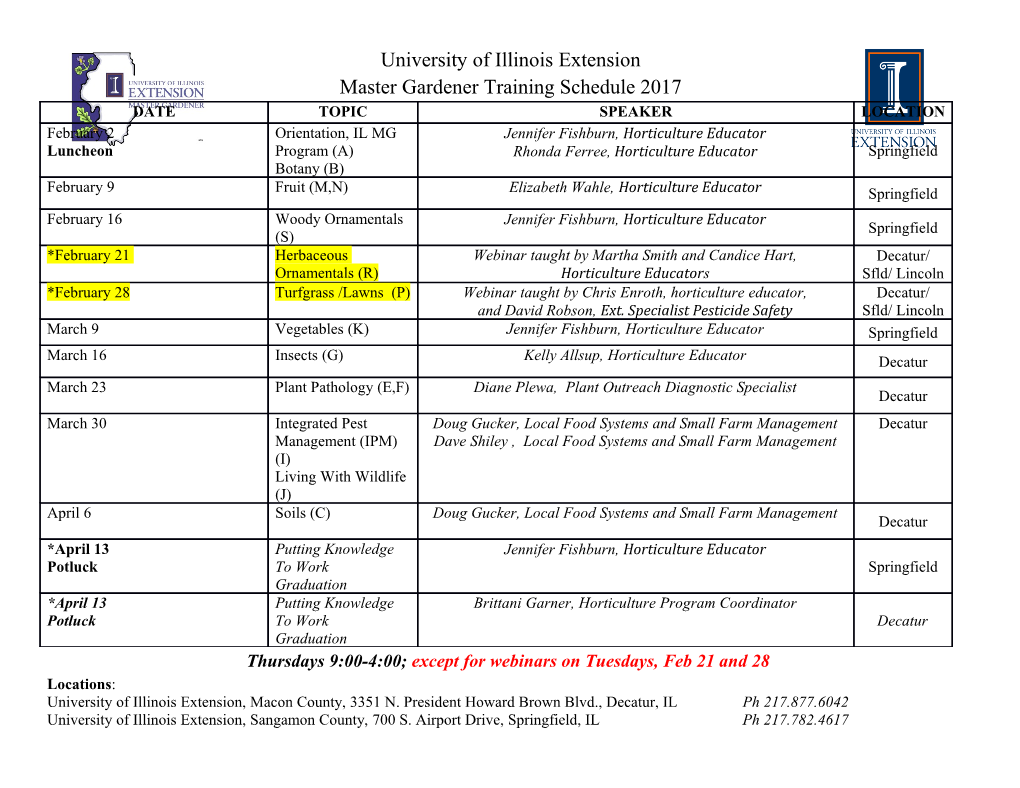
1 A High Performance Block Eigensolver for Nuclear Configuration Interaction Calculations Hasan Metin Aktulga, Md. Afibuzzaman, Samuel Williams, Aydın Buluc¸, Meiyue Shao, Chao Yang, Esmond G. Ng, Pieter Maris, James P. Vary Abstract—As on-node parallelism increases and the performance gap between the processor and the memory system widens, achieving high performance in large-scale scientific applications requires an architecture-aware design of algorithms and solvers. We focus on the eigenvalue problem arising in nuclear Configuration Interaction (CI) calculations, where a few extreme eigenpairs of a sparse symmetric matrix are needed. We consider a block iterative eigensolver whose main computational kernels are the multiplication of a sparse matrix with multiple vectors (SpMM), and tall-skinny matrix operations. We present techniques to significantly improve the SpMM and the transpose operation SpMMT by using the compressed sparse blocks (CSB) format. We achieve 3–4× speedup on the requisite operations over good implementations with the commonly used compressed sparse row (CSR) format. We develop a performance model that allows us to correctly estimate the performance of our SpMM kernel implementations, and we identify cache bandwidth as a potential performance bottleneck beyond DRAM. We also analyze and optimize the performance of LOBPCG kernels (inner product and linear combinations on multiple vectors) and show up to 15× speedup over using high performance BLAS libraries for these operations. The resulting high performance LOBPCG solver achieves 1.4× to 1.8× speedup over the existing Lanczos solver on a series of CI computations on high-end multicore architectures (Intel Xeons). We also analyze the performance of our techniques on an Intel Xeon Phi Knights Corner (KNC) processor. Keywords—Sparse Matrix Multiplication; Block Eigensolver; Configuration Interaction; Extended Roofline Model; Tall-Skinny Matrices F 1 INTRODUCTION of interest, a partial diagonalization of the large CI H^ 2 N×N The choice of numerical algorithms and how efficiently many-body Hamiltonian R is sufficient. More they can be implemented on high performance computer formally, we are interested in finding a small number of (HPC) systems critically affect the time-to-solution for extreme eigenpairs of a large, sparse, symmetric matrix: large-scale scientific applications. Several new numerical Hx^ i = λxi; i = 1; : : : ; m; m N: (1) techniques or adaptations of existing ones that can better leverage the massive parallelism available on modern Iterative methods such as the Lanczos and Jacobi– systems have been developed over the years. Although Davidson [2] algorithms, as well as their variants [3], these algorithms may have slower convergence rates, [4], [5], can be used for this purpose. The key ker- their high degree of parallelism may lead to better time- nels for these methods can be crudely summarized as to-solution on modern hardware [1]. In this paper, we (repeated) sparse matrix–vector multiplications (SpMV) consider the solution of the quantum many-body prob- and orthonormalization of vectors (level-1 BLAS). As lem using the configuration interaction (CI) formulation. alternatives, block versions of these algorithms have We present algorithms and techniques to significantly been developed [6], [7], [8] which improve the arithmetic speed up eigenvalue computations in CI by using a intensity of computations at the cost of a reduced con- block eigensolver and optimizing the key computational vergence rate and increased total number of matrix– kernels involved. vector operations [9]. In block methods, SpMV becomes The quantum many-body problem transcends several a sparse matrix multiple vector multiplication (SpMM) areas of physics and chemistry. The CI method enables and vector operations become level-3 BLAS operations. computing the wave functions associated with discrete Related Work: Due to its importance in scientific com- energy levels of these many-body systems with high puting and machine learning, several optimization tech- accuracy. Since only a small number of low energy states niques have been proposed for SpMV [10], [11], [12], [13], are typically needed to compute the physical observables [14], [15]. Performance of SpMV is ultimately bounded by memory bandwidth [16]. The widening gap between • H. M. Aktulga and M. Afibuzzaman are with Michigan State University, 428 S. Shaw Lane, Room 3115, East Lansing, MI 48824. H. M. Aktulga processor performance and memory bandwidth signifi- also has an affiliate appointment with the Lawrence Berkeley National cantly limits the achievable performance in several im- Laboratory. Corresponding author e-mail: [email protected]. portant applications. On the other hand, in SpMM, one • S. Williams, A. Bulu¸c, M. Shao, C. Yang, and E. G. Ng are with