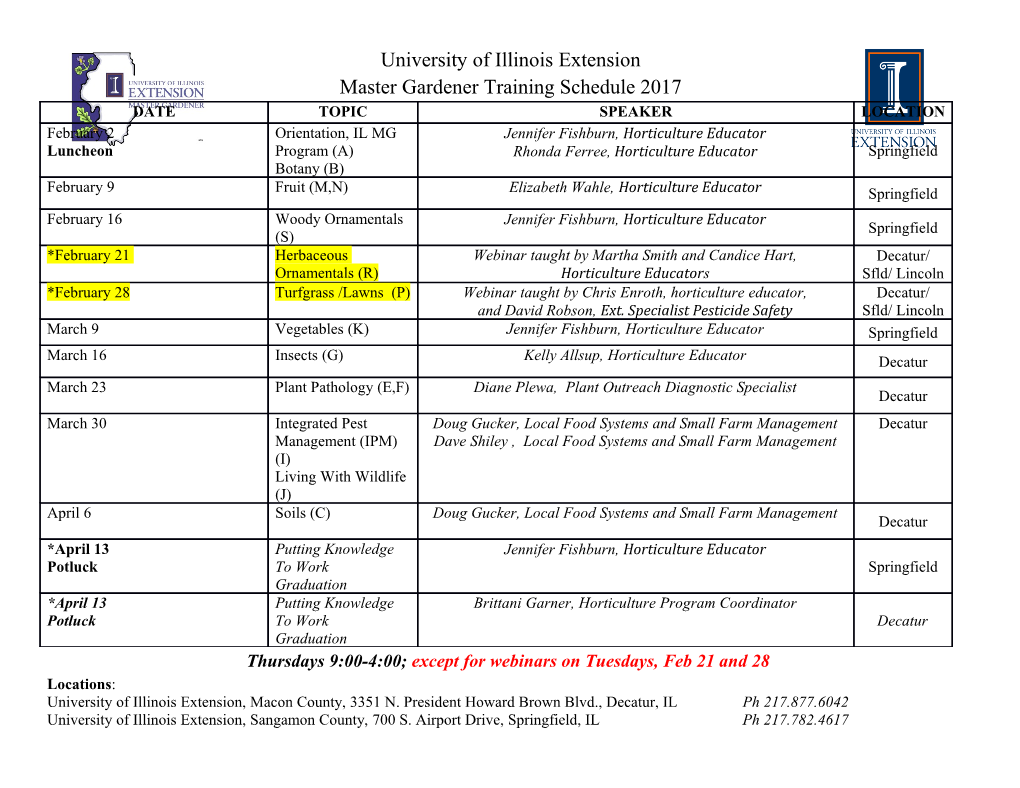
Statistics and Probability Letters 82 (2012) 455–463 Contents lists available at SciVerse ScienceDirect Statistics and Probability Letters journal homepage: www.elsevier.com/locate/stapro Asymptotics for dependent Bernoulli random variables Lan Wu a, Yongcheng Qi b, Jingping Yang a,∗ a LMAM, Department of Financial Mathematics, Center for Statistical Science, Peking University, Beijing 100871, China b Department of Mathematics and Statistics, University of Minnesota Duluth, 1117 University Drive, Duluth, MN 55812, USA article info a b s t r a c t Article history: This paper considers a sequence of Bernoulli random variables which are dependent in a Received 25 March 2011 way that the success probability of a trial conditional on the previous trials depends on Received in revised form 5 December 2011 the total number of successes achieved prior to the trial. The paper investigates almost Accepted 5 December 2011 sure behaviors for the sequence and proves the strong law of large numbers under weak Available online 14 December 2011 conditions. For linear probability functions, the paper also obtains the strong law of large numbers, the central limit theorems and the law of the iterated logarithm, extending the MSC: results by James et al.(2008). 60F15 ' 2011 Elsevier B.V. All rights reserved. Keywords: Dependent Bernoulli random variables Strong law of large numbers Central limit theorem Law of the iterated logarithm 1. Introduction Consider a sequence of Bernoulli random variables fXn; n ≥ 1g, which are dependent in a way that the success probability of a trial conditional on the previous trials depends on the total number of successes achieved to that point. In particular, we assume that P.XnC1 D 1jFn/ D θn C gn.Sn=n/; (1) D Pn ≥ D ≥ where Sn jD1 Xj for n 1, Fn σ .X1;:::; Xn/ is the σ -field generated by X1;:::; Xn, θn 0 is a sequence of constants, and gn.x/ is a non-negative measurable function defined over T0; 1U such that 0 ≤ θn C gn.x/ ≤ 1 for x 2 T0; 1U. When θn D p.1−γ / and gn.x/ D γ x, where p 2 .0; 1/ and γ 2 T0; 1/ are constants, model (1) reduces to the generalized binomial model which was first introduced by Drezner and Farnum(1993). If P.X1 D 1/ D p, then X1; X2;::: are identically distributed Bernoulli random variables, and in this case, Drezner and Farnum(1993) obtained the distribution of Sn. Heyde (2004) investigated the limiting distributions of Sn and proved that the central limit theorem for Sn holds when γ ≤ 1=2. Recently James et al.