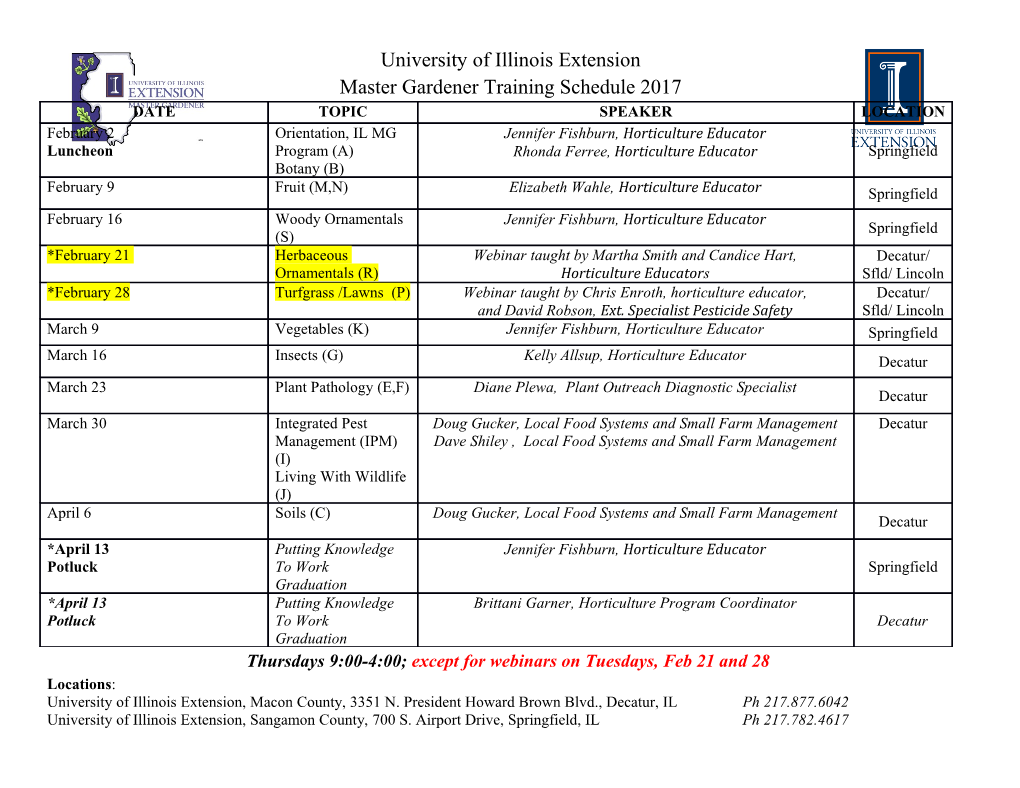
This thesis is presented for the degree of Doctor of Philosophy of The University of Western Australia Can pairs trading act like arbitrage to enforce the law of one price? Keith R L Godfrey BE(Hons) MFinMath December 2012 Financial Studies Business School The University of Western Australia Abstract This thesis confirms empirically that a pairs trading strategy can act to enforce a market price ratio between two closely-related securities, thereby behaving like arbitrage enforcing the law of one price. Any empirical study of arbitrage and pairs trading is difficult because security exchanges report trades anonymously. Trades in two securities cannot be identified as originating from the same trader, nor can the entry and exit trades of a strategy be matched. Much of this thesis is spent designing a methodology for detecting pairs trading through statistical techniques and testing its accuracy in situations where pairs trading can be anticipated. The approach works pleasingly despite being imprecise. It can isolate closely-related pairs in pseudo-random sets of similarly-traded pairs and locate fundamentally similar pairs among large sets formed by pairing index constituents. It can even detect pairs involved in merger arbitrage many months ahead of company mergers. The evidence then shows pairs trading in the twin depositary receipts of BHP Billiton (the world’s largest mining conglomerate with dual-listed company structure) act to push the prices apart when the difference falls below a recent measure, instead of reinforcing their trend towards equality. The detected pairs trades enforce the price difference: reducing it when it becomes too large and increasing it when too small. Evidence rejects a trading history of restoring price equality even though these security twins seem to offer identical dividends, risks, and entitlements. Pairs trading may be enforcing the market price ratio as a proxy for an incalculable valuation ratio underlying the law of one price. Contents Abstract 3 Contents 5 Acknowledgements 9 1 Introduction and overview 11 2 Pairs trading and the law of one price 19 2.1 Taxonomy of the literature 20 2.2 Fundamentally-related pairs 23 2.2.1 The law of one price (LOOP) 23 2.2.2 LOOP in goods and commodity markets 25 2.2.3 LOOP in capital markets 27 2.2.4 Closed-end mutual funds 32 2.2.5 Equity carve-outs and merger arbitrage 34 2.2.6 Cross listings 36 2.2.7 Dual-listed companies (DLCs) or Siamese twins 43 2.2.8 Dual-class shares 46 2.2.9 Simulations of arbitrage trading strategies 49 2.3 Quantitative or technical pairs 53 2.3.1 Pairs selection 53 2.3.2 Simulations of pairs trading strategies 59 2.3.3 Limitations of simulations 66 2.4 Studies of actual arbitrage and pairs trading 68 2.5 The research opportunity 71 3 Theory and methods for inferring the presence of pairs trading 73 3.1 Introduction 73 3.1.1 The mechanics of pairs trading 74 3.1.2 Inferring pairs trading from the trading logs 74 3.1.3 Accuracy before precision 75 3.2 Notation for the analysis of pairs trading 76 3.2.1 Sets of Cartesian