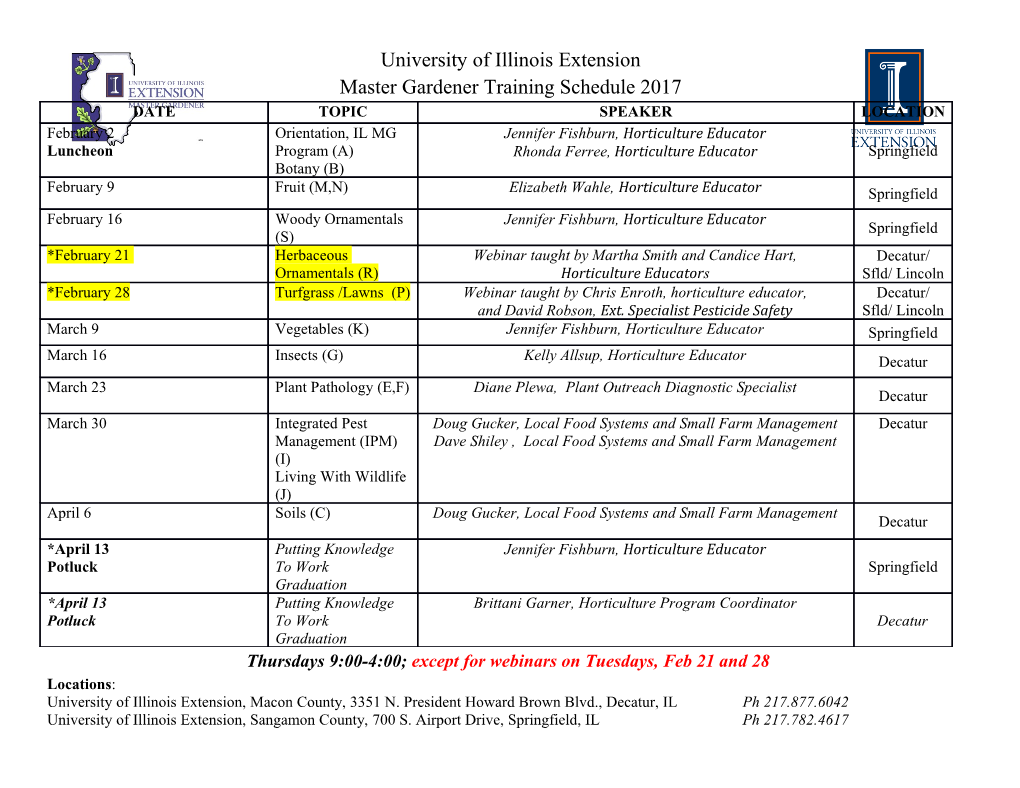
TOPICS IN HIGH-DIMENSIONAL APPROXIMATION THEORY DISSERTATION Presented in Partial Fulfillment of the Requirements for the Degree Doctor of Philosophy in the Graduate School of the Ohio State University By Yeonjong Shin Graduate Program in Mathematics The Ohio State University 2018 Dissertation Committee: Dongbin Xiu, Advisor Ching-Shan Chou Chuan Xue c Copyright by Yeonjong Shin 2018 ABSTRACT Several topics in high-dimensional approximation theory are discussed. The fundamental problem in approximation theory is to approximate an unknown function, called the target function by using its data/samples/observations. Depending on different scenarios on the data collection, different approximation techniques need to be sought in order to utilize the data properly. This dissertation is concerned with four different approximation methods in four different scenarios in data. First, suppose the data collecting procedure is resource intensive, which may require expensive numerical simulations or experiments. As the performance of the approximation highly depends on the data set, one needs to carefully decide where to collect data. We thus developed a method of designing a quasi-optimal point set which guides us where to collect the data, before the actual data collection procedure. We showed that the resulting quasi-optimal set notably outperforms than other standard choices. Second, it is not always the case that we obtain the exact data. Suppose the data is corrupted by unexpected external errors. These unexpected corruption errors can have big magnitude and in most cases are non-random. We proved that a well-known classical method, the least absolute deviation (LAD), could effectively eliminate the corruption er- rors. This is a first systematic and mathematical analysis of the robustness of LAD toward the corruption. Third, suppose the number of data is insufficient. This leads an underdetermined system which admits infinitely many solutions. The common approach is to seek a sparse solution via the `1-norm due to the work of [22, 24]. A key issue is to promote the sparsity using as few data as possible. We consider an alternative approach by employing `1−`2, motivated by its contours. We improve the existing theoretical recovery results of `1−`2, extended it ii to the function approximation. Lastly, suppose the data is sequentially or continuously collected. Due to its large volume, it could be very difficult to store and process all data, which is a common challenge in big data. Thus a sequential function approximation method is presented. It is an iterative method which requires ONLY vector operations (no matrices). It updates the current solution using only one sample at a time, and does not require storage of the data set. Thus the method can handle sequentially collected data and gradually improve its accuracy. We establish sharp upper and lower bounds and also establish the optimal sampling probability measure. We remark that this is the first work on this new direction. iii ACKNOWLEDGMENTS With the completion of this dissertation, one big chapter of my life as a student finally has come to an end. At the moment of writing my dissertation acknowledgments, I find myself somewhat speechless and unsure of where to begin. It has been a long adventurous journey and it