(2008) explored model (1) when θn D p.1 − dn/ and gn.x/ D dnx, where p 2 .0; 1/ and dn 2 T0; 1/ is a sequence of constants. They obtained the conditions for the strong law of large numbers, the central limit theorem and the law of the iterated logarithm for the partial sums of the dependent Bernoulli random variables. In this paper, we are mainly interested in investigating the strong law of large numbers for the partial sum Sn under the general setup (1). We also consider the central limit theorem, and the law of the iterated logarithm when the probability function on the right-hand side of (1) is linear. The main results of the paper are given in Section2, and all the proofs are put in Section3. ∗ Corresponding author. Fax: +86 10 62751801. E-mail address: [email protected] (J. Yang). 0167-7152/$ – see front matter ' 2011 Elsevier B.V. All rights reserved. doi:10.1016/j.spl.2011.12.002 456 L. Wu et al. / Statistics and Probability Letters 82 (2012) 455–463 2. Main results We first present a strong limit theorem from which we conclude the law of large numbers for Sn for general probability functions. Then we consider some linear probability functions and obtain a refined strong law of large numbers, the central limit theorem and the law of the iterated logarithm. We introduce a few examples where conditions for the strong law of large numbers in the general case can be verified. An example is also given to show that the upper limit and the lower limit are attainable in general. 1 Pn D 2 T U Theorem 1. Assume that limn!1 n jD1 θj θ 0; 1 and there exists a non-decreasing continuous function g defined on T0; 1U such that (C1) gn.t/ converges to g.t/ uniformly on T0; 1U, i.e. supx2T0;1U jgn.x/ − g.x/j ! 0 as n ! 1. Then S S ∗ ≤ n ≤ n ≤ ∗ ν1 lim inf lim sup ν2 a.s.; (2) n!1 n n!1 n where ∗ D f 2 T UV − ≥ g ∗ D f 2 T UV − ≤ g ν1 inf x 0; 1 x g.x/ θ ; ν2 sup x 0; 1 x g.x/ θ : (3) Remark 1. Consider a sequence of bounded random variables fXn; n ≥ 1g distributed over an interval Tb1; b2U such that P.XnC1jFn/ D θn C gn.Sn=n/; where fθng is a sequence of constants and fgn; n ≥ 1g is a sequence of functions defined over Tb1; b2U. Replacing all intervals T0; 1U by Tb1; b2U in Theorem 1, then (2) can be proved similarly under the above new model. Since the above model is not the focus in this paper, details for this development are not provided here. Remark 2. In some cases, the lower and upper limits in (2) are attainable. Let θn D 0 and gn.x/ D g.x/ for all n ≥ 1, where T U D D ∗ D g.x/ is a continuous and nondecreasing function defined on 0; 1 with g.0/ 0 and g.1/ 1. It is easy to see that ν1 0 ∗ D D D − D D 2 and ν2 1. Let P.X1 1/ 1 P.X1 0/ p .0; 1/. It is trivial to see that S D I n D 1; if X1 1 n 0; if X1 D 0: Hence, both the lower and upper limits in (2) are attainable with a positive probability. Evidently, E.Sn=n/ D p. Next we will give a less trivial example in which both the lower and upper limits in (2) are achieved with a positive probability. Example 1. Let 0 < v1 < v2 < 1 be two arbitrary constants and g any nondecreasing continuous function defined over T0; 1U such that v ; if x ≤ v I g.x/ D 1 1 v2; if x ≥ v2: Let fδng be a non-increasing sequence of constants satisfying that 2 nδn δ1 < min.v1; 1 − v2/; δn ! 