products of trades 76 3.2.2 Time differences between trades 78 3.2.3 Continuous empirical measure and empirical distribution 79 3.2.4 Discrete empirical measure and empirical distribution 81 3.3 Inferring related trading 82 5 3.3.1 Related trading indicators (RTIs) by distance-to-uniform (DU) method 83 3.3.2 Visualisation using each-way CDFs 88 3.3.3 Related trading indicators (RTIs) by central-to-outer (CO) method 90 3.3.4 Two-dimensional plots for ranking security pairs by related trading 93 3.4 Methodology for inferring aggressive pairs trading 95 3.4.1 Identifying each aggressor 96 3.4.2 Partitioning trade products by relative aggression 97 3.4.3 Aggressive pairs trading indicators (APTIs) 98 3.5 Methodology for inferring passive pairs trading 98 3.5.1 Generalising with a minimum and maximum time difference T0 99 3.5.2 Passive pairs trading indicators (PPTIs) 100 3.5.3 Inferring the more frequent limit-order stock 101 3.6 Inferring the dominant pairs trading strategy 102 3.6.1 Notation for partitioning by K-quantiles 103 3.6.2 K-quantile ordering scores (KQOSs) 105 3.7 Foreseeable limitations 106 3.7.1 Weaknesses in assumptions 106 3.7.2 Differences in reporting delays between exchanges 107 3.7.3 Handling unrealistic and extreme cases 111 3.7.4 Splitting of orders 112 3.8 Summary and perspective 113 4 Experiment Design 115 4.1 Validation testing 115 4.1.1 Selection of closely-related securities 117 4.1.2 Comparison sets of pseudo-random pairs 125 4.1.3 Experimental approach in verifying the closely-related pairs 127 4.1.4 Optimisation of parameters 128 4.1.5 Aggressive and passive pairs trading indicators 129 4.1.6 Robustness to the locations of trades 129 4.2 Exploring index sets for frequently-traded security pairs 131 4.2.1 Sets of security pairs 131 4.2.2 Method of exploring large sets of pairs 134 4.2.3 Automated verification of accuracy 134 4.2.4 Re-optimisation of parameter T from index explorations 136 4.2.5 Comparison of the hypotheses and the measures 137 4.3 Searching for dominant trading strategies 137 4.3.1 Trial strategies 137 4.3.2 Comparing strategies 138 4.4 Data Source 138 4.5 Summary of experimental sequence 139 5 Empirical verification of methodology 143 5.1 Validation testing on closely related pairs 143 5.1.1 Does the related trading methodology work on closely-related pairs? 143 6 5.1.2 Can suitable T and T0 be determined by testing the closely-related pairs? 155 5.1.3 Do the aggressive and passive trading indicators work? 160 5.1.4 Is the methodology robust to the location of trade? 165 5.2 Explorations of broad index constituent pairs 174 5.2.1 Does the aggressive pairs trading methodology find index constituent pairs that can be confirmed as fundamentally related? 175 5.2.2 Is the ranking of pairs by the aggressive pairs trading methodology consistent with the algorithmic connections made by Google Finance? 192 5.2.3 Can a suitable value for T be determined from index explorations? 195 5.2.4 Do the DU and CO methods produce equivalent results? 200 5.2.5 Do the DU.CVM & DU.KS indicators and the CO.S & CO.A indicators each produce equivalent results? 