would not be possible without the help of many individuals. First and foremost, I express my deepest gratitude to my Ph.D. thesis advisor, Professor Dongbin Xiu. During my graduate years, he has been a kind and thoughtful mentor and an enthusiastic and insightful advisor. His scientific insight is exceptional and his ability to share it is second to none. I am truly grateful for the support and opportunities he has provided. He has not only taught me many invaluable academic lessons, but many important life lessons that I will treasure for a lifetime. It was a great pleasure to be his student and to work with him. Secondly, I extend my gratitude to three of my undergraduate professors. Professor Hyoung June Ko is at the Department of Mathematics, Yonsei University in South Korea. He is the one who taught me how to study and understand mathematics and the underlying common concept flowing through all fields of mathematics. Also he has taught what the true treasures in my life are. Professor Jeong-Hoon Kim is also at the Department of Mathematics, Yonsei University. He is the one who helped me a lot in preparing and applying the graduate schools. Without his help, I might not have an opportunity to study abroad. Professor Yoon Mo Jung was at Yonsei University, now at the Department of Mathematics, Sungkyunkwan University in South Korea. He was my undergraduate research advisor. It was him who guided me to the field of computational mathematics and scientific computing. He has been provided so many realistic advice and valuable comments and shared his experience of a path I have yet to tread. Also he is the one who introduces iv Professor Dongbin Xiu to me at Yonsei University, back in 2013. Thirdly, I express my appreciation to my dissertation committee members, Professor Ching-Shan Chou and Professor Chuan Xue, for sparing their precious time. Also I thank to Dr. Jeongjin Lee who recognized my mathematical talent and encouraged me to study more advanced mathematics in my teenage years. Last but not the least, my utmost thanks and immense gratitude go to my family. My parents, Kangseok Shin and Junghee Hwang in South Korea, and my older brother Yeonsang Shin who is an architect in Tokyo, Japan, have always been there for me and believed in me in every step of my life. My parents-in-law, Jangwon Lee and Mihyung Yoo in South Korea, and my brother-in-law Daesop Lee in California, have helped me in various ways in my life in the United States. Altogether my entire family have supported me in all those years and made my life a much better and a much more comfortable one. Without their support and love, this would probably never have been written. Above all I would like to thank my beloved wife, a great pianist, Yunjin Lee, who is a doctoral student at the University of Texas at Austin. Ever since we were undergraduates together at Yonsei University, she has been supportive, encouraging, and taking care of me. From writing thoughtful cards, to listening to my thoughts, fears, and excitement, to doing everything in her power to make sure I had the strength I needed to face the many challenges along the way, she has always been there doing anything she could to lighten the load, and make me smile along the way. v VITA 1988 . Born in Seoul, South Korea 2013 . Bachelor of Science in Mathematics, Yonsei University 