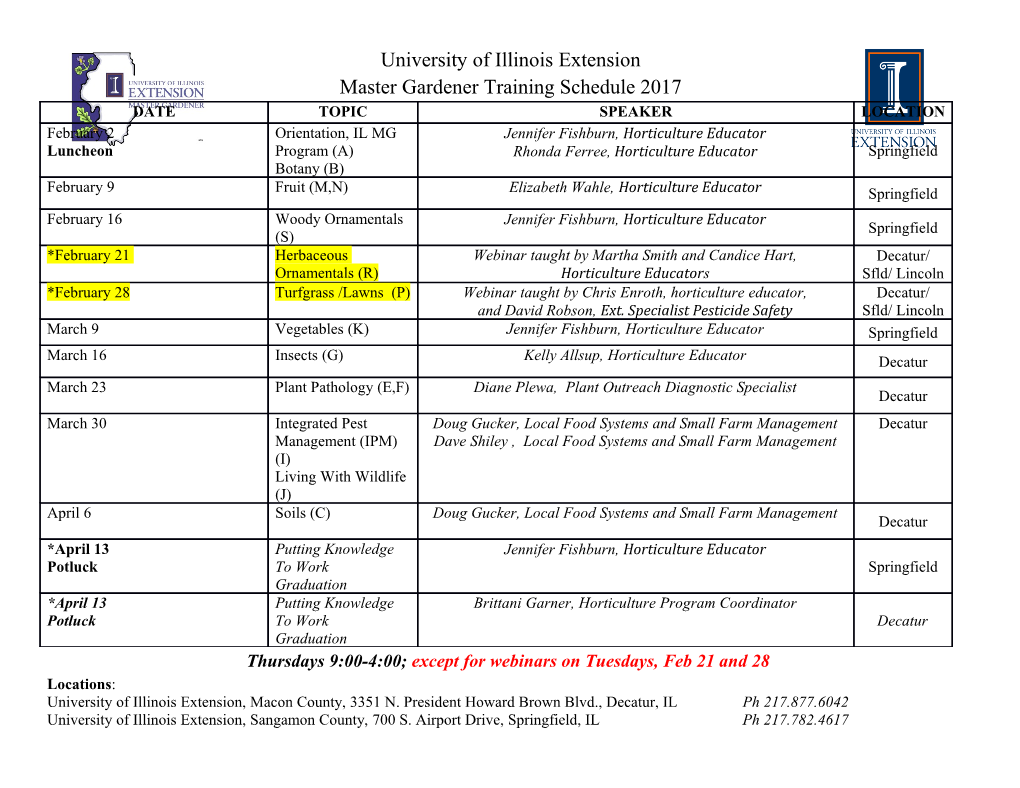
A reprint from American Scientist the magazine of Sigma Xi, The Scientific Research Society This reprint is provided for personal and noncommercial use. For any other use, please send a request Brian Hayes by electronic mail to [email protected]. Computing Science Computer Vision and Computer Hallucinations A peek inside an artificial neural network reveals some pretty freaky images. Brian Hayes eople have an amazing knack images specially designed to fool the network proposes a label. If the choice for image recognition. We networks, much as optical illusions fool is incorrect, an error signal propagates can riffle through a stack of the biological eye and brain. Another backward through the layers, reducing pictures and almost instantly approach runs the neural network in the activation of the wrongly chosen Plabel each one: dog, birthday cake, bi- reverse; instead of giving it an image output neuron. The training process cycle, teapot. What we can’t do is ex- as input and asking for a concept as does not alter the wiring diagram of plain how we perform this feat. When output, we specify a concept and the the network or the internal operations you see a rose, certain neurons in your network generates a corresponding of the individual neurons. Instead, it brain’s visual cortex light up with ac- image. A related technique called deep adjusts the weight, or strength, of the tivity; a tulip stimulates a different set dreaming burst on the scene last spring connections between one neuron and of cells. What distinguishing features following a blog post from Google Re- the next. The discovery of an efficient of the two flowers determine this re- search. Deep dreaming transforms and “backpropagation” algorithm, which sponse? Experiments that might an- embellishes an image with motifs the quickly identifies the weights that most swer such questions are hard to carry network has learned to recognize. A need adjusting, was the key to making out in the living brain. mountaintop becomes a bird’s beak, a neural networks a practical tool. What about studying image recog- button morphs into an eye, landscapes Early neural networks had just one nition in an artificial brain? Comput- teem with turtle-dogs, fish-lizards, and hidden layer, because deeper networks ers have lately become quite good at other chimeric creatures. These fanciful, were too difficult to train. In the past classifying images—so good that ex- grotesque images have become an In- 10 years this problem has been over- pert human classifiers have to work ternet sensation, but they can also serve come by a combination of algorithmic hard to match their performance. as a mirror on the computational mind, innovation, faster hardware, and larger Because these computer systems are however weirdly distorted. training sets. Networks with more than products of human design, it seems a dozen layers are now commonplace. we should be able to say exactly how Learning to See Some networks are fully connected: they work. But no: It turns out com- The neurons of an artificial neural Every neuron in a layer receives input putational vision systems are almost network are simple signal-processing from every neuron in the layer below. as inscrutable as biological ones. They units. Thousands or millions of them The new image-recognition networks are “deep neural networks,” mod- are arranged in layers, with signals are built on a different plan. In most of eled on structures in the brain, and flowing from one layer to the next. the layers each neuron receives inputs their expertise is not preprogrammed A neural network for classifying from only a small region of the layer but rather learned from examples. images has an input layer at the bot- below—perhaps a 3×3 or 5×5 square. What they “know” about images is tom with one neuron for each pixel (or All of these patches share the same stored in huge tables of numeric co- three neurons per pixel for color im- set of weights, and so they detect the efficients, which defy direct human ages.) At the top of the stack is a layer same motifs, regardless of position in comprehension. with one output neuron for each pos- the image plane. The result of apply- In the past year or two, however, sible category of image. Between the ing such position-independent filters neural nets have begun to yield up a input and output layers are “hidden” is known as convolution, and image- few fleeting glimpses of what’s going layers, where features that distinguish processing systems built in this way on inside. One set of clues comes from one class from another are somehow are called convolutional neural networks, extracted and stored. or convnets. Brian Hayes is senior writer for American Scien- A newly constructed neural network The convnet architecture creates a tist. Additional material related to the Comput- is a blank slate; before it can recognize natural hierarchy of image structures. ing Science column