the Computational Research Division, Lawrence Berkeley National Laboratory, can make use of the increased data locality in the vector 1 Cyclotron Rd, MS 50F-1650 Berkeley, CA 94720. block and attain much higher FLOP rates on modern • P. Maris and J. Vary are with Iowa State University, Dept. of Physics and architectures. Gropp et al. was the first to exploit this Astronomy, Ames, IA 50011. idea by using multiple right hand sides for SpMV in a 2 computational fluid dynamics application [17]. SpMM nuclear CI code [23], [24], [25]. We demonstrate through is one of the core operations supported by the auto- numerical experiments that the resulting block eigen- tuned sequential sparse matrix library OSKI [12]. OSKI’s solver can outperform the widely used Lanczos algo- shared memory parallel successor, pOSKI, currently does rithm (based on single vector iterations) with modern not support SpMM [18]. More recently, Liu et al. [1] multicore architectures (Sect. 5.4). We also analyze the investigated strategies to improve the performance of performance of our techniques on an Intel Xeon Phi SpMM 1 using SIMD (AVX/SSE) instructions for Stoke- Knights Corner (KNC) processor to assess the feasibility sian dynamics simulation of biological macromolecules of our implementations for future architectures. on modern multicore CPUs. Rohrig-Z¨ ollner¨ et al. [19] While we focus on nuclear CI computations, the im- discuss performance optimization techniques for the pact of optimizing the performance of key kernels in block Jacobi–Davidson method to compute a few eigen- block iterative solvers is broader. For example, spectral pairs of large-scale sparse matrices, and report reduced clustering, one of the most promising clustering tech- time-to-solution using block methods over single vec- niques, uses eigenvectors associated with the smallest tor counterparts for quantum mechanics problems and eigenvalues of the Laplacian of the data similarity matrix PDEs. Finally, Anzt et al. [20] describe an SpMM imple- to cluster vertices in large symmetric graphs [26], [27]. mentation based on the SELLC matrix format, and show Due to the size of the graphs, it is desirable to exploit that performance improvements in the SpMM kernel the symmetry, and for a k-way clustering problem, k can translate into performance improvements in a block eigenvectors are needed, where typically 10 ≤ k ≤ 100, eigensolver running on GPUs. an ideal range for block eigensolvers. Block methods are Our Contributions: Our work differs from previous also used in solving large-scale sparse singular value efforts substantially, in part due to the immense size decomposition (SVD) problems [28], with most popular of the sparse matrices (with dimensions on the order methods being the subspace iteration and block Lanzcos. of several billions and total number of nonzero matrix SVDs are critical for dimensionality reduction in applica- elements on the order of tens of trillions) involved. We tions like latent semantic indexing [29]. In SVD, singular therefore exploit symmetry to reduce the overall mem- values are obtained by solving the associated symmet- ric eigenproblem that requires subsequent SpMM and ory footprint, and offer an efficient solution to perform T SpMM on a sparse matrix and its transpose (SpMMT ) SpMM computations in each iteration [30]. Thus, our with roughly the same performance [21]. This is achieved techniques can have a positive impact on the adoption through a novel thread parallel SpMM implementation, of block solvers in closely related applications. CSB/OpenMP, which is based on the Compressed Sparse 2 BACKGROUND Blocks (CSB) storage format [22] (Sect.3). We demon- 2.1 Eigenvalue Problem in CI Calculations strate the efficiency of CSB/OpenMP on a series of CI matrices where we obtain 3–4× speedup over the Computational simulations of nuclear systems face mul- commonly used compressed sparse row (CSR) format. tiple hurdles, as the underlying physics involves a To estimate the performance characteristics and better very strong interaction, three-nucleon interactions, and understand the bottlenecks of the SpMM kernel, we complicated collective motion dynamics. The eigenvalue propose an extended Roofline model to account for cache problem arises in nuclear structure calculations because bandwidth limitations (Sect.3). the nuclear wave functions Ψ are solutions of the many- In this paper, we extend our previous work (presented body Schrodinger’s¨ equation expressed as a Hamiltonian in [21]) by considering an end-to-end optimization of a matrix eigenvalue problem, HΨ = EΨ. block eigensolver. As discussed in Sect.4, performance of In the CI approach, both the wave functions Ψ and the tall-skinny matrix operations in block
Details
-
File Typepdf
-
Upload Time-
-
Content LanguagesEnglish
-
Upload UserAnonymous/Not logged-in
-
File Pages14 Page
-
File Size-