203 5.3 Summary 206 6 Investigation of trading strategies 207 6.1 Inferring a dominant strategy for trades in BHP Billiton 207 6.2 Inferring a dominant strategy for trades in Carnival 210 6.3 Inferring a dominant strategy for trades in Unilever 214 6.4 Inferring a dominant strategy for trades in Royal Dutch Shell 214 6.5 Summary 215 7 Review of results and opportunities for further research 217 7.1 Exploring other strategies using the same methodology 217 7.1.1 Merger arbitrage 218 7.1.2 Break-out strategies 219 7.1.3 Broader research questions 219 7.2 Understanding the methodology better 220 7.2.1 German and Australian securities 220 7.2.2 Shape of the frontier 220 7.2.3 High-frequency or short-period artefacts 221 7.3 Other empirical studies 221 7.3.1 Inferences of trading speed 221 7.3.2 Characteristic reporting delays of security exchanges 222 7.3.3 Stock-specific reporting delays 222 7.4 Extensions to the methodology 222 7.4.1 Corrections of exchange delays 223 7.4.2 Incorporation of trading volume 223 7.4.3 Detection of related orders 224 7.4.4 Iterative ranking 224 7.4.5 Basket trading and index arbitrage 225 7.4.6 Currency and derivative markets 225 7.5 Summary 225 8 Speculating a reason for the BHP premium 227 8.1 Model for tax-induced valuation heterogeneity 227 7 8.2 Model for the BHP premium 228 8.3 Information from tax shocks 230 8.4 Summary and implications 230 9 Conclusions 231 References 233 Glossary of acronyms 243 8 Acknowledgements I am indebted to my supervisors Professors Philip Brown and Raymond da Silva Rosa for their guidance throughout this project. Raymond introduced me to the BHP Billiton pricing difference in 2008 and this thesis evolved from there. Philip is a luminary in accounting and finance research and I feel privileged he agreed to take on the supervision of my thesis in his capacity as Emeritus Professor. Overall I am fortunate to have worked with exceptional supervisors and I thank them both. I thank Professors Terry Walter, Doug Foster, Tom Smith, Graeme Partington, Arnold Zellner, and workshop participants at UWA and UTS for further discussions of my work and working papers. I thank professors Pamela Moulton, Carole Comerton-Forde and Robert Faff for examining this thesis and offering guidance on publication. All remaining errors are my own. I am grateful to my family for support and enabling me to undertake this project. I appreciate The University of Western Australia funding this research through a University Postgraduate Research Award and a University Top-Up Scholarship. I thank the Securities Industry Research Centre of Asia-Pacific (SIRCA) for providing access to the Thomson Reuters Tick History (TRTH) data. 9 1 Introduction and overview Some relationships in the stock market make for very profitable trades. – Stéphane Reverre in Pairs Trading (2001) The Law of One Price (LOOP) is the enticing proposition that identical and fungible items should trade at the same price.
Details
-
File Typepdf
-
Upload Time-
-
Content LanguagesEnglish
-
Upload UserAnonymous/Not logged-in
-
File Pages244 Page
-
File Size-