can be found online at http:// anything, it must be trained. An image In the lower layers of the network each bit-player.org. E-mail: [email protected] is presented to the input layer, and the neuron sees a neighborhood of only a 380 American Scientist, Volume 103 © 2015 Brian Hayes. Reproduction with permission only. Contact [email protected]. The process known as deep dreaming transforms a photograph of pe- has learned to “look for” in images. Many of the embellishments seem culiar landforms—conical sandstone “hoodoos” in northern New to arise from local features of the image. A dark patch becomes a dog’s Mexico—into a far stranger collage of animal forms, faces, architectural eye or nose, and the rest of the animal grows from that nucleus. But fantasies and abstract patterns. The algorithm probes the content of an there are also intriguing global transformations. Note how parts of the artificial neural network, accentuating various motifs that the network steep terrain have become a gently sloping plane seen in perspective. few pixels, but as information propa- leagues. The network is a 22-layer ers of the network, they ask what in- gates upward it diffuses over wider convnet with some 60 million param- put image would maximize the target areas. Thus small-scale features (eyes, eters to be adjusted during training. neuron’s level of activation. A varia- nose, mouth) later become elements of tion of the backpropagation algorithm a coherent whole (a face). Seeing in Reverse can answer this question, producing An annual contest called the Image- When a convnet learns to recognize a an image that in some sense embodies Net Large Scale Visual Recognition Welsh springer spaniel, what exactly the network’s vision of a flower or an Challenge has become a benchmark has it learned? If a person performs the automobile. (You might try the same for progress in computer vision. Con- same task, we say that he or she has exercise for yourself. When you sum- testants are given a training set of 1.2 acquired a concept, or mental model, of mon to mind a category such as mea- million images sorted into 1,000 cat- what the dog breed looks like. Perhaps suring cup, what images flash before egories. Then the trained programs the same kind of model is encoded in your eyes?) must classify another 100,000 images, the connection weights of GoogLeNet, The reversal process can never be trying to match the labels suggested by but where should you look for it among complete and unambiguous. Classi- human viewers. Some of the categories those 60 million parameters? fication is a many-to-one mapping, are fairly broad (restaurant, barn), oth- One promising trick for sifting which means the inverse mapping is ers much more specific (Welsh spring- through the network’s knowledge is to one-to-many. Each class concept repre- er spaniel, steel arch bridge). reverse the layer-to-layer flow of infor- sents a potentially infinite collection of For the past three years the con- mation. Among the groups exploring input images. Moreover, the network test has been dominated by convnets. this idea are Andrea Vedaldi and An- does not retain all of the pixels for any The 2014 winner was a system called drew Zisserman of the University of of these images, and so it cannot show GoogLeNet, developed by Christian Oxford and their colleagues. Given a us representative examples. As mem- Szegedy of Google and eight col- specific target neuron in the upper lay- bers of the Oxford group write, “the www.americanscientist.org © 2015 Brian Hayes. Reproduction with permission only. 2015 November–December 381 Contact [email protected]. network captures just a sketch of the ed interest not just from the computer objects.” All we can hope to recover vision community but also from art- is a murky and incomplete collage of ists, cognitive scientists, and the press features that the convnet found to be and public. This new genre of graphic useful in classification. The dalmatian works was given the name inceptionism, image has black and white spots, and alluding to a line in the science fiction the lemon image includes globular yel- film Inception: “We need to go deeper.” low objects, but many other details are A follow-up blog post introduced the missing or indecipherable. term deep dream, which has caught on. The algorithm behind the deep Learning from Failure dream images was devised by Alex- Quite a lot of what’s known about hu- ander Mordvintsev, a Google soft- man cognitive abilities comes from ware engineer in Zurich. In the blog studies of mental malfunctions, in- posts he was joined by two coauthors: cluding the effects of injury and dis- Mike Tyka, a biochemist, artist, and ease as well as more mundane events Google software engineer in Seattle; such as verbal errors and misinter- and Christopher Olah of Toronto, a preted images.
Details
-
File Typepdf
-
Upload Time-
-
Content LanguagesEnglish
-
Upload UserAnonymous/Not logged-in
-
File Pages5 Page
-
File Size-