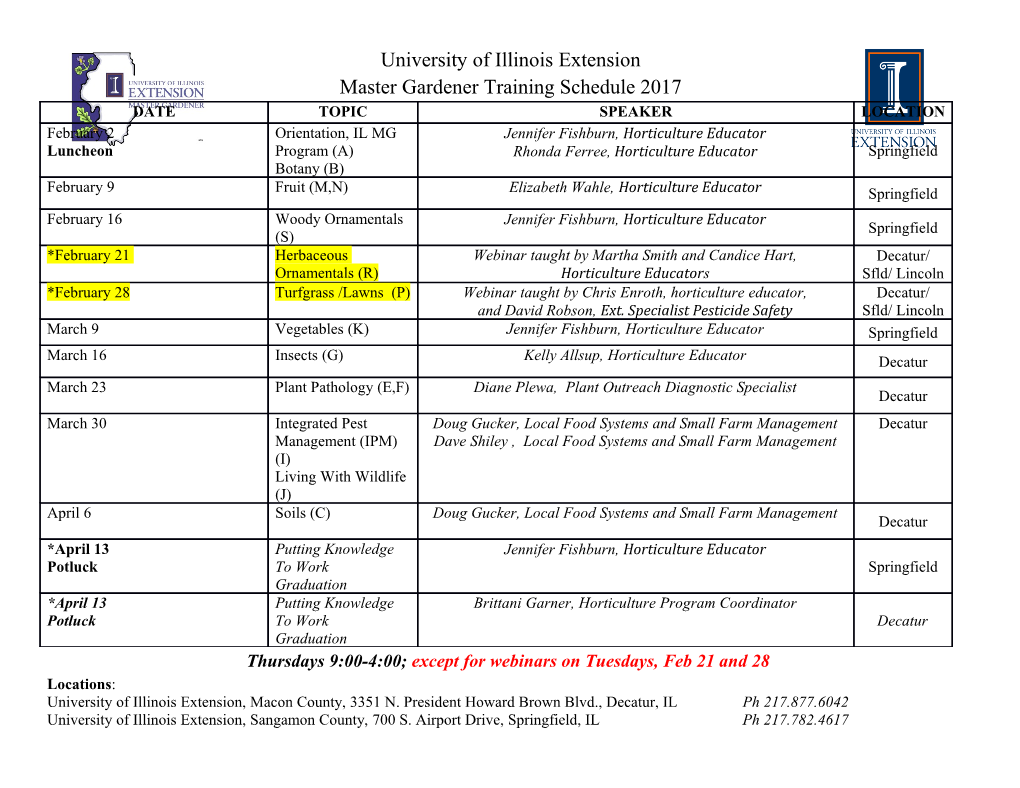
NBER WORKING PAPER SERIES GROUP-AVERAGE OBSERVABLES AS CONTROLS FOR SORTING ON UNOBSERVABLES WHEN ESTIMATING GROUP TREATMENT EFFECTS: THE CASE OF SCHOOL AND NEIGHBORHOOD EFFECTS Joseph G. Altonji Richard K. Mansfield Working Paper 20781 http://www.nber.org/papers/w20781 NATIONAL BUREAU OF ECONOMIC RESEARCH 1050 Massachusetts Avenue Cambridge, MA 02138 December 2014 We thank Steven Berry, Greg Duncan, Phil Haile, Hidehiko Ichimura, Amanda Kowalski, Costas Meghir, Richard Murnane, Douglas Staiger, Jonathan Skinner, Shintaro Yamiguchi and as well as seminar participants at Cornell University, Dartmouth College, Duke University, the Federal Reserve Bank of Cleveland, McMaster University, the NBER Economics of Education Conference, Yale University, Paris School of Economics, University of Colorado-Denver, and the University of Western Ontario for helpful comments and discussions. This research uses data from the National Center for Education Statistics as well as from North Carolina Education Research Data Center at Duke University. We acknowledge both the U.S. Department of Education and the North Carolina Department of Public Instruction for collecting and providing this information. We would also thank the Russell Sage Foundation and the Yale Economics Growth Center for financial support. A portion of this research was conducted while Altonji was a visitor at the LEAP Center and the Department of Economics, Harvard University The views expressed herein are those of the authors and do not necessarily reflect the views of the National Bureau of Economic Research. NBER working papers are circulated for discussion and comment purposes. They have not been peer- reviewed or been subject to the review by the NBER Board of Directors that accompanies official NBER publications. © 2014 by Joseph G. Altonji and Richard K. Mansfield. All rights reserved. Short sections of text, not to exceed two paragraphs, may be quoted without explicit permission provided that full credit, including © notice, is given to the source. Group-Average Observables as Controls for Sorting on Unobservables When Estimating Group Treatment Effects: the Case of School and Neighborhood Effects Joseph G. Altonji and Richard K. Mansfield NBER Working Paper No. 20781 December 2014 JEL No. C20,I20,I24,I26,R20 ABSTRACT We consider the classic problem of estimating group treatment effects when individuals sort based on observed and unobserved characteristics that affect the outcome. Using a standard choice model, we show that controlling for group averages of observed individual characteristics potentially absorbs all the across-group variation in unobservable individual characteristics. We use this insight to bound the treatment effect variance of school systems and associated neighborhoods for various outcomes. Across four datasets, our most conservative estimates indicate that a 90th versus 10th percentile school system increases the high school graduation probability by between 0.047 and 0.085 and increases the college enrollment probability by between 0.11 and 0.13. We also find large effects on adult earnings. We discuss a number of other applications of our methodology, including measurement of teacher value-added. Joseph G. Altonji Department of Economics Yale University Box 208264 New Haven, CT 06520-8264 and NBER [email protected] Richard K. Mansfield 266 Ives Hall School of Industrial and Labor Relations Cornell University Ithaca, NY 14853 [email protected] 1 Introduction Society is replete with contexts in which (1) a person’s outcome depends on both individual and group-level inputs, and (2) the group is endogenously chosen either by the individuals themselves or by administrators, partly based on the individual’s own inputs. Examples include health outcomes and hospitals, earnings and workplace characteristics, and test scores and teacher value-added.1 Generations of social scientists have been interested in determining whether group outcomes differ because the groups influence individual outcomes or because the groups have succeeded or failed in attracting the individuals who would have thrived regardless of the group chosen. In some cases, sources of exogenous variation are available that may be used to assess the consequences of a partic- ular group treatment. However, assessment of the overall distribution of group treatments is much more difficult, and researchers and governments frequently rely on non-experimental estimators of group treatment effects (e.g. school report cards and teacher value-added). In this paper we show that in certain circumstances the tactic of controlling for group averages of observed individual-level characteristics, generally thought to control for “sorting on observables” only, will absorb all of the between-group variation in both observable and