0 and ! 1 as n ! 1: log log n Define (v1 − δn; if 0 ≤ x ≤ v1I gn.x/ D g.x/; if v1 < x < v2I v2 C δn; if v2 ≤ x ≤ 1: j − j ! D D ∗ D Then supx2T0;1U gn.x/ g.x/ 0. Now assume (1) holds with θn 0 for all n and 0 < P.X1 1/ < 1. Hence v1 v1 and ∗ D v2 v2 in (3). We prove in Section3 that S n D ∗ D P lim vi > 0 i 1; 2: (4) n!1 n Under some additional conditions, we conclude the strong law of large numbers for Sn as a consequence of Theorem 1. L. Wu et al. / Statistics and Probability Letters 82 (2012) 455–463 457 Corollary 1. In addition to the conditions in Theorem 1, if (C2) there exists a unique solution, say ν∗, to equation x − g.x/ D θ, x 2 T0; 1U, then Sn ∗ lim D v a.s.: (5) n!1 n Corollary 2. Assume g.x/ is a non-decreasing continuous function on T0; 1U satisfying the assumptions in Theorem 1. Then condition (C2) is fulfilled (and hence (5) holds) if any one of the following conditions holds: (C3) g.x/ is strictly convex such that g.0/ C θ > 0 and g.1/ C θ < 1; (C4) g.x/ is strictly concave such that g.0/ C θ > 0 and g.1/ C θ < 1; (C5) x − g.x/ is strictly increasing on T0; 1U. We present some examples in which the conditions in Corollary 2 can be easily verified. Example 2. Assume constants c > 0, β > 0 and θ 2 .0; 1/ such that θ C β < 1. Define g.x/ D βxc , x 2 T0; 1U. Then condition (C3) is satisfied if c > 1, condition (C4) is satisfied if c < 1, and condition (C5) is satisfied if c D 1. Example 3. Assume θ 2 .0; 1/, and g.x/ is a non-decreasing continuous function on T0; 1U. If g0.x/ < 1 for x 2 .0; 1/, then condition (C5) is satisfied. Consider some linear probability functions gn.x/ D dnx in (1), i.e., we assume that Xn, n ≥ 1 satisfy the model −1 P.XnC1 D 1jFn/ D θn C dnn Sn; (6) where 0 ≤ θn < 1, 0 ≤ dn < 1 and 0 ≤ θn C dn < 1 for every n ≥ 1. This is an extension of the model considered in James et al.(2008) where θn D p.1 − dn/ for some p 2 .0; 1/. From (6), the success probabilities pn VD P.Xn D 1/, n ≥ 1 can be recursively obtained by the formula n−1 dn−1 X pn D θn−1 C pj; n ≥ 2: (7) n − 1 jD1 Under model (6), we can obtain a necessary and sufficient condition for the strong law of large numbers for Sn. Theorem 2. Assume model (6) holds. Then Sn − E.Sn/ lim D 0 a.s.
Details
-
File Typepdf
-
Upload Time-
-
Content LanguagesEnglish
-
Upload UserAnonymous/Not logged-in
-
File Pages9 Page
-
File Size-