.4.5 Prediction . 69 5.5 Conclusion . 70 II Robust Multivariate Methods for Incomplete Income Data 73 6 Robust Multivariate Methods: An Overview 75 6.1 Aggregation of the Income Components . 76 AMELI-WP4-D4.2 Contents VII 7 Multivariate Outliers 79 7.1 Introduction................................... 79 7.2 Outlier-, contamination- and missingness-mechanisms . 79 7.2.1 Notation . 80 7.2.2 Mechanisms . 81 7.2.3 Inference . 84 8 EM-based Regression Imputation Using Robust Methods 89 8.1 Introduction................................... 89 8.1.1 Imputation methods . 91 8.1.2 Software for imputation . 91 8.2 The algorithm IVEWARE ............................. 92 8.3 The algorithm IRMI ............................... 93 8.3.1 Properties . 94 8.4 Comparison using exploratory examples including outliers . 96 8.5 Simulationstudies ............................... 99 8.5.1 Error measures . 99 8.5.2 First configuration: varying the correlation structure . 100 8.5.3 Second configuration: varying the number of variables . 101 8.5.4 Third/fourth configuration: varying the amount of outliers using variables with high/low correlation . 103 8.6 Application to EU-SILC . 103 8.7 Conclusions . 106 9 Robust Methods for Elliptically Contoured Data 111 9.1 Data Preparation . 113 9.2 Outlier Detection . 113 9.2.1 BACON-EEM . 113 9.2.2 GIMCD: Gaussian Imputation followed by MCD-detection . 114 9.2.3 TRC: Tranformed Rank Correlations . 114 9.3 Imputation ................................... 115 © http://ameli.surveystatistics.net/ - 2011 Contents 1 10 Robust Methods for Non-Elliptically Contoured Data 117 10.1 Detection by the Epidemic Algorithm . 118 10.2 Imputation by Reverse Epidemic Algorithm . 119 11 Robust Imputation for Compositional Data 121 11.1Introduction................................... 121 11.1.1 Imputation ............................... 121 11.1.2 Compositional Data . 122 11.2 One-to-One Transformations for Compositional Data and Their Properties Related to Imputation . 122 11.3 Challenges . 123 11.3.1 Outliers ................................. 123 11.3.2 The Structure of Missing Values . 124 11.3.3 Zeros................................... 125 11.3.4 Measuring the Uncertainty of the Imputations . 125 11.4 Imputation Algorithms for Compositional Data . 126 11.4.1 k-Nearest Neighbor Imputation . 126 11.4.2 alr-EM algorithm . 126 11.4.3 Iterative Robust Model-Based Imputation . 126 11.4.4 Other Imputation Methods for Compositional Data . 127 11.5Results...................................... 128 11.5.1 Data Used . 128 11.6 Conclusions . 130 12 Robust Methods for Semi-Continuous Data 135 12.1 Adaption of Robust Methods for Semi-continuous Variables . 135 12.1.1 The OGK Estimator for Semi-continuous Variables . 136 12.1.2 The sign1 Covariance Matrix for Semi-continuous Variables . 142 12.1.3 Quadrant Correlation and Covariance for Semi-continuous Variables 147 12.1.4 Outlier Detection for Semi-continuous Variables . 149 12.2 Simulations and Results . 150 12.2.1 Data Generation . 150 12.2.2 Simulation................................ 152 12.3 Summary and Conclusions . 164 AMELI-WP4-D4.2 2 Contents © http://ameli.surveystatistics.net/ - 2011 Chapter 1 Introduction The workpackage on robustness could profit from the development work in the EUREDIT (cf. EUREDIT, 2003) project of the 5th Framework for Research Technology Develop- ment (RTD) of the European Union. The work of EUREDIT resulted in two important papers on multivariate outlier detection and imputation: Beguin´ and Hulliger (2004) and Beguin´ and Hulliger (2008). In that project, research concentrated more on the issues
Details
-
File Typepdf
-
Upload Time-
-
Content LanguagesEnglish
-
Upload UserAnonymous/Not logged-in
-
File Pages173 Page
-
File Size-