unobservable individual inputs. We then show how this insight can be used to estimate a lower bound on the variance in the contributions of group-level treatments to individual outcomes. We also examine the conditions under which causal effects of particular observed group characteristics can be estimated. We apply our methodological insight and demonstrate its empirical value by addressing a classic question in social science: How much does the school and surrounding community that we choose for our children matter for their long run educational and labor market outcomes?2 To illustrate the sorting problem consider the following simplified production function relating education outcomes to individuals’ characteristics and the inputs of the schools/neighborhoods they choose. Let Ysi denote the outcome (e.g. attendance at a four-year college) of student i who attends and lives near 3 4 school s. Ysi is determined according to U U U U Ys;i = [Xib + Xi b ] + [ZsG + Zs G ] . (1) U Let the vectors Xi and Xi be the complete set of child and family characteristics that have a causal U impact on student i’s educational attainment. Xi is observed by the econometrician and Xi is 1Ash et al. (2012) provide an overview of the issues involved in assessing hospitals. Doyle Jr et al. (2012) also discuss the issues and provide a short literature survey. They are among a small set of studies that use a quasi-experimental design to assess effects of particular hospital characteristics on outcomes. See Chetty et al. (2014) and Rothstein (2014) for discussions and references related to the estimation of teacher value-added. 2See Duncan and Murnane (2011) for recent papers on school and neighborhood effects, with references to the lit- erature. Meghir and Rivkin (2010) discuss alternative approaches to estimating school fixed effects and the effects of particular school inputs, and highlight the problem of endogenous selection of schools and neighborhoods, among other econometric issues. 3Despite the growing popularity of open enrollment systems, most school choice is still mediated through choice of community in which to live, and most students still choose schools close to home even when given the opportunity. Thus, we aim instead to measure the importance of the combined school/neighborhood choice. 4Later we will introduce additional components to the outcome model. 1 U unobserved. Analogously, the row vectors Zs (observed) and Zs (unobserved) capture the com- plete set of school and neighborhood level influences common to students live in s, so that the U U school/neighborhood treatment effect is given by [ZsG + Zs G ]. U U Unfortunately, sorting will lead the school average of Xi , denoted Xs , to vary across s. This will U U contaminate estimates of G and fixed effect estimates of the school treatment effect ZsG + Zs G . While various studies have included controls for group-level averages of individual observables (denoted Xs), the role played by such controls in mitigating sorting bias has generally been under- appreciated. Our key insight follows directly from the parent’s school/neighborhood choice decision—average values of student characteristics differ across schools only because students/families with different characteristics value school or neighborhood amenities differently. This means that school-averages of individual characteristics such as parental education, family income, and athletic ability will be functions of the vector of amenity factors (denoted As) that parents consider when making their U school choices. Thus, the school averages Xs and Xs will be different vector-valued functions of U U U the same common set of amenities: Xs = f (As) and Xs = f (As). The functions f and f , are determined by the sorting equilibrium and reflect the equilibrium prices of the amenities. If the dimension of the underlying amenity space is smaller than the number of observed characteristics, then under certain conditions one can invert this vector-valued function to express the amenities in −1 terms of school-averages of observed characteristics: As = f (Xs). But this implies that the vector of school averages of unobserved characteristics can also be written as a function of observed char- U U −1 U acteristics: Xs = f ( f (Xs)). This function of Xs can serve as a control function for Xs when estimating group effects. We formalize this intuition by introducing a multidimensional spatial equilibrium model of school choice and providing conditions under which this mapping is exact. Given an additively U separable specification of utility, we show that the average unobserved student variables Xs are a U linear function of Xs. As we make precise in Proposition 1 below, X and X need
Details
-
File Typepdf
-
Upload Time-
-
Content LanguagesEnglish
-
Upload UserAnonymous/Not logged-in
-
File Pages73 Page
-
File Size-