2013 . Bachelor of Arts in Economics, Yonsei University Present . Graduate Research Associate, The Ohio State University PUBLICATIONS [7] Y. Shin, K. Wu and D. Xiu, Sequential function approximation using randomized samples, J. Comput. Phys., 2017 (submitted for publication). [6] K. Wu, Y. Shin and D. Xiu, A randomized tensor quadrature method for high dimensional polynomial approximation, SIAM J. Sci. Comput., 39(5), A1811-A1833 (2017). [5] Y. Shin and D. Xiu, A randomized algorithm for multivariate function approximation, SIAM J. Sci. Comput., 39(3), A983-A1002 (2017). [4] L. Yan, Y. Shin and D. Xiu, Sparse approximation using `1-`2 minimization and its applications to stochastic collocation, SIAM J. Sci. Comput., 39(1), A229-A254 (2017). [3] Y. Shin and D. Xiu, Correcting data corruption errors for multivariate function approximation, SIAM J. Sci. Comput., 38(4), A2492-A2511 (2016). vi [2] Y. Shin and D. Xiu, On a near optimal sampling strategy for least squares polynomial regression, J. Comput. Phys., 326, 931-946 (2016). [1] Y. Shin and D. Xiu, Nonadaptive quasi-optimal points selection for least squares linear regression, SIAM J. Sci. Comput. 38(1), A385-A411 (2016). FIELDS OF STUDY Major Field: Mathematics Specialization: Approximation theory vii TABLE OF CONTENTS Page Abstract . ii Acknowledgments . iv Vita............................................. vi List of Figures . xii List of Tables . xvi Chapters 1 Introduction ..................................... 1 1.1 Expensive data and Least squares . 3 1.2 Few data and Advanced sparse approximation . 6 1.3 Big data and Sequential approximation . 7 1.4 Corrupted data and Least absolute deviation . 10 1.5 Application: Uncertainty Quantification . 11 1.6 Objective and Outline . 13 2 Review: Approximation, regression and orthogonal polynomials .... 14 2.1 Function approximation . 14 2.2 Overdetermined linear system . 17 2.3 Underdetermined linear system . 19 2.3.1 Sparsity . 20 2.4 Orthogonal Polynomials . 21 viii 2.5 Tensor Quadrature . 24 2.6 Christoffel function and the pluripotential equilibrium . 26 2.6.1 Pluripotential equilibrium measure on Bounded domains . 27 2.6.2 Pluripotential equilibrium measure on Unbounded domains . 27 2.7 Uncertainty Quantification . 30 2.7.1 Stochastic Galerkin method . 31 2.7.2 Stochastic collocation . 32 3 Optimal Sampling .................................. 34 3.1 Quasi-optimal subset selection . 35 3.1.1 S-optimality . 39 3.2 Greedy algorithm and implementation . 41 3.2.1 Fast greedy algorithm without determinants . 43 3.3 Polynomial least squares via quasi-optimal subset . 44 3.4 Orthogonal polynomial least squares via quasi-optimal subset . 45 3.4.1 Asymptotic distribution of quasi-optimal points . 46 3.5 Near optimal subset: quasi-optimal subset for Christoffel least squares . 51 3.5.1 Asymptotic distribution of near optimal points . 54 3.6 Summary . 57 3.6.1 Quasi optimal sampling for ordinary least squares . 57 3.6.2 Near optimal sampling for Christoffel least squares . 58 4 Advanced Sparse Approximation ........................ 59 4.1 Review on `1-`2 minimization . 59 4.2 Recovery properties of `1-`2 minimization . 61 4.3 Function approximation by Legendre polynomials via `1-`2 .
Details
-
File Typepdf
-
Upload Time-
-
Content LanguagesEnglish
-
Upload UserAnonymous/Not logged-in
-
File Pages217 Page
-
File Size-